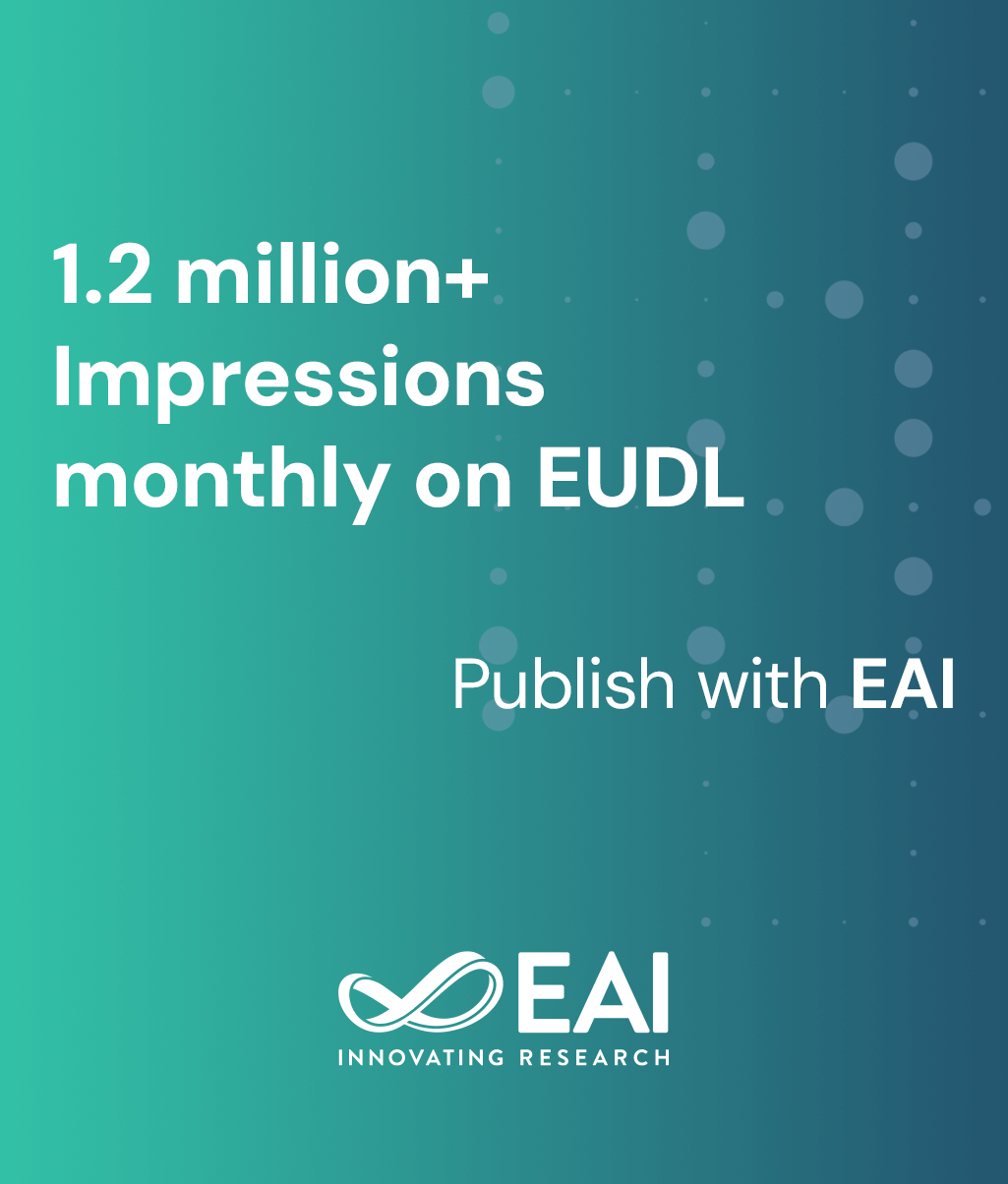
Research Article
HFA-MD: An Efficient Hybrid Features Analysis Based Android Malware Detection Method
@INPROCEEDINGS{10.1007/978-3-319-78078-8_25, author={Yang Zhao and Guangquan Xu and Yao Zhang}, title={HFA-MD: An Efficient Hybrid Features Analysis Based Android Malware Detection Method}, proceedings={Quality, Reliability, Security and Robustness in Heterogeneous Systems. 13th International Conference, QShine 2017, Dalian, China, December 16 -17, 2017, Proceedings}, proceedings_a={QSHINE}, year={2018}, month={4}, keywords={Android malware detection Machine learning Static analysis Dynamic analysis Feature selection}, doi={10.1007/978-3-319-78078-8_25} }
- Yang Zhao
Guangquan Xu
Yao Zhang
Year: 2018
HFA-MD: An Efficient Hybrid Features Analysis Based Android Malware Detection Method
QSHINE
Springer
DOI: 10.1007/978-3-319-78078-8_25
Abstract
Lack of supervision and management of many Android third-party application markets has led to a growing number of malware on android platforms. This causes a serious privacy threat to the user’s sensitive information. To solve this problem, in this paper, a new hybrid features analysis method aiming at Android malware detection is proposed, which obtains a hybrid feature vector by extracting the information of permission requests, API calls and runtime behaviors. The characteristic of this work is the use of machine learning classification algorithms to detect malicious software. In addition, the feature selection algorithm is used to further optimize the extracted information to remove some useless features. Our experiments are based on real-world Apps, and use five different classification algorithms to detect the malware. The experiment results show that our proposed hybrid feature extraction method can improve the accuracy rate of Android malware detection compared with using static methods alone.