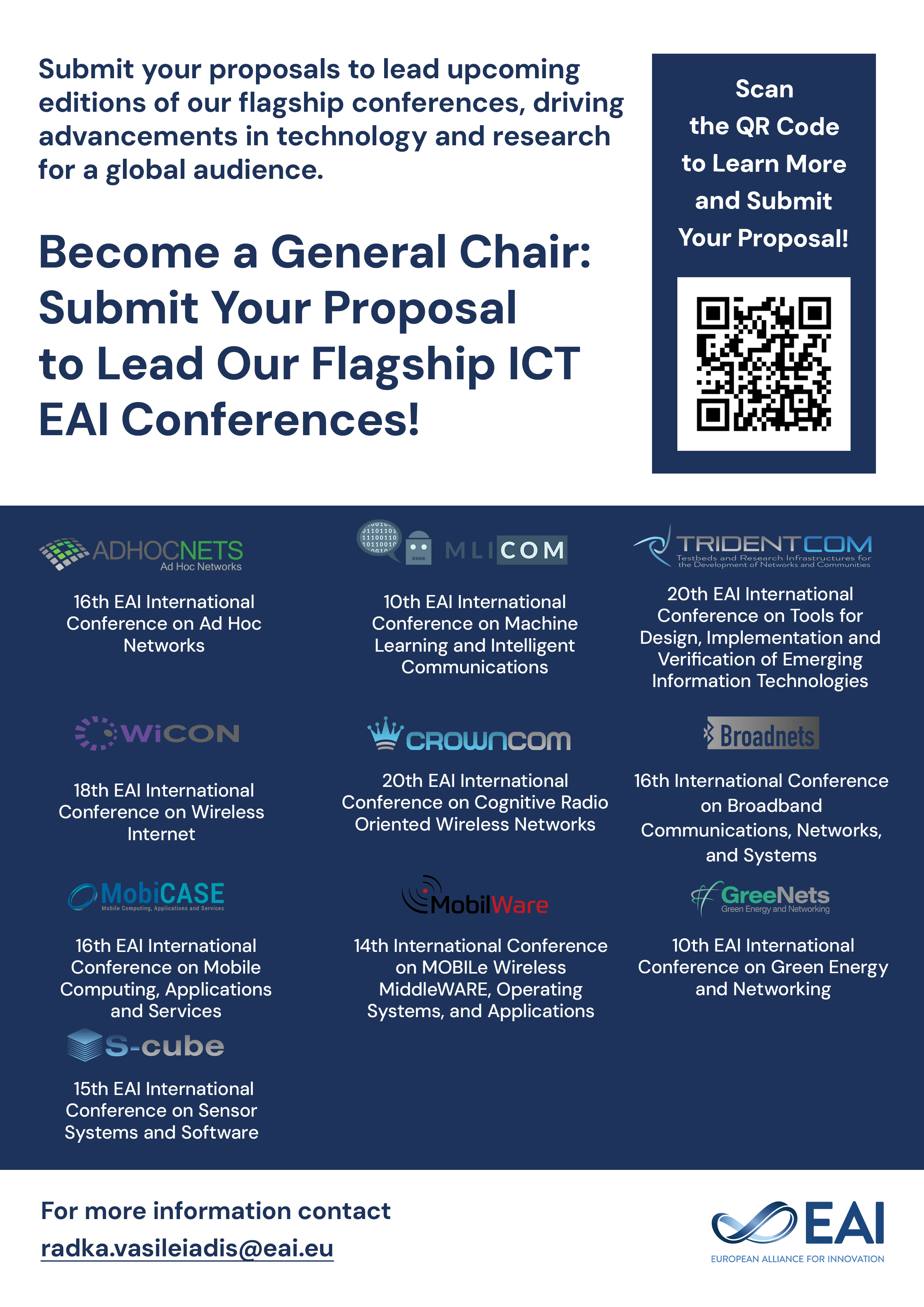
Research Article
An Efficient Design of a Machine Learning-Based Elderly Fall Detector
@INPROCEEDINGS{10.1007/978-3-319-76213-5_5, author={L. Nguyen and M. Saleh and R. Bouquin Jeann\'{e}s}, title={An Efficient Design of a Machine Learning-Based Elderly Fall Detector}, proceedings={Internet of Things (IoT) Technologies for HealthCare. 4th International Conference, HealthyIoT 2017, Angers, France, October 24-25, 2017, Proceedings}, proceedings_a={HEALTHYIOT}, year={2018}, month={2}, keywords={Elderly fall detection Micro electro mechanical system Inertial measurement unit Support vector machine Multi-layer perceptron K-nearest neighbors}, doi={10.1007/978-3-319-76213-5_5} }
- L. Nguyen
M. Saleh
R. Bouquin Jeannès
Year: 2018
An Efficient Design of a Machine Learning-Based Elderly Fall Detector
HEALTHYIOT
Springer
DOI: 10.1007/978-3-319-76213-5_5
Abstract
Elderly fall detection is an important health care application as falls represent the major reason of injuries. An efficient design of a machine learning-based wearable fall detection system is proposed in this paper. The proposed system depends only on a 3-axial accelerometer to capture the elderly motion. As the power consumption is proportional to the sampling frequency, the performance of the proposed fall detector is analyzed as a function of this frequency in order to determine the best trade-off between performance and power consumption. Thanks to efficient extracted features, the proposed system achieves a sensitivity of 99.73% and a specificity of 97.7% using a 40 Hz sampling frequency notably outperforming reference algorithms when tested on a large dataset.