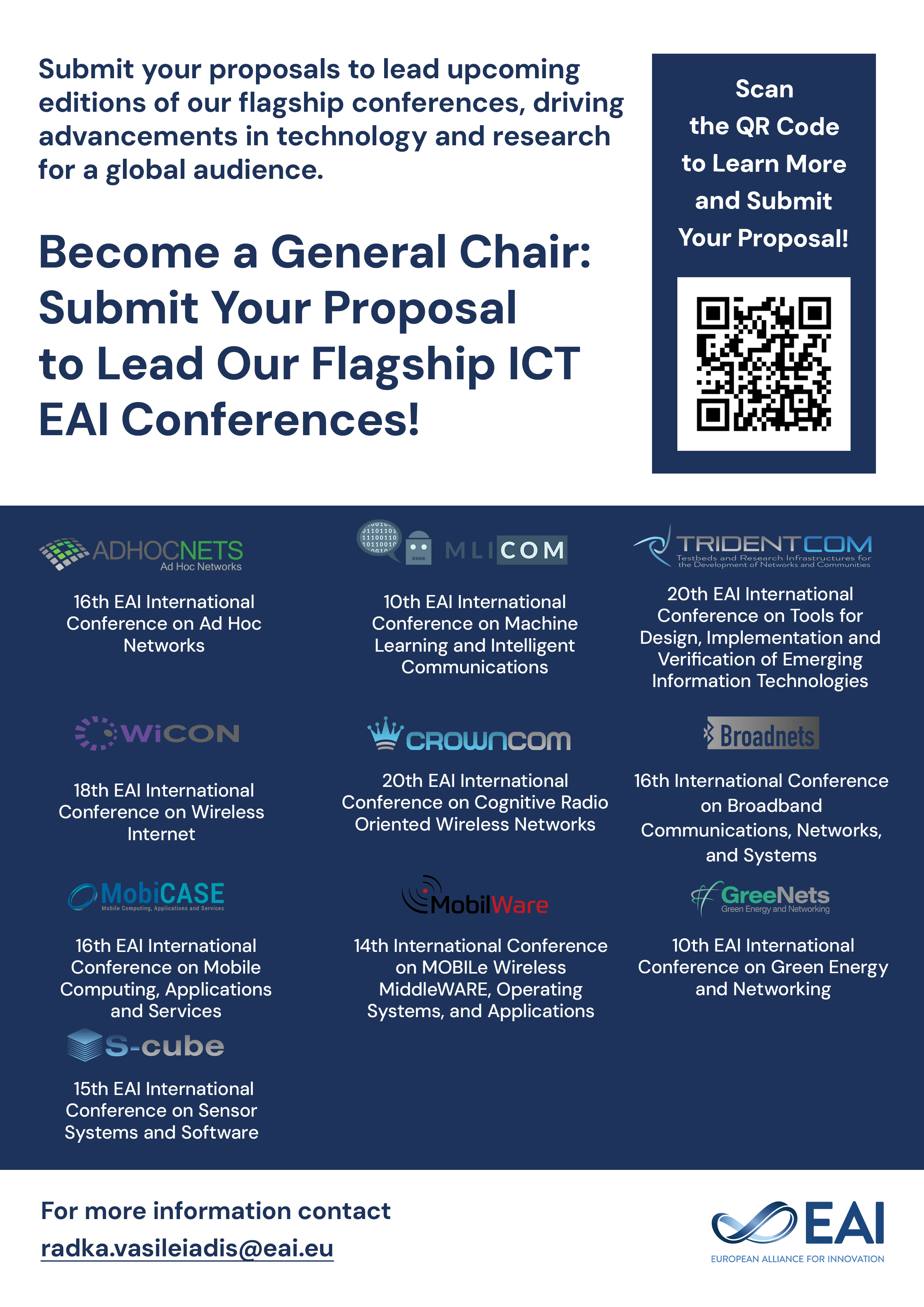
Research Article
Self-organization and Local Learning Methods for Improving the Applicability and Efficiency of Data-Centric Sensor Networks
@INPROCEEDINGS{10.1007/978-3-642-10625-5_40, author={Gabriele Monti and Gianluca Moro}, title={Self-organization and Local Learning Methods for Improving the Applicability and Efficiency of Data-Centric Sensor Networks}, proceedings={Quality of Service in Heterogeneous Networks. 6th International ICST Conference on Heterogeneous Networking for Quality, Reliability, Security and Robustness, QShine 2009 and 3rd International Workshop on Advanced Architectures and Algorithms for Internet Delivery and Applications, AAA-IDEA 2009, Las Palmas, Gran Canaria, November 23-25, 2009 Proceedings}, proceedings_a={QSHINE}, year={2012}, month={10}, keywords={}, doi={10.1007/978-3-642-10625-5_40} }
- Gabriele Monti
Gianluca Moro
Year: 2012
Self-organization and Local Learning Methods for Improving the Applicability and Efficiency of Data-Centric Sensor Networks
QSHINE
Springer
DOI: 10.1007/978-3-642-10625-5_40
Abstract
In data-centric sensor networks each device is like a minimal computer with cpu and memory able to sense, manage and transmit data performing in-network processing by means of insertions, querying and multi-hop routings. Saving energy is one of the most important goals, therefore radio transmissions, which are the most expensive operations, should be limited by optimizing the number of routings. Moreover the network traffic should be balanced among nodes in order to avoid premature discharge of some devices and then network partitions. In this paper we present a fully decentralized infrastructure able to self-organize fully functional data centric sensor networks from local interactions and learning among devices. Differently from existing solutions, our proposal does not require complex devices that need global information or external help from systems, such as the Global Positioning System (GPS), which works only outdoor with a precision and an efficacy both limited by weather conditions and obstacles. Our solution can be applied to a wider number of scenarios, including mesh networks and wireless community networks. The local learning occurs by exploiting implicit cost-free overhearing at sensors. The work reports an extensive number of comparative experiments, using several distributions of sensors and data, with a well-know competitor solution in literature, showing that an approach fully based on self-organization is more efficient than traditional solutions depending on GPS.