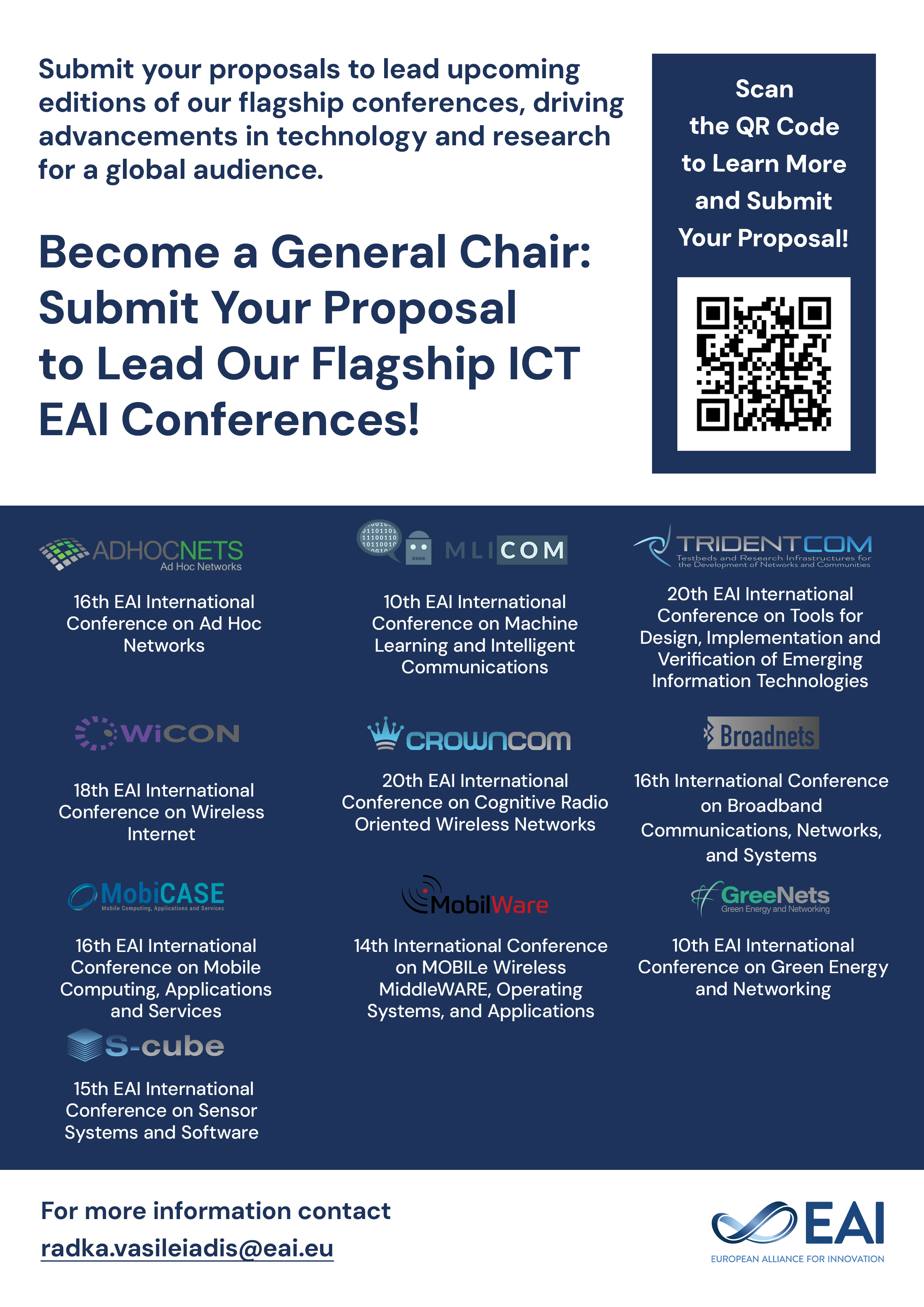
Research Article
Tracking the Evolution in Social Network: Methods and Results
@INPROCEEDINGS{10.1007/978-3-642-02466-5_67, author={Shengqi Yang and Bin Wu and Bai Wang}, title={Tracking the Evolution in Social Network: Methods and Results}, proceedings={Complex Sciences. First International Conference, Complex 2009, Shanghai, China, February 23-25, 2009. Revised Papers, Part 1}, proceedings_a={COMPLEX PART 1}, year={2012}, month={5}, keywords={Social Network Dynamic patterns Community Detection}, doi={10.1007/978-3-642-02466-5_67} }
- Shengqi Yang
Bin Wu
Bai Wang
Year: 2012
Tracking the Evolution in Social Network: Methods and Results
COMPLEX PART 1
Springer
DOI: 10.1007/978-3-642-02466-5_67
Abstract
Contrary to previous static knowledge, our dynamic view in social network is so limited. Recent uncovering those hidden dynamic patterns has posed a series of challenging problems in network evolution. To make effective exploration, we present a fundamentally novel framework for uncovering the intricate properties of evolutionary networks. Different from static snapshots methods, we firstly trace the timelines of networks, which could explicitly characterize the network to several evolving segments. Then based on extracted smooth segments from the timeline, a graph approximation algorithm is devised to capture the frequent characteristics of the network and reduce the noise of interactions. Moreover, by employing the relationship between multi-attributes, an innovative community detection algorithm is proposed for detailed analysis on the approximate graphs. Besides the algorithms, to track these dynamic communities, we also introduce a community correlation and evaluation criterion. Finally, applying this framework to several synthetic and real-world datasets, we demonstrate the critical relationship between event and social evolution, and find that close-knit relationship with well-distributed tie strengths among members of large communities will contribute to a longer life span.