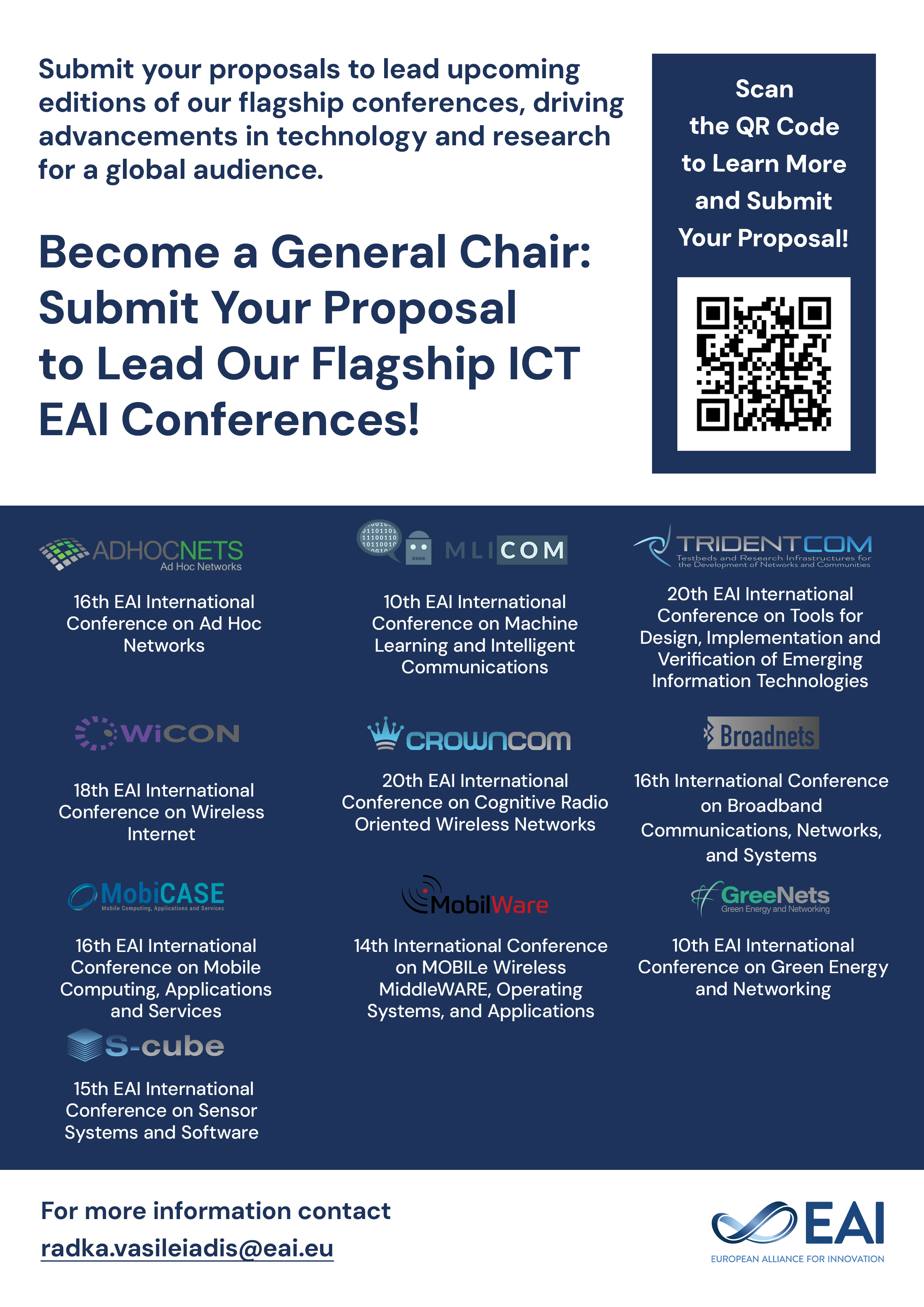
Research Article
An Innovative Approach to Association Rule Mining Using Graph and Clusters
@INPROCEEDINGS{10.1007/978-3-642-27317-9_7, author={N. Raja and G. Balakrishnan}, title={An Innovative Approach to Association Rule Mining Using Graph and Clusters}, proceedings={Advances in Computer Science and Information Technology. Computer Science and Information Technology. Second International Conference, CCSIT 2012, Bangalore, India, January 2-4, 2012. Proceedings, Part III}, proceedings_a={CCSIT PART III}, year={2012}, month={11}, keywords={Association rule mining Data Mining Relational cluster graph GCBARM}, doi={10.1007/978-3-642-27317-9_7} }
- N. Raja
G. Balakrishnan
Year: 2012
An Innovative Approach to Association Rule Mining Using Graph and Clusters
CCSIT PART III
Springer
DOI: 10.1007/978-3-642-27317-9_7
Abstract
There is a common belief that the association rule mining has a major stake in data mining research domain. Numerous algorithms are proposed for discovering frequent itemsets. This is the key process in association rule mining. This proposed paper introduces two concepts. First leads to analyze the ability to explore the demographic parameters of the underlying entities and their inter relations using the traditional graph theory approach(vertices and edges). Second is the improved algorithm based on graph and clustering based mining association rules. This improved algorithm is named Graph and Clustering Based Association Rule Mining (GCBARM). The GCBARM algorithm scans the database of transaction only once to generate a cluster table and then clusters the transactions into cluster according to their length. The GCBARM algorithm is used to find frequent itemsets and will be extracted directly by scanning the cluster table. This method reduces memory requirement and time to retrieve the datasets and hence it is scalable for any large size of the database.