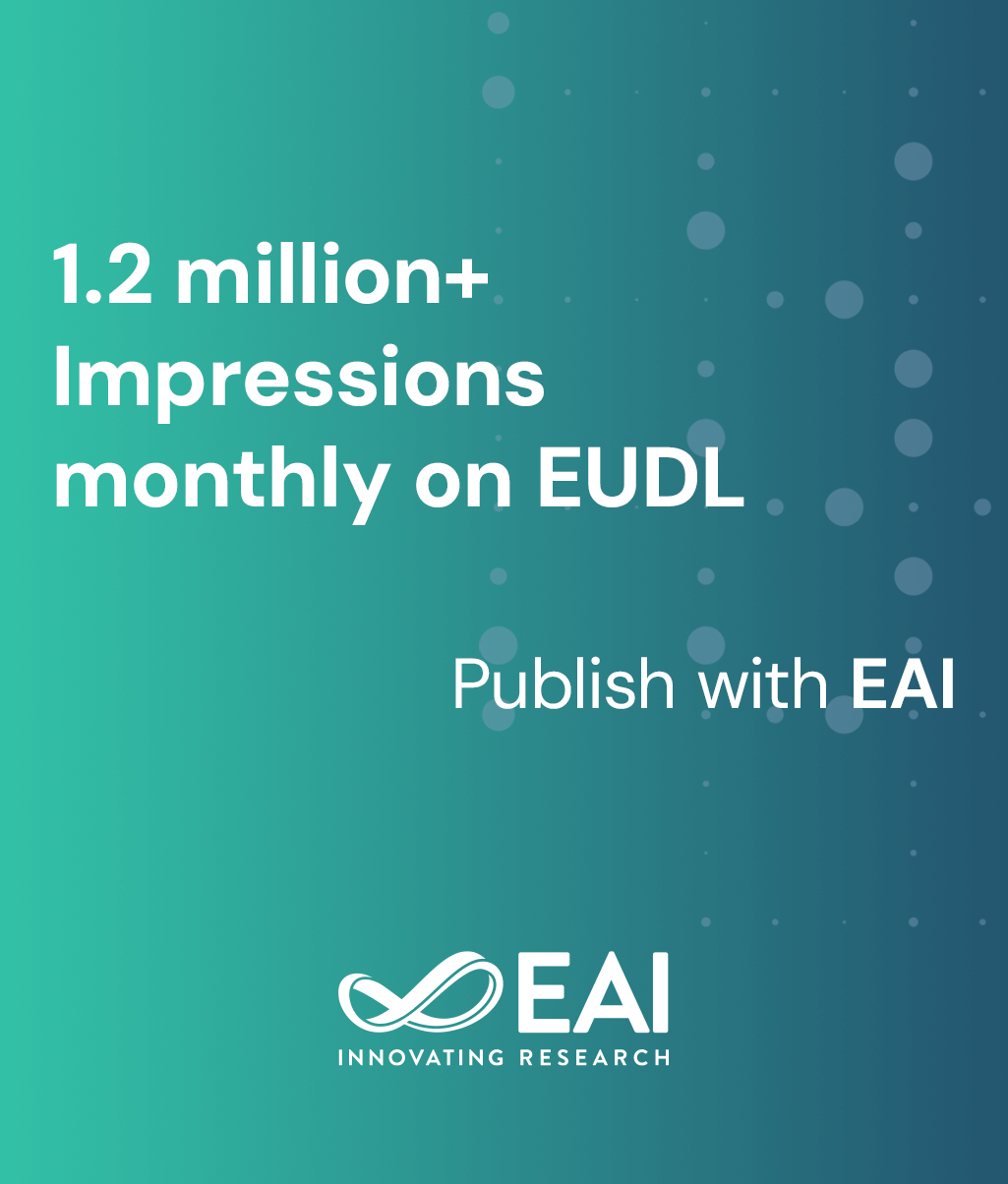
Research Article
An Intrusion Detection System Based on Machine Learning for CAN-Bus
720 downloads
@INPROCEEDINGS{10.1007/978-3-319-74176-5_25, author={Daxin Tian and Yuzhou Li and Yunpeng Wang and Xuting Duan and Congyu Wang and Wenyang Wang and Rong Hui and Peng Guo}, title={An Intrusion Detection System Based on Machine Learning for CAN-Bus}, proceedings={Industrial Networks and Intelligent Systems. 3rd International Conference, INISCOM 2017, Ho Chi Minh City, Vietnam, September 4, 2017, Proceedings}, proceedings_a={INISCOM}, year={2018}, month={1}, keywords={CAN-Bus Information security IDS Machine learning GBDT Entropy Detection performance}, doi={10.1007/978-3-319-74176-5_25} }
- Daxin Tian
Yuzhou Li
Yunpeng Wang
Xuting Duan
Congyu Wang
Wenyang Wang
Rong Hui
Peng Guo
Year: 2018
An Intrusion Detection System Based on Machine Learning for CAN-Bus
INISCOM
Springer
DOI: 10.1007/978-3-319-74176-5_25
Abstract
The CAN-Bus is currently the most widely used vehicle bus network technology, but it is designed for needs of vehicle control system, having massive data and lacking of information security mechanisms and means. The Intrusion Detection System (IDS) based on machine learning is an efficient active information security defense method and suitable for massive data processing. We use a machine learning algorithm—Gradient Boosting Decision Tree (GBDT) in IDS for CAN-Bus and propose a new feature based on entropy as the feature construction of GBDT algorithm. In detection performance, the IDS based on GBDT has a high True Positive (TP) rate and a low False Positive (FP) rate.
Copyright © 2017–2025 EAI