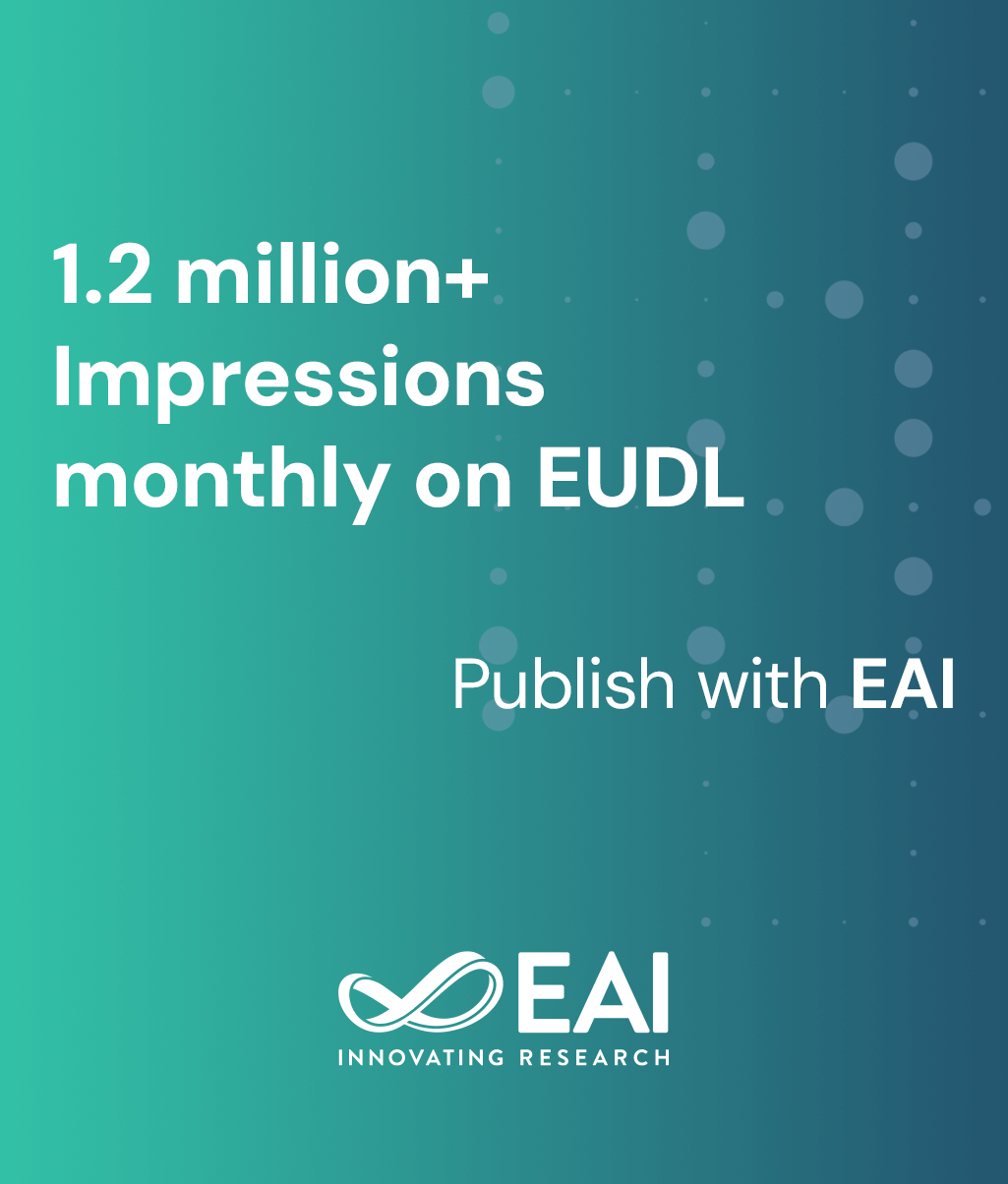
Research Article
Clinical Practice for Diagnostic Causes for Obstructive Sleep Apnea Using Artificial Intelligent Neural Networks
@INPROCEEDINGS{10.1007/978-3-319-95450-9_22, author={Mashail Alsalamah and Saad Amin and Vasile Palade}, title={Clinical Practice for Diagnostic Causes for Obstructive Sleep Apnea Using Artificial Intelligent Neural Networks}, proceedings={Emerging Technologies in Computing. First International Conference, iCETiC 2018, London, UK, August 23--24, 2018, Proceedings}, proceedings_a={ICETIC}, year={2018}, month={7}, keywords={Obstructive sleep apnea Deep learning Neural networks Sleep disorder Big data}, doi={10.1007/978-3-319-95450-9_22} }
- Mashail Alsalamah
Saad Amin
Vasile Palade
Year: 2018
Clinical Practice for Diagnostic Causes for Obstructive Sleep Apnea Using Artificial Intelligent Neural Networks
ICETIC
Springer
DOI: 10.1007/978-3-319-95450-9_22
Abstract
Sleep apnea is a serious sleep disorder phenomena which happens when a person’s breathing is paused during sleep. The most common diagnostic technique that is used to deal with sleep apnea is Polysomnography (PSG) which is done at special sleeping labs. This technique is expensive and uncomfortable. New automated methods have been developed for sleep apnea detection using artificial intelligence algorithms, which are more convenient and comfortable for patients. This paper proposes a novel scheme based on deep learning for sleep apnea detection and quantification using statistical features of ECG signals. The proposed approach is experimented with three phases: (1) minute-based apnea classification, (2) class identification and minute-by-minute detection for each ECG recording unlike state-of-the-art methods which either identify apnea class or detect its presence at each minute and (3) comparison of the proposed scheme with the well-known methods that have been proposed in the literature, which may have not used the same features and/or the same dataset. The obtained results demonstrate that the proposed approach provides significant performance improvements when compared to state-of-the-art methods. The outcome of this study can be used as an assistant tool by cardiologists to help them make more consistent diagnosis of sleep apnea disorder.