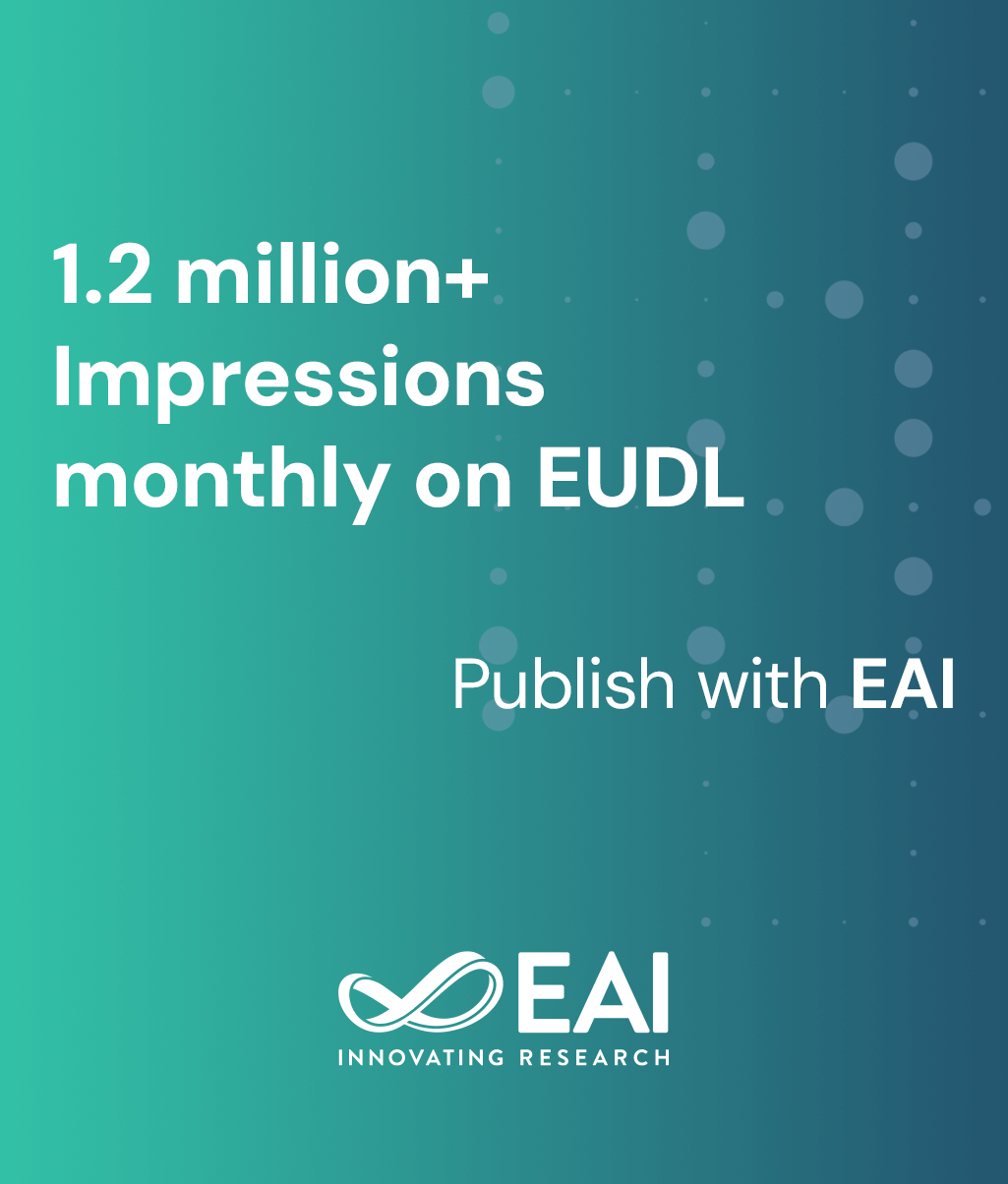
Research Article
A Recommender System for Sweaty Sock Syndrome
@INPROCEEDINGS{10.1007/978-3-642-27299-8_10, author={L. Arockiam and S. Charles and C. Lalitha and I. Carol}, title={A Recommender System for Sweaty Sock Syndrome}, proceedings={Advances in Computer Science and Information Technology. Networks and Communications. Second International Conference, CCSIT 2012, Bangalore, India, January 2-4, 2012. Proceedings, Part I}, proceedings_a={CCSIT PART I}, year={2012}, month={11}, keywords={Multilayer Perceptron (MLP) EM Clustering Sweaty Sock Syndrome (SSS) Dermatosis}, doi={10.1007/978-3-642-27299-8_10} }
- L. Arockiam
S. Charles
C. Lalitha
I. Carol
Year: 2012
A Recommender System for Sweaty Sock Syndrome
CCSIT PART I
Springer
DOI: 10.1007/978-3-642-27299-8_10
Abstract
Dermatosis disease is also known as Sweaty Sock Syndrome (SSS). Most of the children and young teenagers are affected by SSS. It damages the skin of the children and the young teenagers with red soles on the feet. A new methodology is used to find the stages of Sweaty Sock Syndrome using Multilayer perceptron (MLP) and EM clustering technique. The symptoms and stages of SSS are classified by using predictive modeling. In Multilayer perceptron technique, data objects are classified based on the stages of SSS and find out their efficiency and accuracy. EM Clustering is an unsupervised technique, which is characterized the objects based on the weights. Supervised learning identifies the various symptoms of SSS disease. It categorizes the data such as initial, non severe and severe by using learning by example. Learning by observation method categorizes the data into different clusters, which is grouped as initial, non severe and severe. It helps to know the various stages of dermatosis by using predictive and descriptive modeling. This prediction helps to recommend the patients those who are affected by SSS and provide suggestion to the patients.