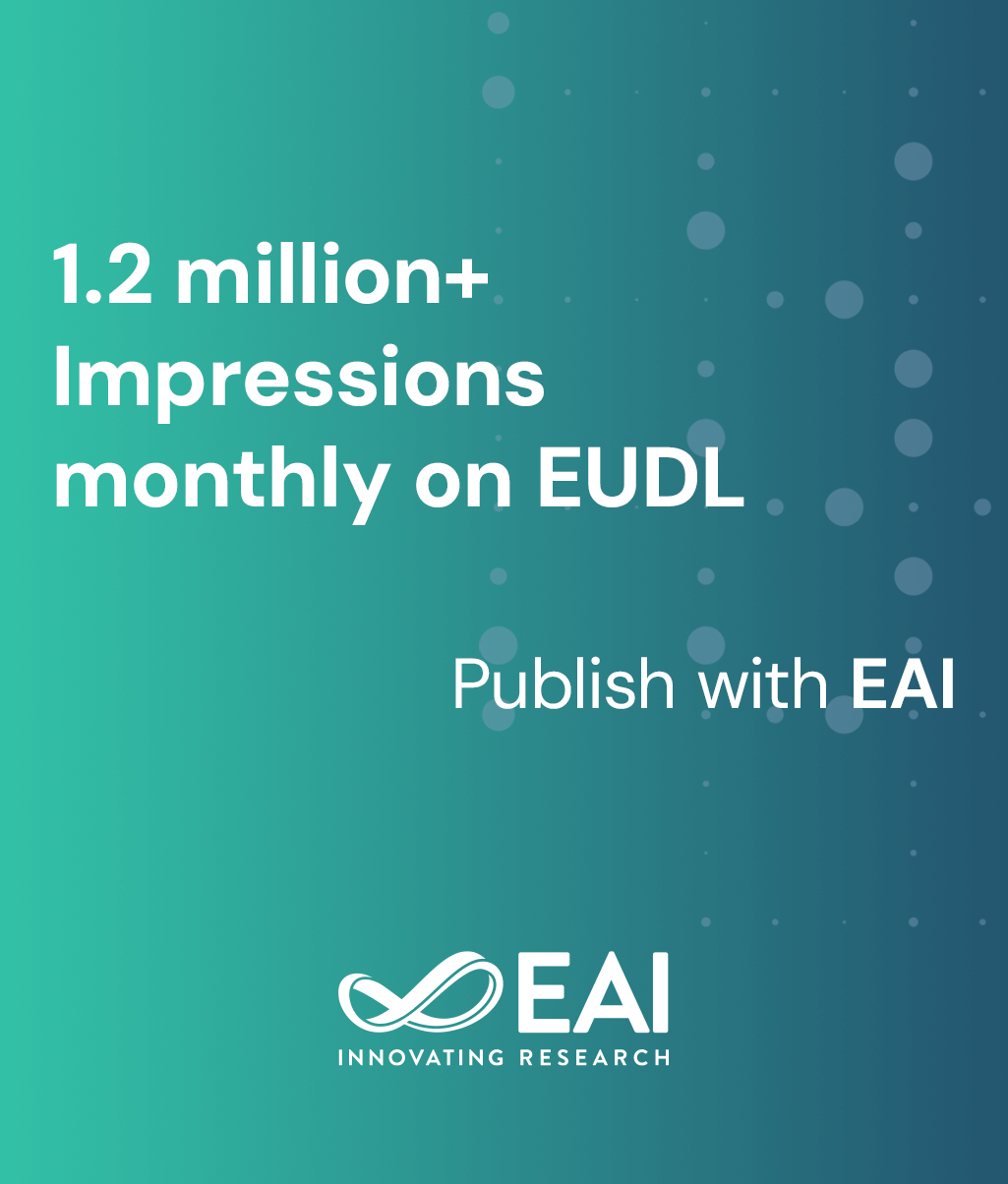
Research Article
Designing Anomaly Detection System for Cloud Servers by Frequency Domain Features of System Call Identifiers and Machine Learning
@INPROCEEDINGS{10.1007/978-3-319-90775-8_12, author={Waqas Haider and Jiankun Hu and Nour Moustafa}, title={Designing Anomaly Detection System for Cloud Servers by Frequency Domain Features of System Call Identifiers and Machine Learning}, proceedings={Mobile Networks and Management. 9th International Conference, MONAMI 2017, Melbourne, Australia, December 13-15, 2017, Proceedings}, proceedings_a={MONAMI}, year={2018}, month={5}, keywords={HIDS HADS Operating system security Intrusion detection}, doi={10.1007/978-3-319-90775-8_12} }
- Waqas Haider
Jiankun Hu
Nour Moustafa
Year: 2018
Designing Anomaly Detection System for Cloud Servers by Frequency Domain Features of System Call Identifiers and Machine Learning
MONAMI
Springer
DOI: 10.1007/978-3-319-90775-8_12
Abstract
The protection of operating systems from the current cyber threats has paramount importance. This importance is reflected by the functional dependency of any known or unknown cyber-attack upon the machines operating system. In order to design an anomaly detection system to protect an operating system from unknown attacks, acquiring comprehensive information related to running activities is the first crucial step. System call identifiers are one of the most reflective logs related to running activities in an operating system. Number of system call identifiers based host anomaly detection systems have been presented from the last two decades by using logs as raw system call identifiers. However, due to the stealth and penetration power of the unknown attacks, there is a need of acquiring and investigating more possible logs from machines operating system for the reliable protection. In this paper, firstly we apply the sine and Fourier transformation to the short sequence of system call identifiers, in order to model the frequency domain feature vector of any running activity at the cloud server. Second, different machine learning algorithms are trained and tested as anomaly detection engine using frequency domain transformed feature vectors of the short sequence of system call identifiers. The proposed work is evaluated using recently released intrusion detection systems data-set i.e., NGIDS-DS alongside two other old data-sets for comparative purposes. The experimental results indicate that the frequency domain feature vectors of short sequence of system call identifiers have comparatively superior performance than raw short sequence of system call identifiers, in detecting anomalies and building normal profile.