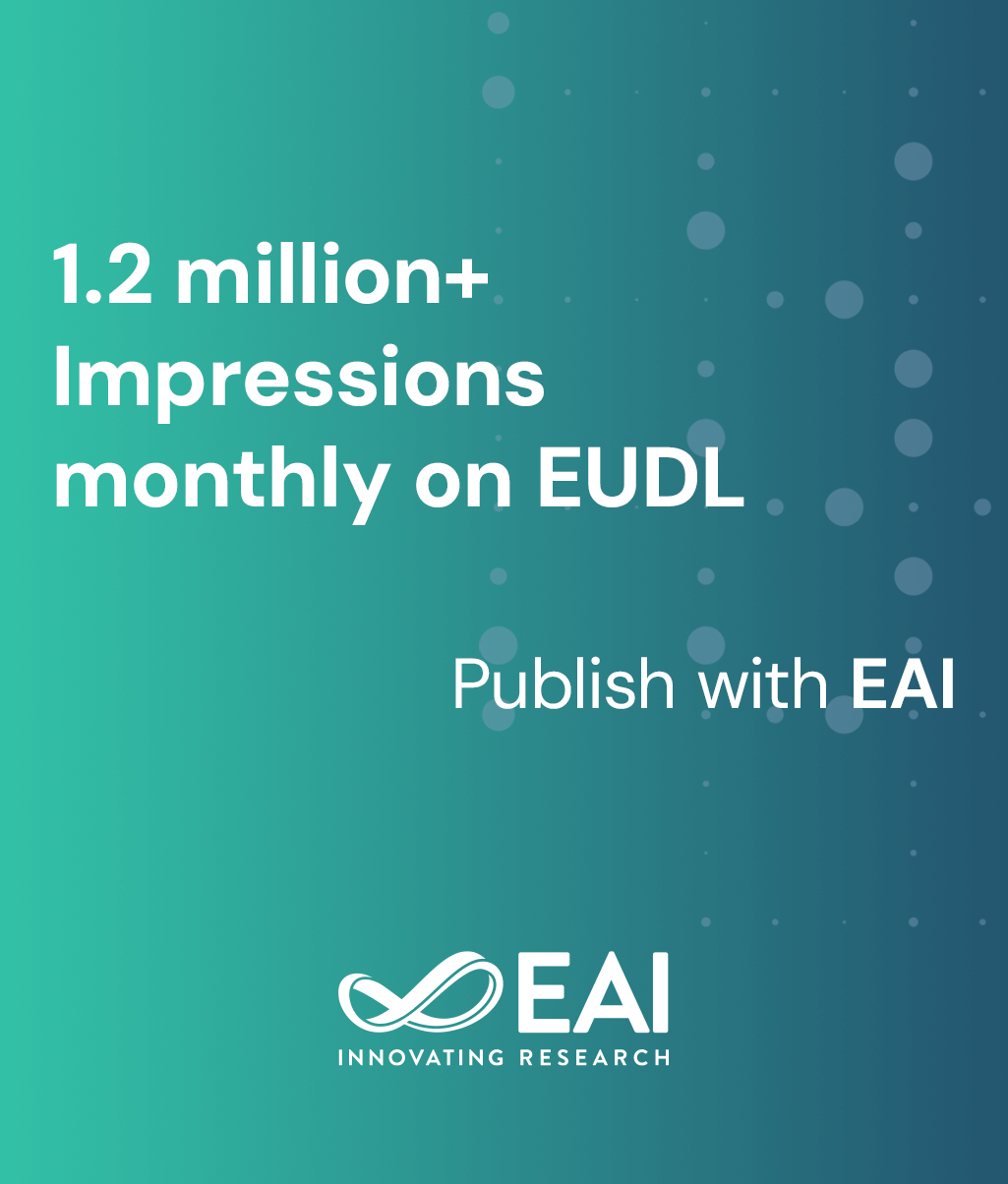
Research Article
Machine Learning of User Attentions in Sensor Data Visualization
@INPROCEEDINGS{10.1007/978-3-319-90740-6_8, author={Keita Fujino and Sozo Inoue and Tomohiro Shibata}, title={Machine Learning of User Attentions in Sensor Data Visualization}, proceedings={Mobile Computing, Applications, and Services. 9th International Conference, MobiCASE 2018, Osaka, Japan, February 28 -- March 2, 2018, Proceedings}, proceedings_a={MOBICASE}, year={2018}, month={5}, keywords={Sensor data Visualization User attention Attention points Machine learning}, doi={10.1007/978-3-319-90740-6_8} }
- Keita Fujino
Sozo Inoue
Tomohiro Shibata
Year: 2018
Machine Learning of User Attentions in Sensor Data Visualization
MOBICASE
Springer
DOI: 10.1007/978-3-319-90740-6_8
Abstract
In this paper, we propose a method for automatically estimating important points of large sensor data by collecting attention points of the user when visualized, and applying a supervised machine-learning algorithm. For large-scale sensor data, it is difficult to find important points simply through visualization, because such points are buried in a large scope of visualization. We also provide the results of an estimation, the accuracy of which was over 80% for multiple visualizations. In addition, the method has the advantage that the trained model can be reused to any other visualization from the same type of the sensors. We show the results of such reusability for the new type of visualization, which achieved an accuracy rate of 70–80%.