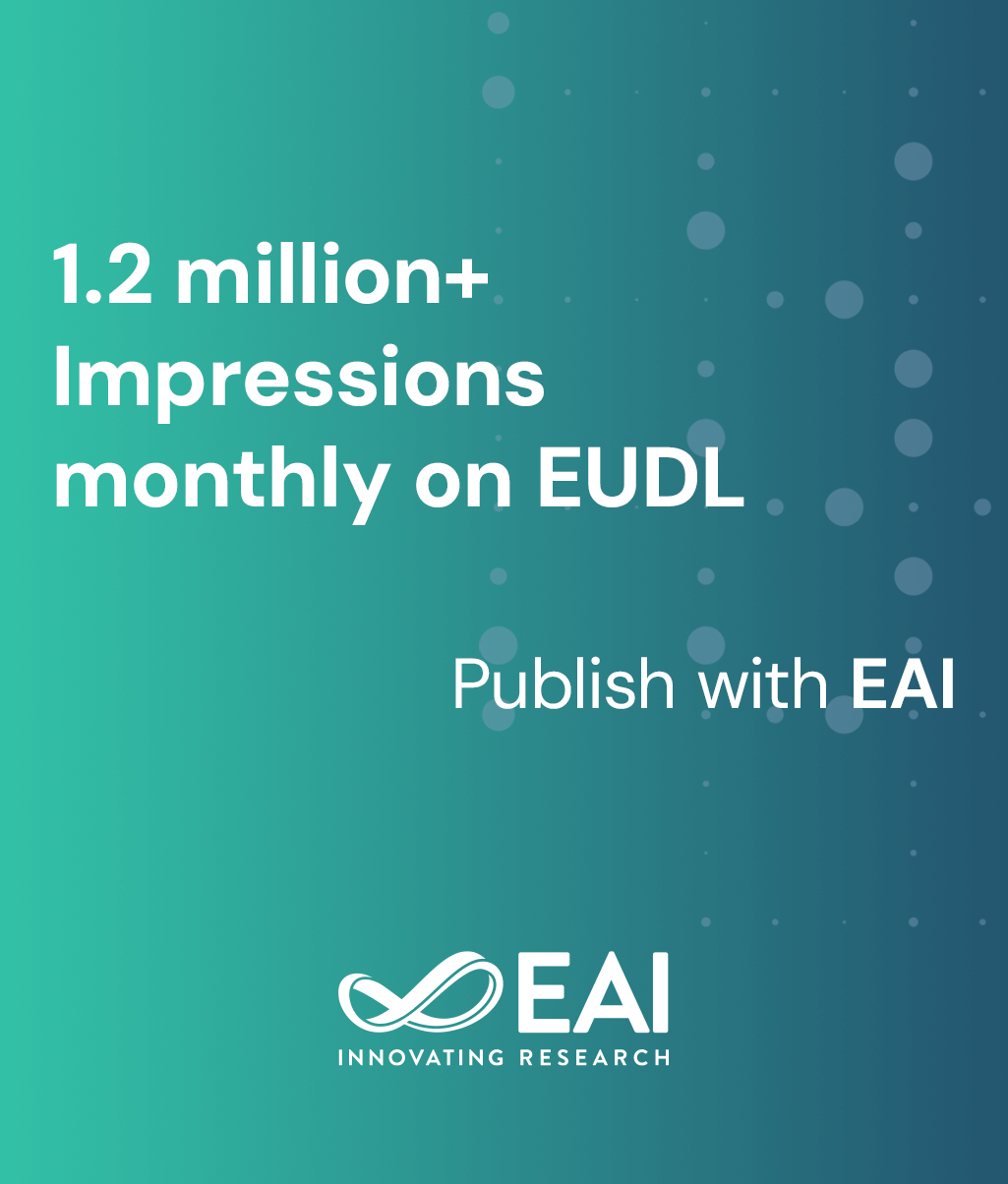
Research Article
Traffic Incident Recognition Using Empirical Deep Convolutional Neural Networks Model
@INPROCEEDINGS{10.1007/978-3-319-77818-1_9, author={Nam Vu and Cuong Pham}, title={Traffic Incident Recognition Using Empirical Deep Convolutional Neural Networks Model}, proceedings={Context-Aware Systems and Applications, and Nature of Computation and Communication. 6th International Conference, ICCASA 2017, and 3rd International Conference, ICTCC 2017, Tam Ky, Vietnam, November 23-24, 2017, Proceedings}, proceedings_a={ICCASA \& ICTCC}, year={2018}, month={3}, keywords={Convolutional neural network Traffic incident Vehicle detection}, doi={10.1007/978-3-319-77818-1_9} }
- Nam Vu
Cuong Pham
Year: 2018
Traffic Incident Recognition Using Empirical Deep Convolutional Neural Networks Model
ICCASA & ICTCC
Springer
DOI: 10.1007/978-3-319-77818-1_9
Abstract
Traffic incident detection plays an important role for a broad range of intelligent transport systems and applications such as driver- assistant, accident warning, and traffic data analysis. The primary goal of traffic incident detection systems in real-world is to identify traffic violations happening on the road in real-time. Although research community has made a significant attempt for detecting on-road violations, there are still challenges such as poor performance under real-world circumstances and real-time detection. In this paper, we propose a novel method which utilizes the powerful deep convolutional neural networks for vehicle recognition task to detect traffic events on the separate lane. Experimental results on real-world dataset videos as well as live stream in real-time from digital cameras demonstrate the feasibility and effectiveness of the proposed method for identifying incidents under various conditions of urban roads and highways.