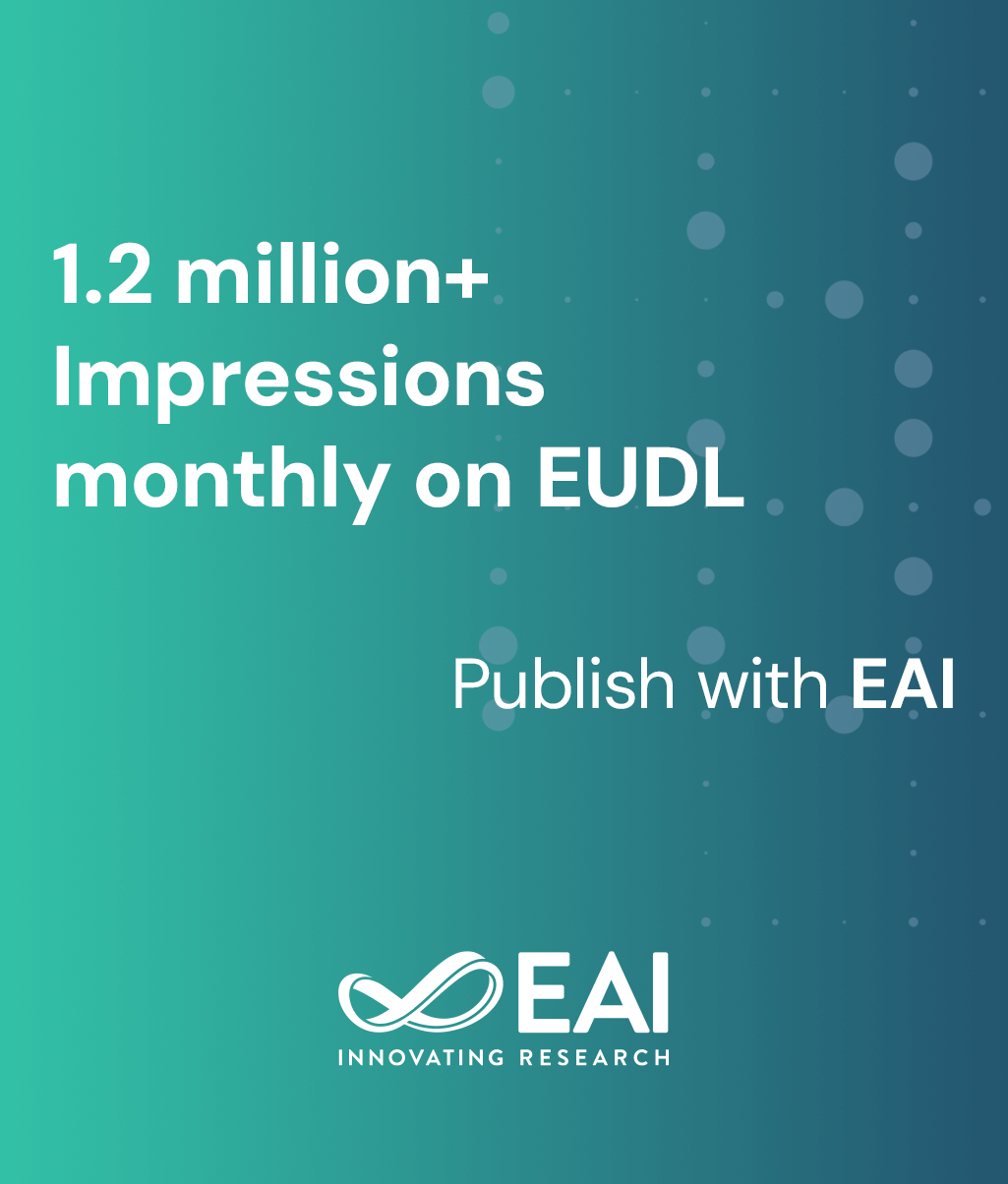
Research Article
Comparative Analysis of Supervised Learning for Sentiment Classification
@INPROCEEDINGS{10.1007/978-3-319-98827-6_26, author={Afusat Muyili and Oladipupo Sennaike}, title={Comparative Analysis of Supervised Learning for Sentiment Classification}, proceedings={e-Infrastructure and e-Services for Developing Countries. 9th International Conference, AFRICOMM 2017, Lagos, Nigeria, December 11-12, 2017, Proceedings}, proceedings_a={AFRICOMM}, year={2018}, month={8}, keywords={Sentiment analysis Twitter Feature extraction Na\~{n}ve Bayes Decision Tree Linear Support Vector Machine Multinomial Na\~{n}ve Bayes Bernoulli Na\~{n}ve Bayes}, doi={10.1007/978-3-319-98827-6_26} }
- Afusat Muyili
Oladipupo Sennaike
Year: 2018
Comparative Analysis of Supervised Learning for Sentiment Classification
AFRICOMM
Springer
DOI: 10.1007/978-3-319-98827-6_26
Abstract
Sentiment analysis is an active research area which deals with information extraction and knowledge discovery from text using Natural Language Processing and Data Mining techniques. Sentiment analysis, also known as opinion mining, plays a major role in detection of customer’s attitude, response and opinion towards a product or service. The aim of this paper is to perform sentiment analysis on a particular service to discover how users perceive the service automatically. Data is extracted from twitter, pre-processed and classified according to the sentiment expressed in them: positive, negative or neutral using five supervised learning classifiers-The Naïve Bayes, Multinomial Naïve Bayes (MNB), Bernoulli Naïve Bayes (BNB), Linear Support Vector Machine (SVM) and Decision Tree classifiers. Finally, the performance of all the classifiers is compared with respect to their accuracy. In addition, the results from the classifiers show that supervised learning classifiers perform excellently in sentiment classification.