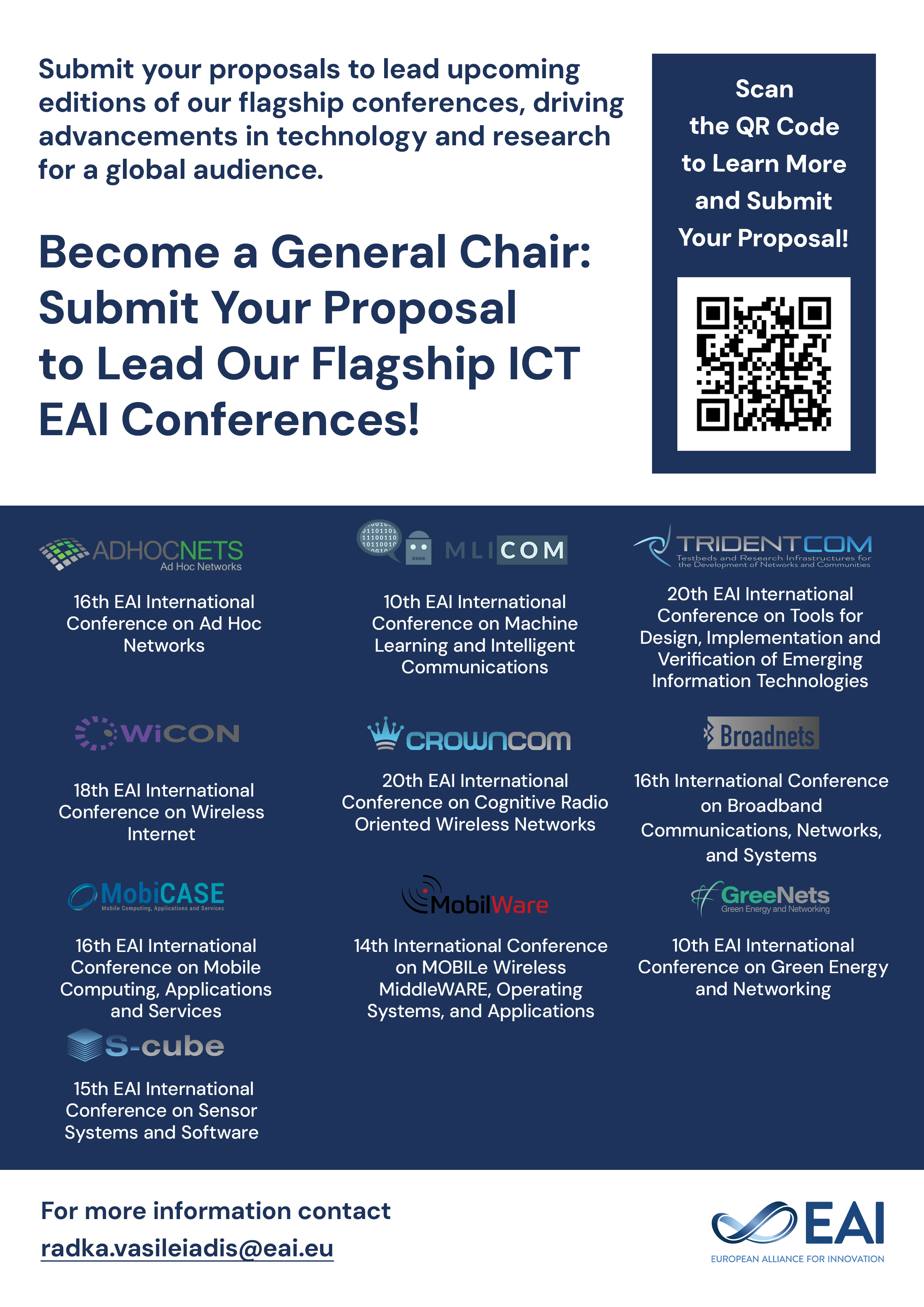
Research Article
Classification of Moving Vehicles in Traffic Videos
@INPROCEEDINGS{10.1007/978-3-642-27308-7_22, author={Elham Dallalzadeh and D. Guru and S. Manjunath and M. Suraj}, title={Classification of Moving Vehicles in Traffic Videos}, proceedings={Advances in Computer Science and Information Technology. Computer Science and Engineering. Second International Conference, CCSIT 2012, Bangalore, India, January 2-4, 2012. Proceedings, Part II}, proceedings_a={CCSIT PATR II}, year={2012}, month={11}, keywords={Corner-based tracking shape reconstruction shape normalization shape feature extraction vehicle classification k-nearest neighbor weighted k-nearest neighbor}, doi={10.1007/978-3-642-27308-7_22} }
- Elham Dallalzadeh
D. Guru
S. Manjunath
M. Suraj
Year: 2012
Classification of Moving Vehicles in Traffic Videos
CCSIT PATR II
Springer
DOI: 10.1007/978-3-642-27308-7_22
Abstract
In this paper, we propose a model for classification of moving vehicles in traffic videos. We present a corner-based tracking method to track and detect moving vehicles. The detected vehicles are classified into 4 different types of vehicle classes using optimal classifiers. The proposed classification method is based on overlapping the boundary curves of each vehicle while tracking it in sequence of frames to reconstruct a complete boundary shape of it. The reconstructed boundary shape is normalized and a set of efficient shape features are extracted. Vehicles are classified by k-NN rule and the proposed weighted k-NN classifier. Experiments are conducted on 23.02 minutes of moderate traffic videos of roadway scenes taken in an uncontrolled environment during day time. The proposed method has 94.32% classification accuracy which demonstrates the effectiveness of our method. The proposed method has 87.45% of precision with 79% recall rate for classification of moving vehicles.