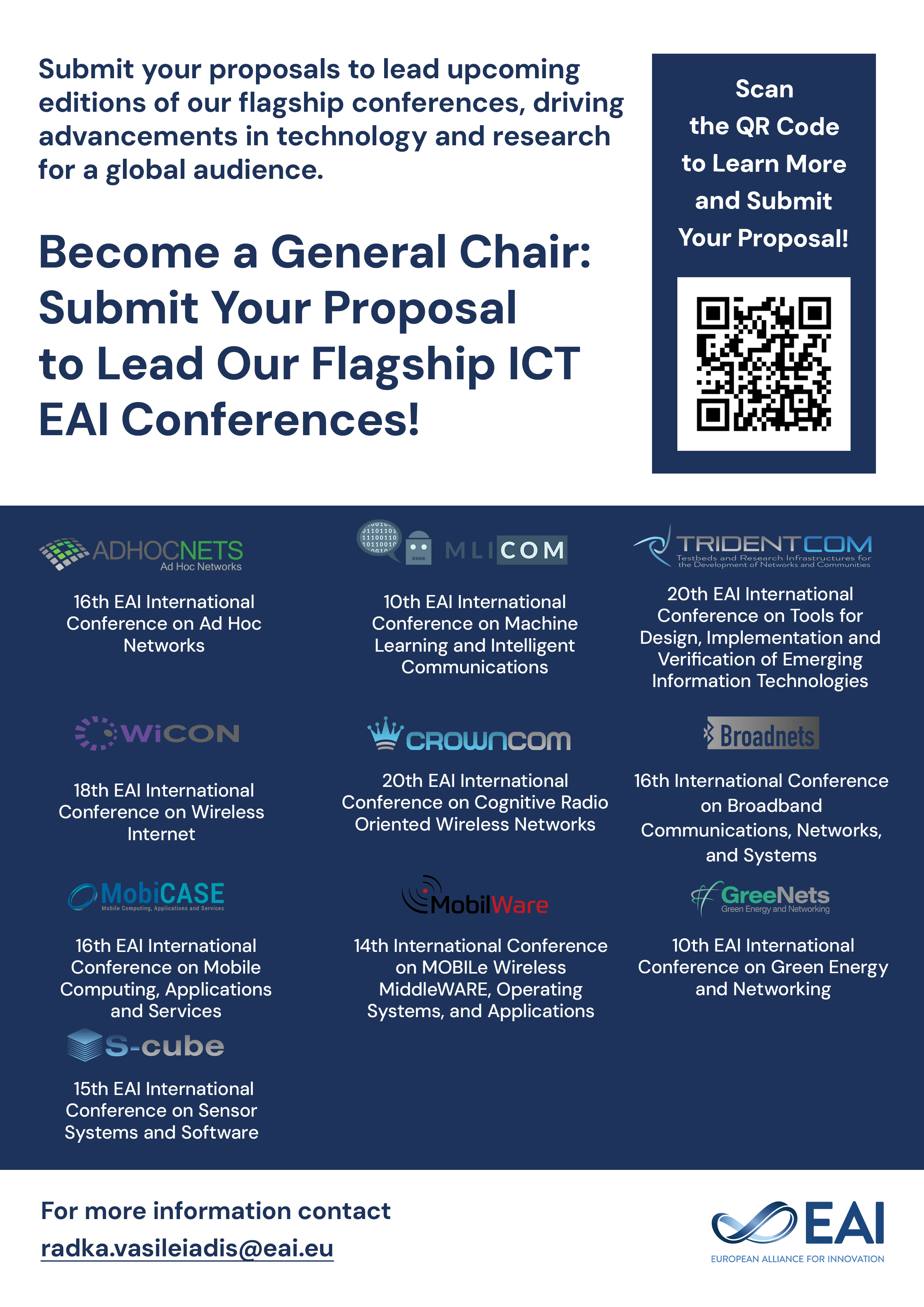
Research Article
Distributed Multivariate Physiological Signal Analytics for Drivers’ Mental State Monitoring
@INPROCEEDINGS{10.1007/978-3-319-76213-5_4, author={Shaibal Barua and Mobyen Ahmed and Shahina Begum}, title={Distributed Multivariate Physiological Signal Analytics for Drivers’ Mental State Monitoring}, proceedings={Internet of Things (IoT) Technologies for HealthCare. 4th International Conference, HealthyIoT 2017, Angers, France, October 24-25, 2017, Proceedings}, proceedings_a={HEALTHYIOT}, year={2018}, month={2}, keywords={Physiological signals Distributed analytics Case-based reasoning}, doi={10.1007/978-3-319-76213-5_4} }
- Shaibal Barua
Mobyen Ahmed
Shahina Begum
Year: 2018
Distributed Multivariate Physiological Signal Analytics for Drivers’ Mental State Monitoring
HEALTHYIOT
Springer
DOI: 10.1007/978-3-319-76213-5_4
Abstract
This paper presents a distributed data analytics approach for drivers’ mental state monitoring using multivariate physiological signals. Driver’s mental states such as cognitive distraction, sleepiness, stress, etc. can be fatal contributing factors and to prevent car crashes these factors need to be understood. Here, a cloud-based approach with heterogeneous sensor sources that generates extremely large data sets of physiological signals need to be handled and analysed in a big data scenario. In the proposed physiological big data analytics approach, for driver state monitoring, heterogeneous data coming from multiple sources i.e., multivariate physiological signals are used, processed and analyzed to aware impaired vehicle drivers. Here, in a distributed big data environment, multi-agent case-based reasoning facilitates parallel case similarity matching and handles data that are coming from single and multiple physiological signal sources.