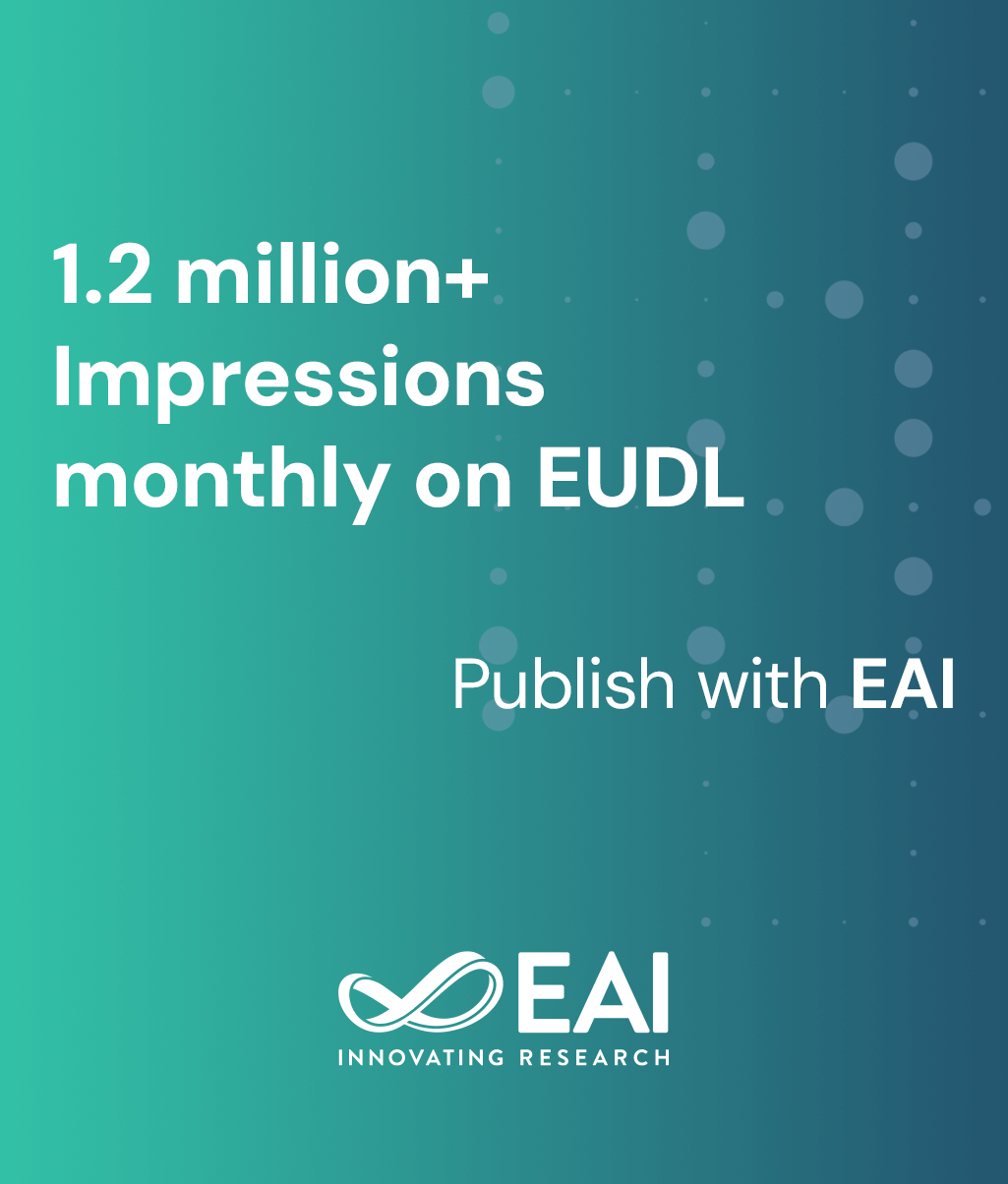
Research Article
Mining Emerging Sequential Patterns for Activity Recognition in Body Sensor Networks
@INPROCEEDINGS{10.1007/978-3-642-29154-8_9, author={Tao Gu and Liang Wang and Hanhua Chen and Guimei Liu and Xianping Tao and Jian Lu}, title={Mining Emerging Sequential Patterns for Activity Recognition in Body Sensor Networks}, proceedings={Mobile and Ubiquitous Systems: Computing, Networking, and Services. 7th International ICST Conference, MobiQuitous 2010, Sydeny, Australia, December 6-9, 2010, Revised Selected Papers}, proceedings_a={MOBIQUITOUS}, year={2012}, month={10}, keywords={Body sensor networks activity recognition data mining}, doi={10.1007/978-3-642-29154-8_9} }
- Tao Gu
Liang Wang
Hanhua Chen
Guimei Liu
Xianping Tao
Jian Lu
Year: 2012
Mining Emerging Sequential Patterns for Activity Recognition in Body Sensor Networks
MOBIQUITOUS
Springer
DOI: 10.1007/978-3-642-29154-8_9
Abstract
Body Sensor Networks offer many applications in healthcare, well-being and entertainment. One of the emerging applications is recognizing activities of daily living. In this paper, we introduce a novel knowledge pattern named Emerging Sequential Pattern (ESP)—a sequential pattern that discovers significant class differences—to recognize both simple (i.e., sequential) and complex (i.e., interleaved and concurrent) activities. Based on ESPs, we build our complex activity models directly upon the sequential model to recognize both activity types. We conduct comprehensive empirical studies to evaluate and compare our solution with the state-of-the-art solutions. The results demonstrate that our approach achieves an overall accuracy of 91.89%, outperforming the existing solutions.