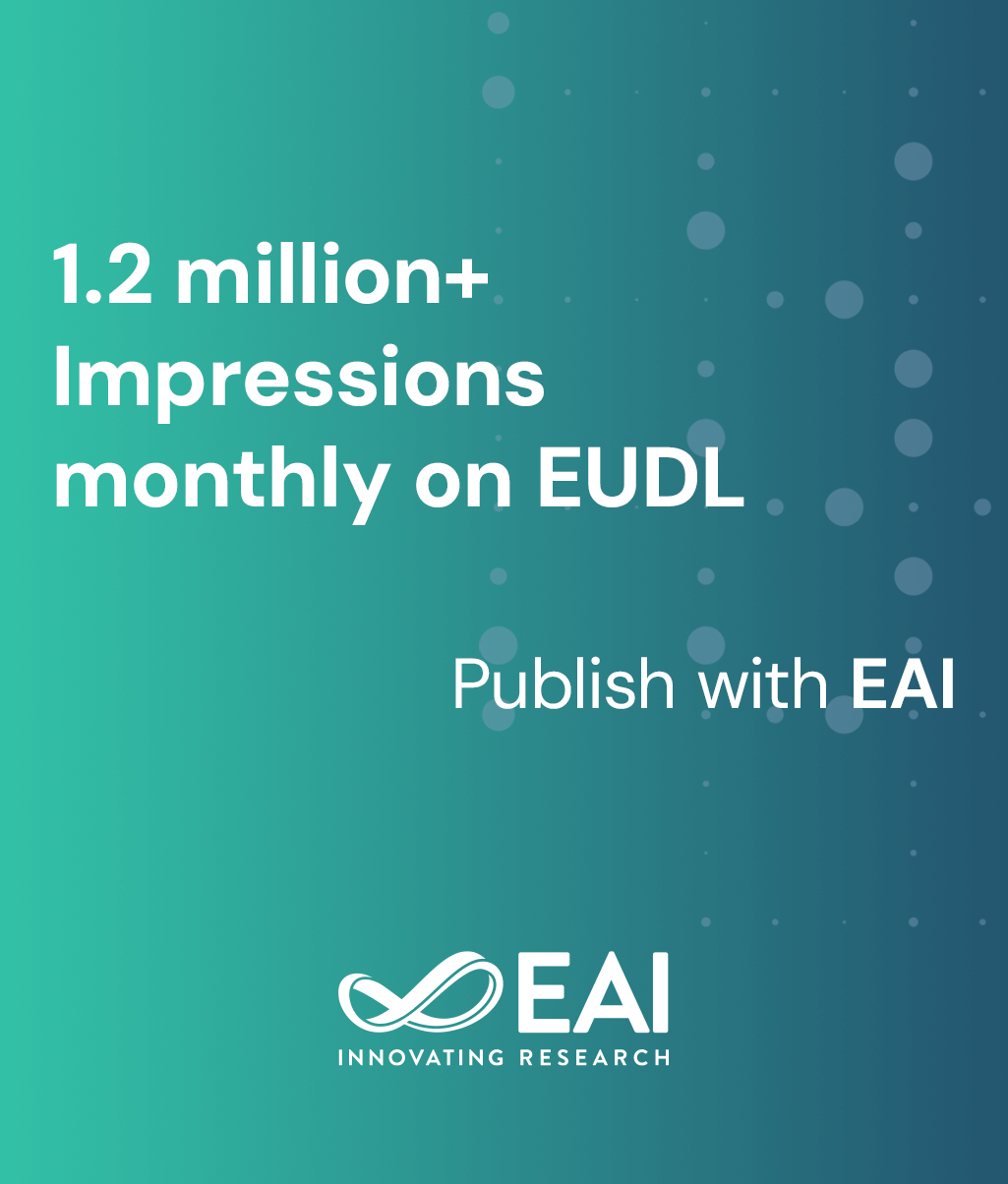
Research Article
On Scale-Free Prior Distributions and Their Applicability in Large-Scale Network Inference with Gaussian Graphical Models
@INPROCEEDINGS{10.1007/978-3-642-02466-5_9, author={Paul Sheridan and Takeshi Kamimura and Hidetoshi Shimodaira}, title={On Scale-Free Prior Distributions and Their Applicability in Large-Scale Network Inference with Gaussian Graphical Models}, proceedings={Complex Sciences. First International Conference, Complex 2009, Shanghai, China, February 23-25, 2009. Revised Papers, Part 1}, proceedings_a={COMPLEX PART 1}, year={2012}, month={5}, keywords={Bayesian inference complex networks Gaussian graphical model Markov chain Monte Carlo prior distribution scale-free “small large ” problem small-sample inference}, doi={10.1007/978-3-642-02466-5_9} }
- Paul Sheridan
Takeshi Kamimura
Hidetoshi Shimodaira
Year: 2012
On Scale-Free Prior Distributions and Their Applicability in Large-Scale Network Inference with Gaussian Graphical Models
COMPLEX PART 1
Springer
DOI: 10.1007/978-3-642-02466-5_9
Abstract
This paper concerns the specification, and performance, of scale-free prior distributions with a view toward large-scale network inference from small-sample data sets. We devise three scale-free priors and implement them in the framework of Gaussian graphical models. Gaussian graphical models are used in gene network inference where high-throughput data describing a large number of variables with comparatively few samples are frequently analyzed by practitioners. And, although there is a consensus that many such networks are scale-free, the is to assign a random network prior. Simulations demonstrate that the scale-free priors outperform the random network prior at recovering scale-free trees with degree exponents near 2, such as are characteristic of many real-world systems. On the other hand, the random network prior compares favorably at recovering scale-free trees characterized by larger degree exponents.