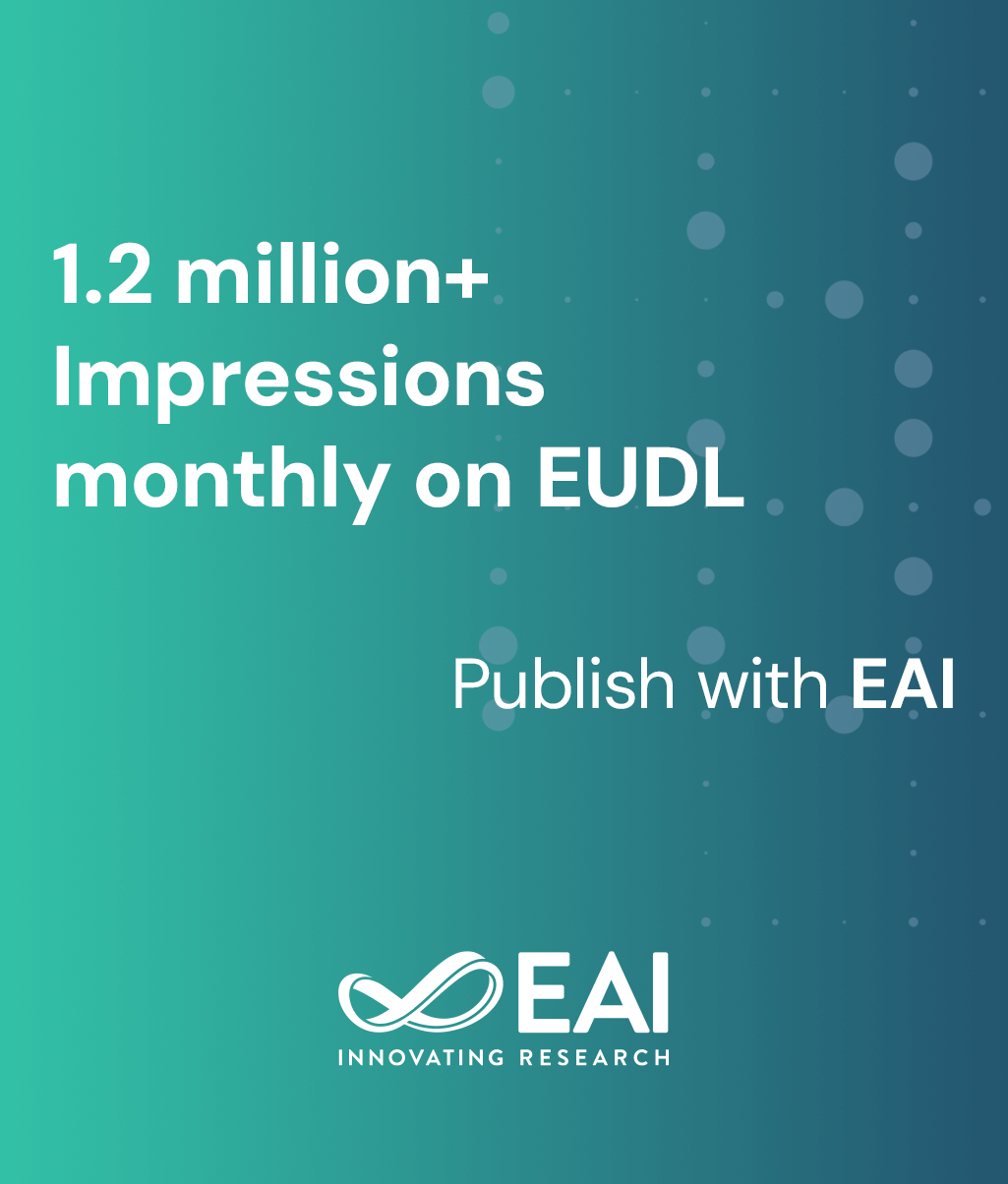
Research Article
#Swineflu: Twitter Predicts Swine Flu Outbreak in 2009
@INPROCEEDINGS{10.1007/978-3-642-23635-8_3, author={Martin Szomszor and Patty Kostkova and Ed Quincey}, title={\#Swineflu: Twitter Predicts Swine Flu Outbreak in 2009}, proceedings={Electronic Healthcare. Third International Conference, eHealth 2010, Casablanca, Morocco, December 13-15, 2010, Revised Selected Papers}, proceedings_a={E-HEALTH}, year={2012}, month={10}, keywords={Epidemic Intelligence Twitter H1N1 Pandemic Flu}, doi={10.1007/978-3-642-23635-8_3} }
- Martin Szomszor
Patty Kostkova
Ed Quincey
Year: 2012
#Swineflu: Twitter Predicts Swine Flu Outbreak in 2009
E-HEALTH
Springer
DOI: 10.1007/978-3-642-23635-8_3
Abstract
Early warning systems for the identification and tracking of infections disease outbreaks have become an important tool in the field of epidemiology. While government lead initiatives to increase the sharing of surveillance data have improved early detection and control, along with advanced web monitoring and analytics services, the recent swine flu outbreak of 2009 demonstrated the important role social media has and the wealth of data it exposes. In this paper, we present an investigation into Twitter, using around 3 Million tweets gathered between May and December 2009, as a possible source of surveillance data and its feasibility to serve as an early warning system. By performing simple filtering and normalization, we demonstrate that Twitter can serve as a self-reporting tool, and hence, provide indications of increased infection spreading. Our initial findings indicate that Twitter can detect such events up to one week before conventional GP reported surveillance data.