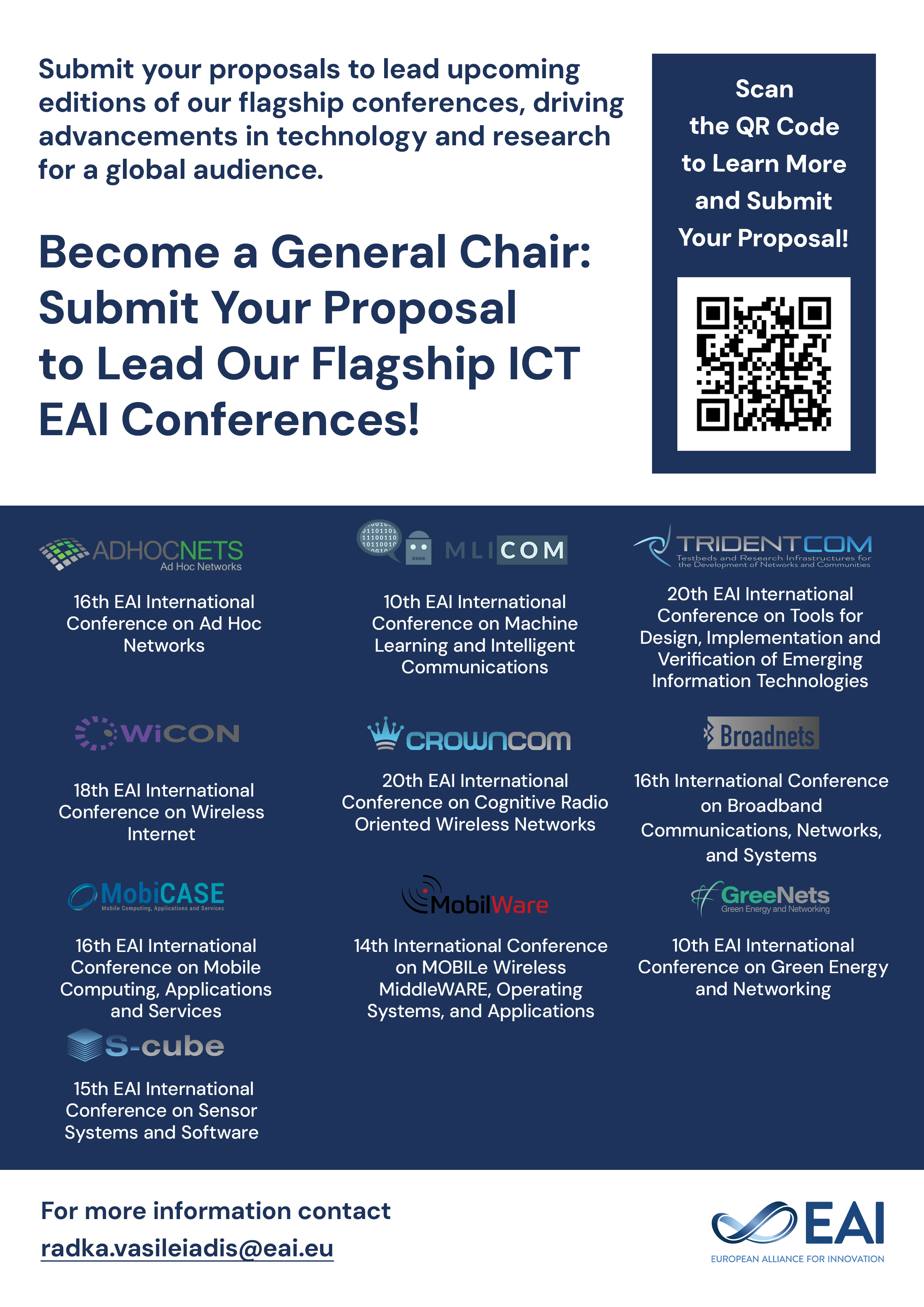
Research Article
Parkinson’s Disease Detection from Speech Using Convolutional Neural Networks
@INPROCEEDINGS{10.1007/978-3-319-76111-4_21, author={Evaldas Vaiciukynas and Adas Gelzinis and Antanas Verikas and Marija Bacauskiene}, title={Parkinson’s Disease Detection from Speech Using Convolutional Neural Networks}, proceedings={Smart Objects and Technologies for Social Good. Third International Conference, GOODTECHS 2017, Pisa, Italy, November 29-30, 2017, Proceedings}, proceedings_a={GOODTECHS}, year={2018}, month={3}, keywords={Parkinson’s disease Audio signal processing Convolutional neural network Information fusion}, doi={10.1007/978-3-319-76111-4_21} }
- Evaldas Vaiciukynas
Adas Gelzinis
Antanas Verikas
Marija Bacauskiene
Year: 2018
Parkinson’s Disease Detection from Speech Using Convolutional Neural Networks
GOODTECHS
Springer
DOI: 10.1007/978-3-319-76111-4_21
Abstract
Application of deep learning tends to outperform hand-crafted features in many domains. This study uses convolutional neural networks to explore effectiveness of various segments of a speech signal, – text-dependent pronunciation of a short sentence, – in Parkinson’s disease detection task. Besides the common Mel-frequency spectrogram and its first and second derivatives, inclusion of various other input feature maps is also considered. Image interpolation is investigated as a solution to obtain a spectrogram of fixed length. The equal error rate (EER) for sentence segments varied from 20.3% to 29.5%. Fusion of decisions from sentence segments achieved EER of 14.1%, whereas the best result when using the full sentence exhibited EER of 16.8%. Therefore, splitting speech into segments could be recommended for Parkinson’s disease detection.