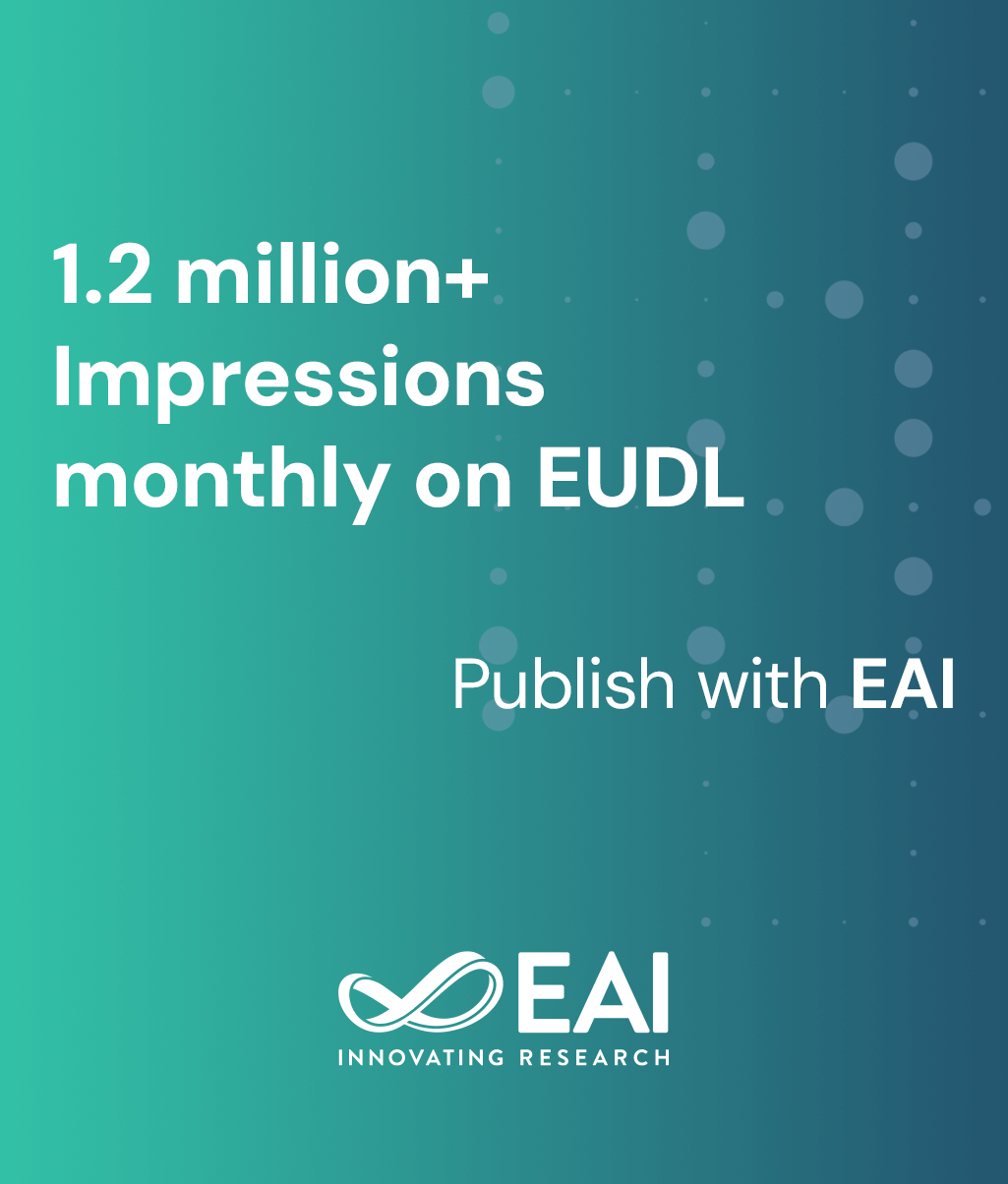
Research Article
Assessment of Machine Learning Algorithms for the Purpose of Primary Sjögren’s Syndrome Grade Classification from Segmented Ultrasonography Images
@INPROCEEDINGS{10.1007/978-3-319-92213-3_35, author={Arso Vukicevic and Alen Zabotti and Salvatore Vita and Nenad Filipovic}, title={Assessment of Machine Learning Algorithms for the Purpose of Primary Sj\o{}gren’s Syndrome Grade Classification from Segmented Ultrasonography Images}, proceedings={Future Access Enablers for Ubiquitous and Intelligent Infrastructures. Third International Conference, FABULOUS 2017, Bucharest, Romania, October 12-14, 2017, Proceedings}, proceedings_a={FABULOUS}, year={2018}, month={7}, keywords={Sj\o{}gren’s syndrome Classification Ultrasonography}, doi={10.1007/978-3-319-92213-3_35} }
- Arso Vukicevic
Alen Zabotti
Salvatore Vita
Nenad Filipovic
Year: 2018
Assessment of Machine Learning Algorithms for the Purpose of Primary Sjögren’s Syndrome Grade Classification from Segmented Ultrasonography Images
FABULOUS
Springer
DOI: 10.1007/978-3-319-92213-3_35
Abstract
Primary Sjögren’s syndrome (pSS) is a chronic autoimmune disease that affects primarily women (9 females/1 male). Recently, a great interest has arisen for salivary gland ultrasonography (SGUS) as a valuable tool for the assessment of major salivary gland involvement in primary Sjögren’s syndrome. The aim of this study was to assess accuracy of state of the art machine learning algorithms for the purpose of classifying pSS from SGUS images. The five-step procedure was carried out, including: image pre- processing, feature extraction, data set balancing and feature extraction, classifiers (K-Nearest Neighbour, Decision trees, Naive bayes, Discriminant analysis classifier, Random forest, Multilayer perceptron, Linear logistic regression) learning and their corresponding assessment. The preliminary results on the growing HarmonicSS cohort showed that Naive bayes (72.8% accuracy on training set, and 73.3% accuracy on test set) and Multilayer perceptron (85.0% accuracy in training stage, and 70.1% accuracy at test stage) are the most suitable for the purpose of pSS grade classification.