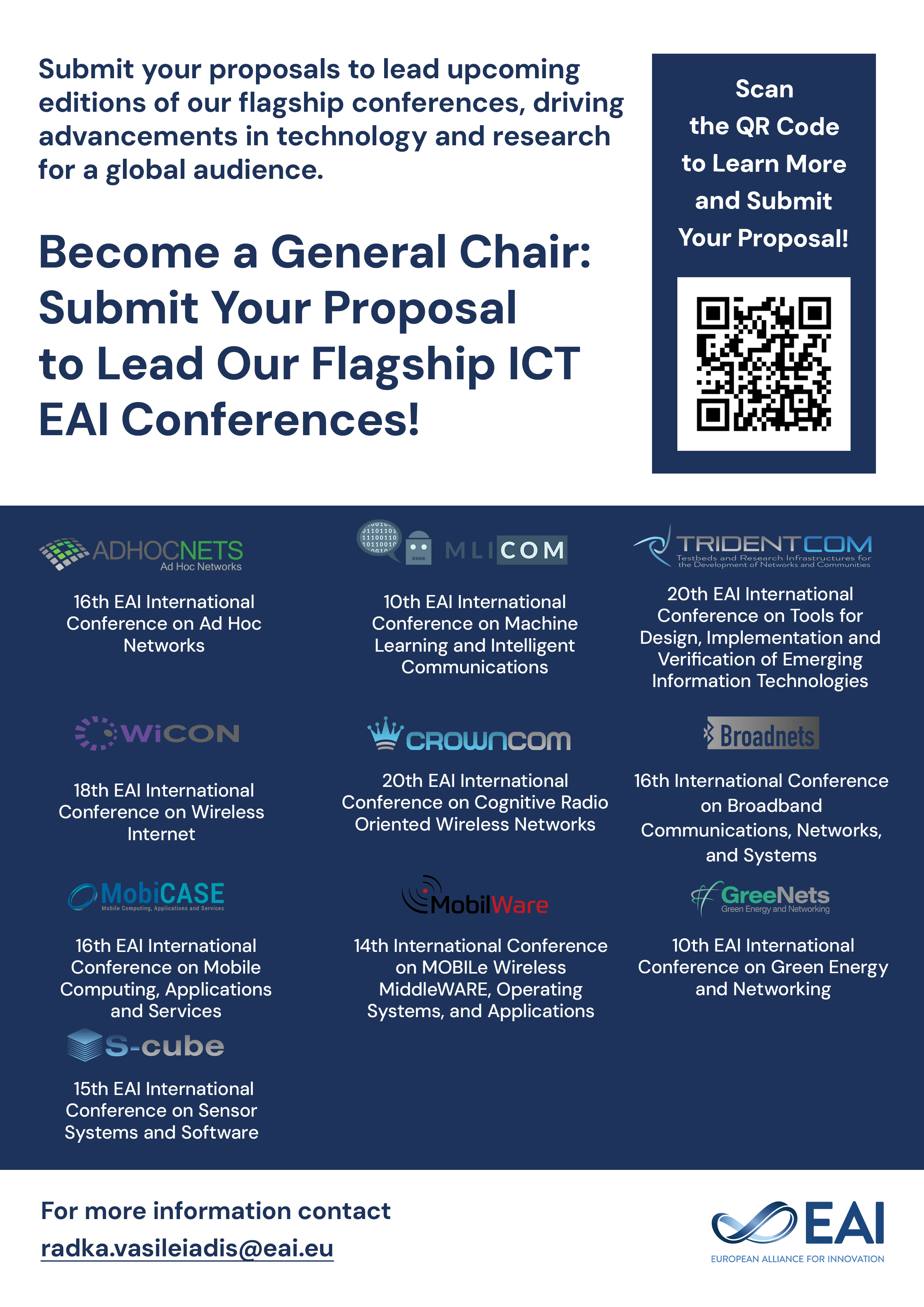
Research Article
Strong Dependence of Infection Profiles on Grouping Dynamics during Epidemiological Spreading
@INPROCEEDINGS{10.1007/978-3-642-02466-5_96, author={Zhenyuan Zhao and Guannan Zhao and Chen Xu and Pak Hui and Neil Johnson}, title={Strong Dependence of Infection Profiles on Grouping Dynamics during Epidemiological Spreading}, proceedings={Complex Sciences. First International Conference, Complex 2009, Shanghai, China, February 23-25, 2009. Revised Papers, Part 1}, proceedings_a={COMPLEX PART 1}, year={2012}, month={5}, keywords={complex systems networks epidemics group dynamics}, doi={10.1007/978-3-642-02466-5_96} }
- Zhenyuan Zhao
Guannan Zhao
Chen Xu
Pak Hui
Neil Johnson
Year: 2012
Strong Dependence of Infection Profiles on Grouping Dynamics during Epidemiological Spreading
COMPLEX PART 1
Springer
DOI: 10.1007/978-3-642-02466-5_96
Abstract
The spreading of an epidemic depends on the connectivity of the underlying host population. Because of the inherent difficulties in addressing such a problem, research to date on epidemics in networks has focused either on static networks, or networks with relatively few rewirings per timestep. Here we employ a simple, yet highly non-trivial, model of dynamical grouping to investigate the extent to which the underlying dynamics of tightly-knit communities can affect the resulting infection profile. Individual realizations of the spreading tend to be dominated by large peaks corresponding to infection resurgence, and a generally slow decay of the outbreak. In addition to our simulation results, we provide an analytical analysis of the run-averaged behaviour in the regime of fast grouping dynamics. We show that the true run-averaged infection profile can be closely mimicked by employing a suitably weighted static network, thereby dramatically simplifying the level of difficulty.