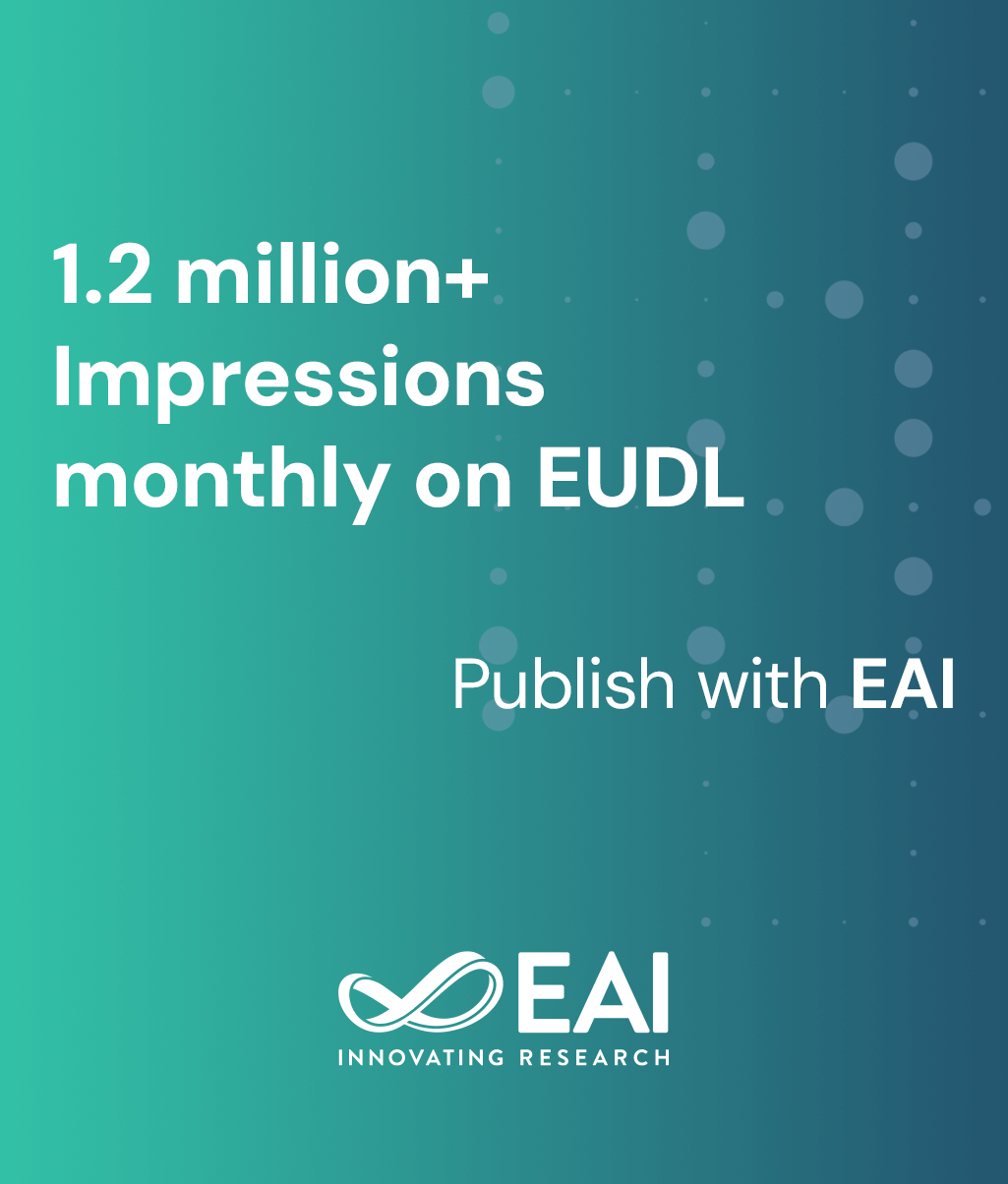
Research Article
Single Reduct Generation by Attribute Similarity Measurement Based on Relative Indiscernibility
@INPROCEEDINGS{10.1007/978-3-642-27308-7_51, author={Shampa Sengupta and Asit Das}, title={Single Reduct Generation by Attribute Similarity Measurement Based on Relative Indiscernibility}, proceedings={Advances in Computer Science and Information Technology. Computer Science and Engineering. Second International Conference, CCSIT 2012, Bangalore, India, January 2-4, 2012. Proceedings, Part II}, proceedings_a={CCSIT PATR II}, year={2012}, month={11}, keywords={Rough Set Theory Attribute Similarity Relative Indiscernibility Relation Reduct}, doi={10.1007/978-3-642-27308-7_51} }
- Shampa Sengupta
Asit Das
Year: 2012
Single Reduct Generation by Attribute Similarity Measurement Based on Relative Indiscernibility
CCSIT PATR II
Springer
DOI: 10.1007/978-3-642-27308-7_51
Abstract
In real world everything is an object which represents particular classes. Every object can be fully described by its attributes. Any real world dataset contains large number of attributes and objects. Classifiers give poor performance when these huge datasets are given as input to it for proper classification. So from these huge dataset most useful attributes need to be extracted that contribute the maximum to the decision. In the paper, attribute set is reduced by generating reducts using the indiscernibility relation of Rough Set Theory (RST). The method measures similarity among the attributes using relative indiscernibility relation and computes attribute similarity set. Then the set is minimized and an attribute similarity table is constructed from which attribute similar to maximum number of attributes is selected so that the resultant minimum set of selected attributes (called reduct) cover all attributes of the attribute similarity table. The method has been applied on glass dataset collected from the UCI repository and the classification accuracy is calculated by various classifiers. The result shows the efficiency of the proposed method.