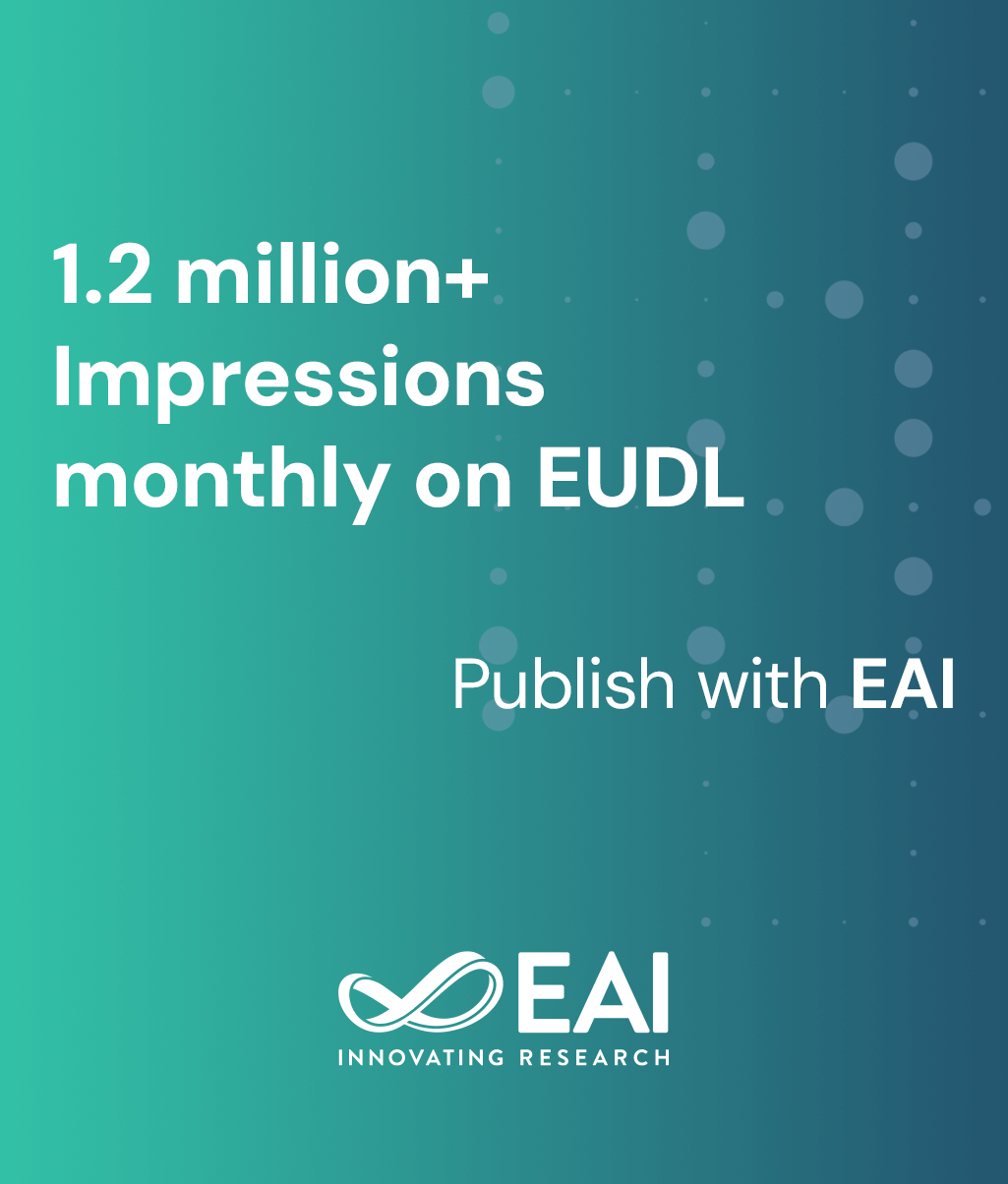
Research Article
Classification of Mammograms Using Convolutional Neural Network Based Feature Extraction
@INPROCEEDINGS{10.1007/978-3-319-95153-9_9, author={Taye Debelee and Mohammadreza Amirian and Achim Ibenthal and G\'{y}nther Palm and Friedhelm Schwenker}, title={Classification of Mammograms Using Convolutional Neural Network Based Feature Extraction}, proceedings={Information and Communication Technology for Development for Africa. First International Conference, ICT4DA 2017, Bahir Dar, Ethiopia, September 25--27, 2017, Proceedings}, proceedings_a={ICT4DA}, year={2018}, month={7}, keywords={Breast cancer Mammogram CNN K-nearest neighbour Feature extraction}, doi={10.1007/978-3-319-95153-9_9} }
- Taye Debelee
Mohammadreza Amirian
Achim Ibenthal
Günther Palm
Friedhelm Schwenker
Year: 2018
Classification of Mammograms Using Convolutional Neural Network Based Feature Extraction
ICT4DA
Springer
DOI: 10.1007/978-3-319-95153-9_9
Abstract
Breast cancer is the most common cause of death among women in the entire world and the second cause of death after lung cancer. The use of automatic breast cancer detection and classification might possibly enhance the survival rate of the patients through starting early treatment. In this paper, the convolutional Neural Networks (CNN) based feature extraction method is proposed. The features dimensionality was reduced using Principal Component Analysis (PCA). The reduced features are given to the K-Nearest Neighbors (KNN) to classify mammograms as normal or abnormal using 10-fold cross-validation. The experimental result of the proposed approach performed on Mammography Image Analysis Society (MIAS) and Digital Database for Screening Mammography (DDSM) datasets were found to be promising compared to previous studies in the area of image processing, artificial intelligence and CNN with an accuracy of 98.75 and 98.90 on MIAS and DDSM dataset respectively.