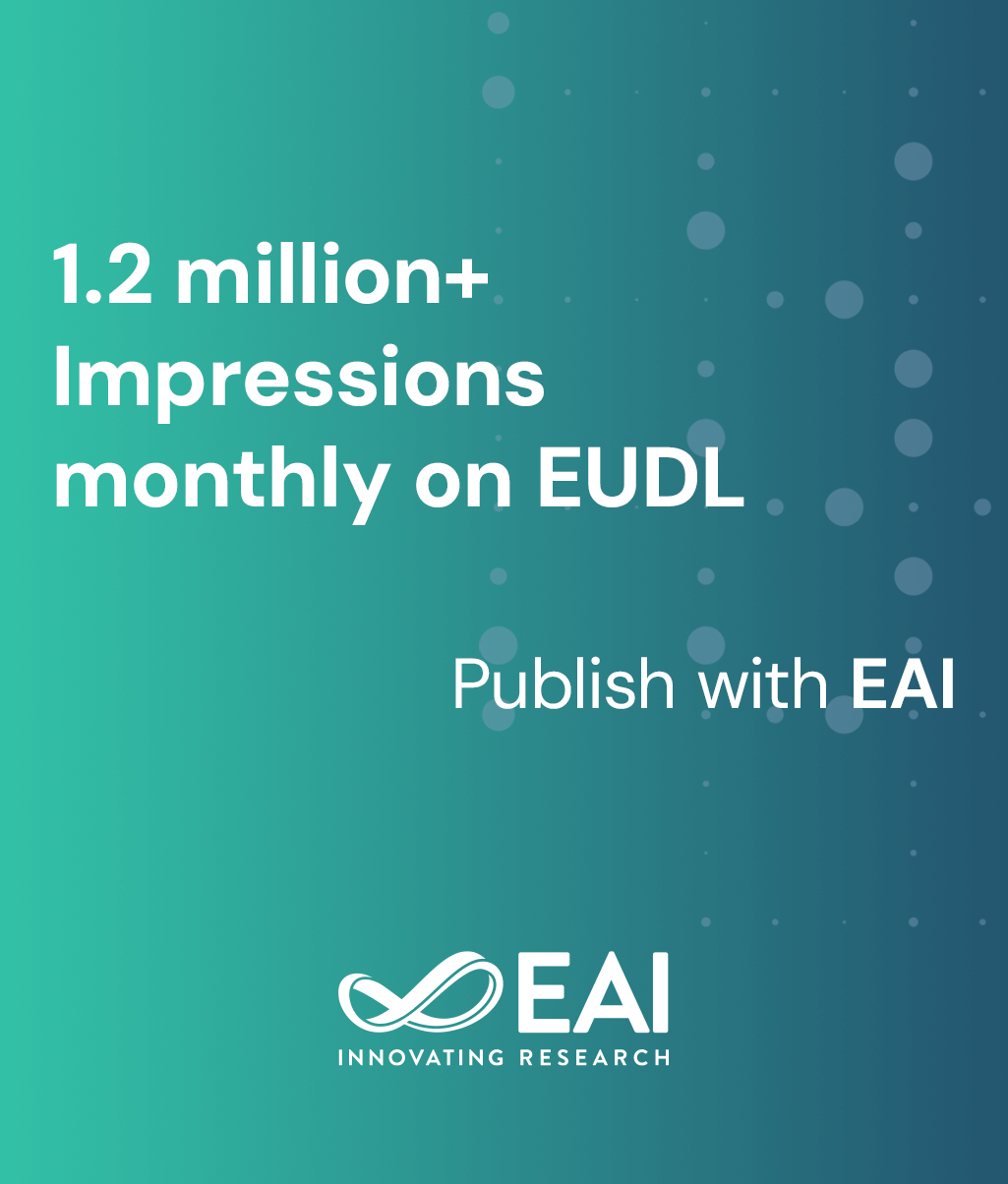
Research Article
Machine Learning-Aided Radio Scenario Recognition for Cognitive Radio Networks in Millimeter-Wave Bands
@INPROCEEDINGS{10.1007/978-3-319-76207-4_5, author={Jingyun Wang and Youping Zhao and Xin Guo and Chen Sun}, title={Machine Learning-Aided Radio Scenario Recognition for Cognitive Radio Networks in Millimeter-Wave Bands}, proceedings={Cognitive Radio Oriented Wireless Networks. 12th International Conference, CROWNCOM 2017, Lisbon, Portugal, September 20-21, 2017, Proceedings}, proceedings_a={CROWNCOM}, year={2018}, month={3}, keywords={BP-AdaBoost Channel condition recognition Cognitive radio networks Machine learning Radio scenario recognition}, doi={10.1007/978-3-319-76207-4_5} }
- Jingyun Wang
Youping Zhao
Xin Guo
Chen Sun
Year: 2018
Machine Learning-Aided Radio Scenario Recognition for Cognitive Radio Networks in Millimeter-Wave Bands
CROWNCOM
Springer
DOI: 10.1007/978-3-319-76207-4_5
Abstract
Radio scenario recognition is critically important to acquire comprehensive situation awareness for cognitive radio networks in the millimeter-wave bands, especially for dense small cell environment. In this paper, a generic framework of machine learning-aided radio scenario recognition scheme is proposed to acquire the environmental awareness. Particularly, an advanced back propagation neural network-based AdaBoost classification algorithm is developed to recognize various radio scenarios, in which different channel conditions such as line-of-sight (LOS), non-line-of-sight (NLOS), and obstructed line-of-sight (OLOS) are encountered by the desired signal or co-channel interference. Moreover, the advanced AdaBoost algorithm takes the offline training performance into account during the decision fusion. Simulation results show that machine learning can be exploited to recognize the complicated radio scenarios reliably and promptly.