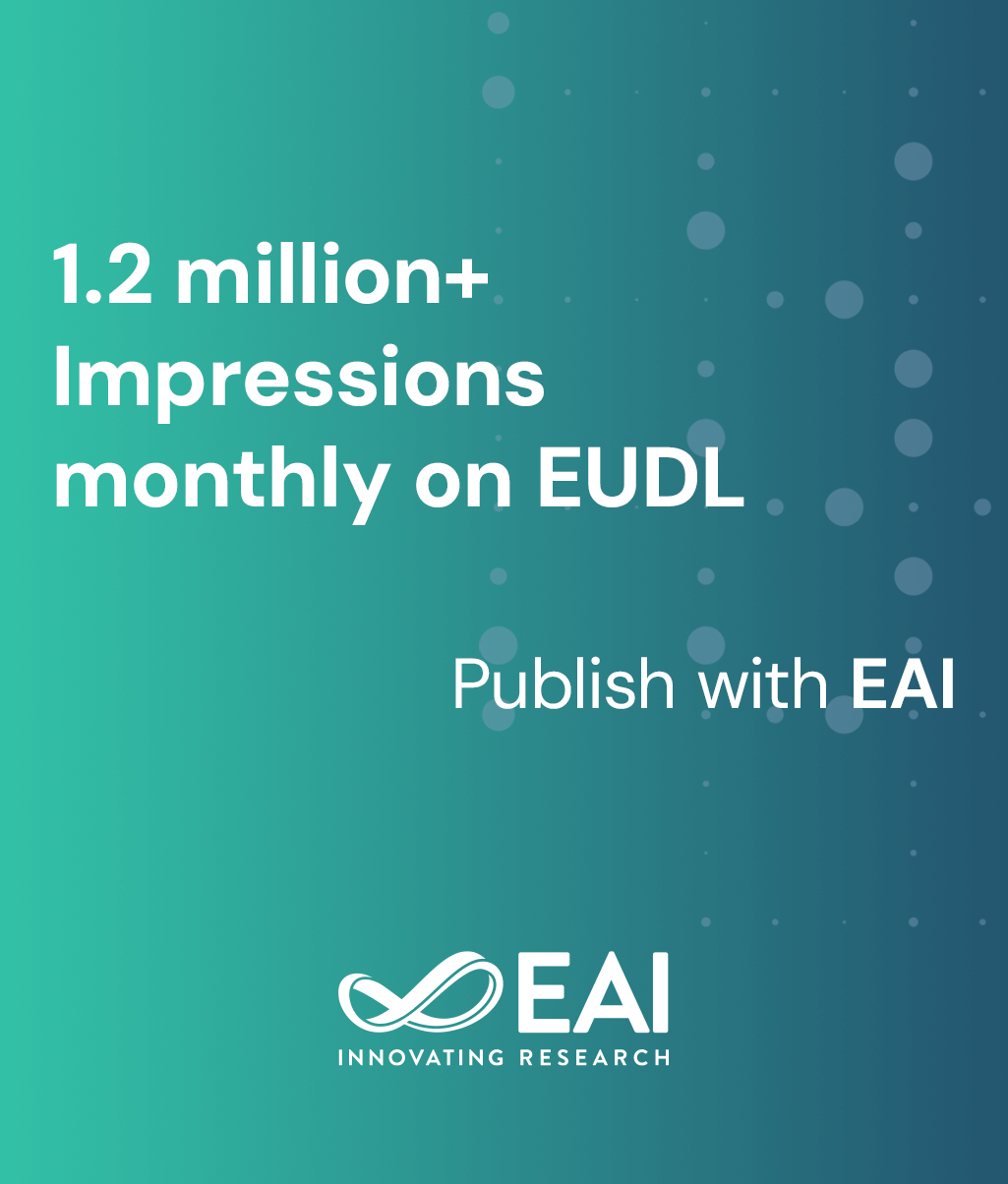
Research Article
Detecting Network Intrusions Using Hierarchical Temporal Memory
@INPROCEEDINGS{10.1007/978-3-642-23828-4_5, author={Gift Khangamwa}, title={Detecting Network Intrusions Using Hierarchical Temporal Memory}, proceedings={E-Infrastuctures and E-Services for Developing Countries. Second International ICST Conference, AFRICOM 2010, Cape Town, South Africa, November 25-26, 2010, Revised Selected Papers}, proceedings_a={AFRICOMM}, year={2012}, month={5}, keywords={Intrusion detection Artificial Intelligence Hierarchical Temporal Memory Network anomaly detection}, doi={10.1007/978-3-642-23828-4_5} }
- Gift Khangamwa
Year: 2012
Detecting Network Intrusions Using Hierarchical Temporal Memory
AFRICOMM
Springer
DOI: 10.1007/978-3-642-23828-4_5
Abstract
Intrusion Detection Systems (IDS) are a very popular network security tool. These tools can allow network administrators, to identify and react to hostile traffic aimed at, or generated from their own network. In general there are two common Intrusion Detection approaches which are behavior or traffic anomaly based and knowledge or signature based. As a result of the increased sophistication of intrusion attacks, one very desirable feature of advanced IDS is to be capable of learning and generalizing from known traffic patterns of a system, process or a user’s behavior. In this project we investigated the use of a novel Artificial Intelligence (AI) approach to intrusion detection based on network traffic anomaly detection. The AI technique used is based on the Hierarchical Temporal Memory (HTM) paradigm developed by Numenta, which is a relatively new AI concept that mimics the operation of the neocortex area of the human brain[11,14]. The developed AI scheme was evaluated using the corpus of data from Massachusetts Institute of Technology, Lincoln Laboratories in USA [20]. Our results show that HTM based intrusion detection can achieve relatively high success rates in identifying anomalous traffic in computer networks, furthermore our research also shows that HTM based schemes can achieve very fast detection rates making them a very good alternative for real time intrusion detection engine.