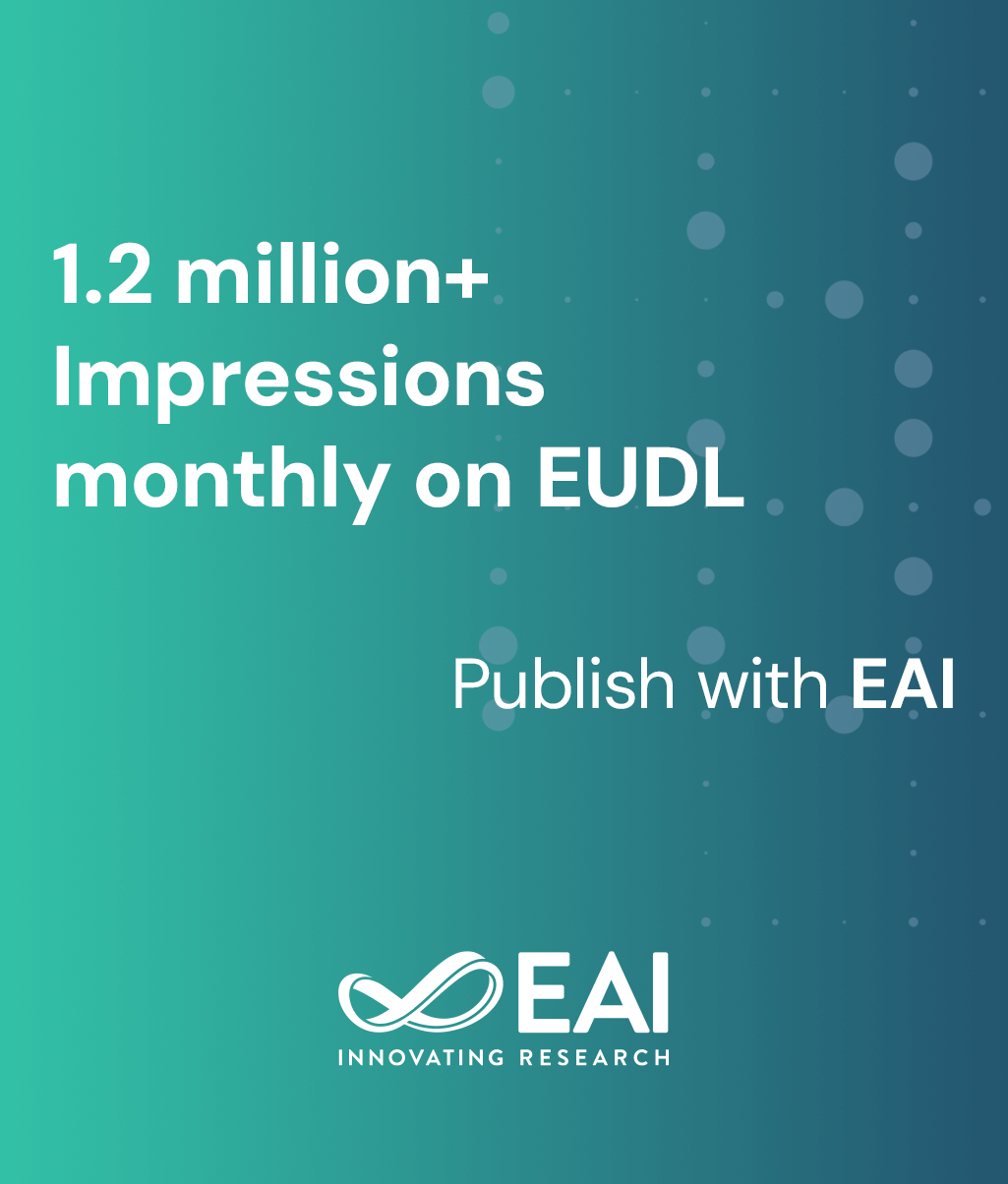
Research Article
Privacy Preserving Multidimensional Profiling
@INPROCEEDINGS{10.1007/978-3-319-76111-4_15, author={Francesca Pratesi and Anna Monreale and Fosca Giannotti and Dino Pedreschi}, title={Privacy Preserving Multidimensional Profiling}, proceedings={Smart Objects and Technologies for Social Good. Third International Conference, GOODTECHS 2017, Pisa, Italy, November 29-30, 2017, Proceedings}, proceedings_a={GOODTECHS}, year={2018}, month={3}, keywords={Privacy risk assessment Mobile phone data Retail data}, doi={10.1007/978-3-319-76111-4_15} }
- Francesca Pratesi
Anna Monreale
Fosca Giannotti
Dino Pedreschi
Year: 2018
Privacy Preserving Multidimensional Profiling
GOODTECHS
Springer
DOI: 10.1007/978-3-319-76111-4_15
Abstract
Recently, big data had become central in the analysis of human behavior and the development of innovative services. In particular, a new class of services is emerging, taking advantage of different sources of data, in order to consider the multiple aspects of human beings. Unfortunately, these data can lead to re-identification problems and other privacy leaks, as diffusely reported in both scientific literature and media. The risk is even more pressing if multiple sources of data are linked together since a potential adversary could know information related to each dataset. For this reason, it is necessary to evaluate accurately and mitigate the individual privacy risk before releasing personal data. In this paper, we propose a methodology for the first task, i.e., assessing privacy risk, in a multidimensional scenario, defining some possible privacy attacks and simulating them using real-world datasets.