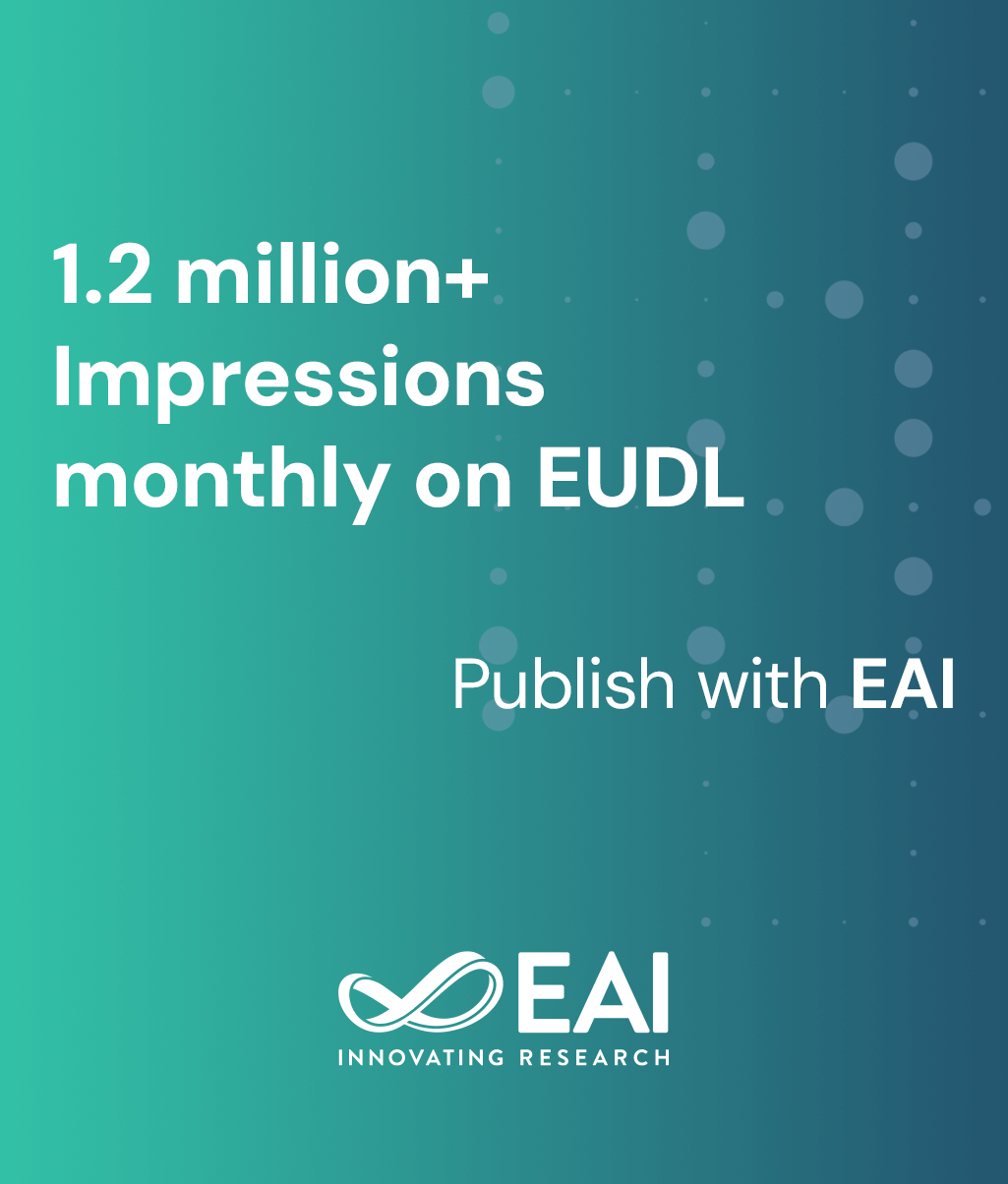
Research Article
Meet an Fantastic Sibyl: A Powerful Model in Cognitive Radio Networks
@INPROCEEDINGS{10.1007/978-3-319-76207-4_2, author={Wei Yang and Xiaojun Jing and Hai Huang}, title={Meet an Fantastic Sibyl: A Powerful Model in Cognitive Radio Networks}, proceedings={Cognitive Radio Oriented Wireless Networks. 12th International Conference, CROWNCOM 2017, Lisbon, Portugal, September 20-21, 2017, Proceedings}, proceedings_a={CROWNCOM}, year={2018}, month={3}, keywords={Dynamic spectrum access Linear prediction AR-PUER CRNs}, doi={10.1007/978-3-319-76207-4_2} }
- Wei Yang
Xiaojun Jing
Hai Huang
Year: 2018
Meet an Fantastic Sibyl: A Powerful Model in Cognitive Radio Networks
CROWNCOM
Springer
DOI: 10.1007/978-3-319-76207-4_2
Abstract
Dynamic spectrum access is challenging, since an individual secondary user usually just has limited sensing abilities. One key insight is that primary user emergence forecasting among secondary users can help to make the most of the inherent association structure in both time and space, it also enables users to obtain more informed spectrum opportunities. Therefore, primary user presence forecasting is vital to cognitive radio networks (CRNs). With this insight, an auto regressive enhanced primary user emergence reasoning (AR-PUER) model for the occurrence of primary user prediction is derived in this paper. The proposed method combines linear prediction and primary user emergence reasoning. Historical samples are selected to train the AR-PUER model in order to capture the current distinction pattern of primary user. The training samples of the primary user emergence reasoning (PUER) model are combined with the recent samples of auto regressive (AR) model tracking recent parallel. Our scheme does not require the knowledge of the signal or of the noise power. Furthermore, the proposed model in this paper is blind in the detection that it does not require information about the channel. To verify the performance of the proposed model, we apply it to the data during the past two months, and then compare it with other method. The simulation results demonstrate that the AR-PUER model is effective and generates the most accurate forecasting of primary user occasion in several cases. Besides, it also performs much better than the commonly used energy detector, which usually suffers from the noise uncertainty problem.