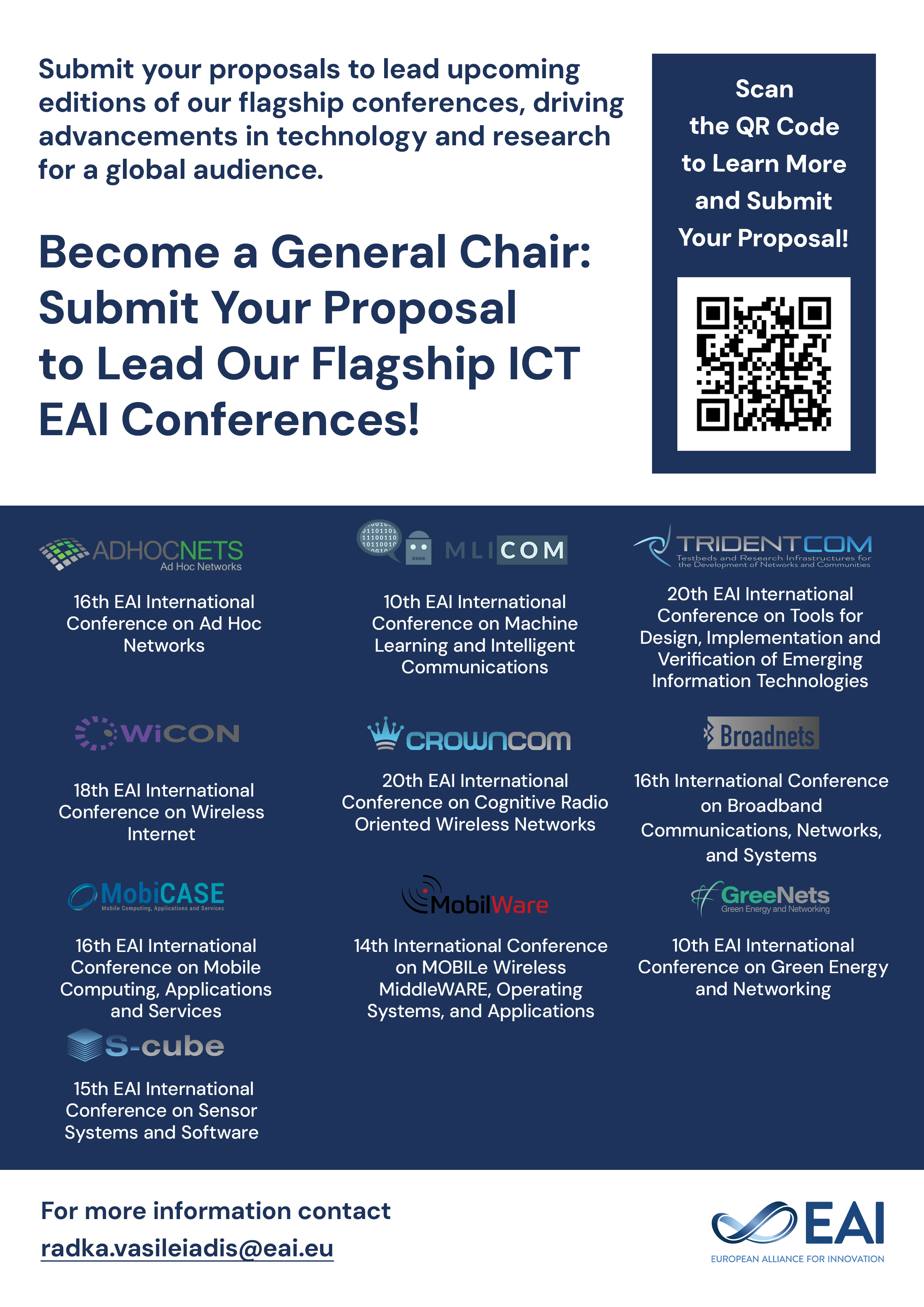
Research Article
Adaptive MMSE Equalizer through LMS Algorithm Based CMA Channel Equalization
@INPROCEEDINGS{10.1007/978-3-642-27299-8_33, author={R. Nirmala Devi and Tara Saikumar and K. Kishan Rao}, title={Adaptive MMSE Equalizer through LMS Algorithm Based CMA Channel Equalization}, proceedings={Advances in Computer Science and Information Technology. Networks and Communications. Second International Conference, CCSIT 2012, Bangalore, India, January 2-4, 2012. Proceedings, Part I}, proceedings_a={CCSIT PART I}, year={2012}, month={11}, keywords={CMA LMS Algorithm MMSE}, doi={10.1007/978-3-642-27299-8_33} }
- R. Nirmala Devi
Tara Saikumar
K. Kishan Rao
Year: 2012
Adaptive MMSE Equalizer through LMS Algorithm Based CMA Channel Equalization
CCSIT PART I
Springer
DOI: 10.1007/978-3-642-27299-8_33
Abstract
The adaptive algorithm has been widely used in the digital signal processing like channel estimation, channel equalization, echo cancellation, and so on. One of the most important adaptive algorithms is the LMS algorithm. We present in this paper an multiple objective optimization approach to fast blind channel equalization. By investigating first the performance (mean-square error) of the standard fractionally spaced CMA (constant modulus algorithm) equalizer in the presence of noise, we show that CMA local minima exist near the minimum mean-square error (MMSE) equalizers. Consequently, CMA may converge to a local minimum corresponding to a poorly designed MMSE receiver with considerablely large mean-square error. The step size in the LMS algorithm decides both the convergence speed and the residual error level, the highest speed of convergence and residual error level.