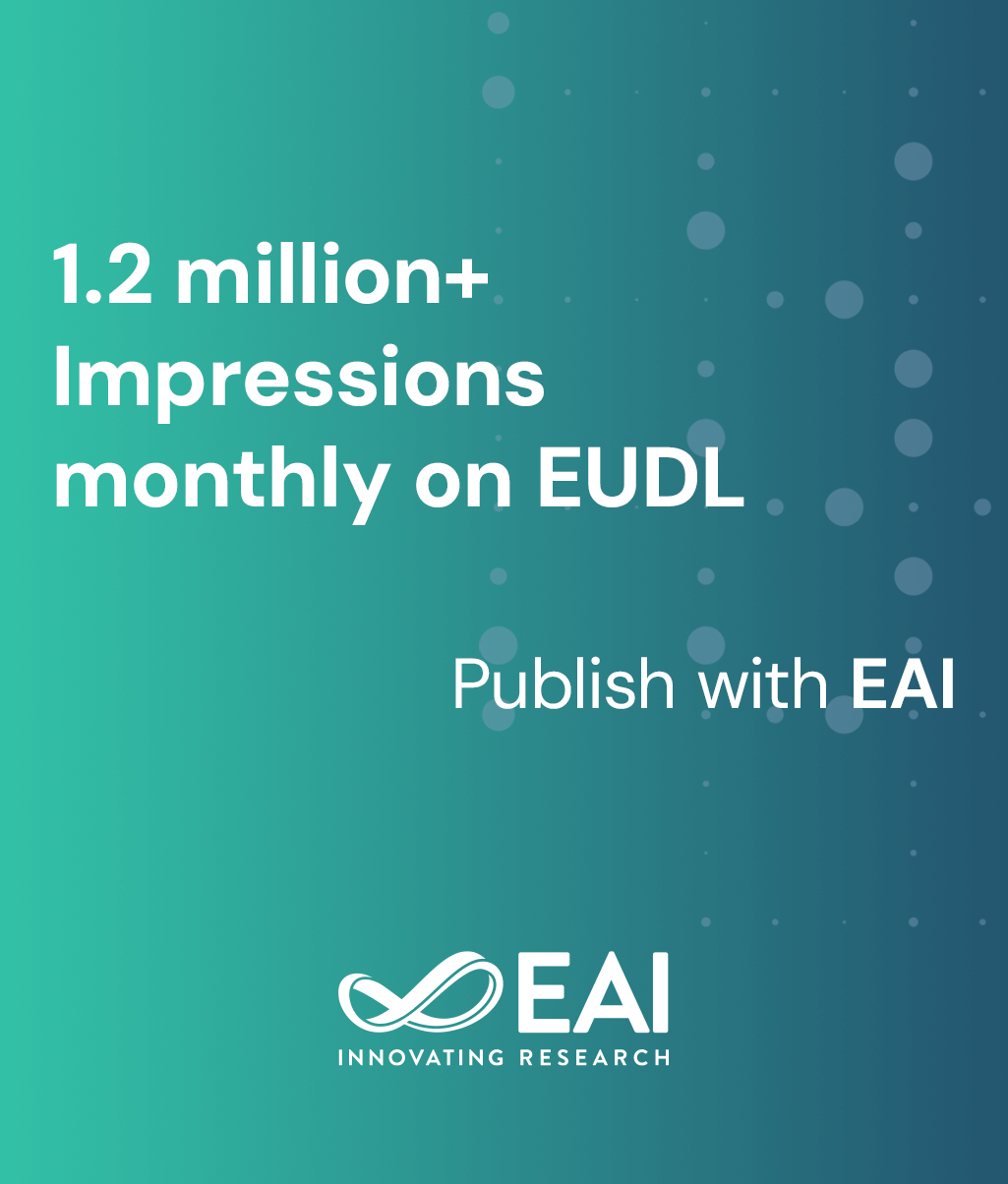
Research Article
Cross-site Input Inference Attacks on Mobile Web Users
@INPROCEEDINGS{10.1007/978-3-319-78813-5_32, author={Rui Zhao and Chuan Yue and Qi Han}, title={Cross-site Input Inference Attacks on Mobile Web Users}, proceedings={Security and Privacy in Communication Networks. 13th International Conference, SecureComm 2017, Niagara Falls, ON, Canada, October 22--25, 2017, Proceedings}, proceedings_a={SECURECOMM}, year={2018}, month={4}, keywords={Mobile Web Cross-site input inference Motion sensor}, doi={10.1007/978-3-319-78813-5_32} }
- Rui Zhao
Chuan Yue
Qi Han
Year: 2018
Cross-site Input Inference Attacks on Mobile Web Users
SECURECOMM
Springer
DOI: 10.1007/978-3-319-78813-5_32
Abstract
In this paper, we investigate severe cross-site input inference attacks that may compromise the security of every mobile Web user, and quantify the extent to which they can be effective. We formulate our attacks as a typical multi-class classification problem, and build an inference framework that trains a classifier in the training phase and predicts a user’s new inputs in the attacking phase. To make our attacks effective and realistic, we design unique techniques, and address major data quality and data segmentation challenges. We intensively evaluate the effectiveness of our attacks using keystrokes collected from 20 participants. Overall, our attacks are effective, for example, they are about 10.8 times more effective than the random guessing attacks regarding inferring letters. Our results demonstrate that researchers, smartphone vendors, and app developers should pay serious attention to the severe cross-site input inference attacks that can be pervasively performed, and should start to design and deploy effective defense techniques.