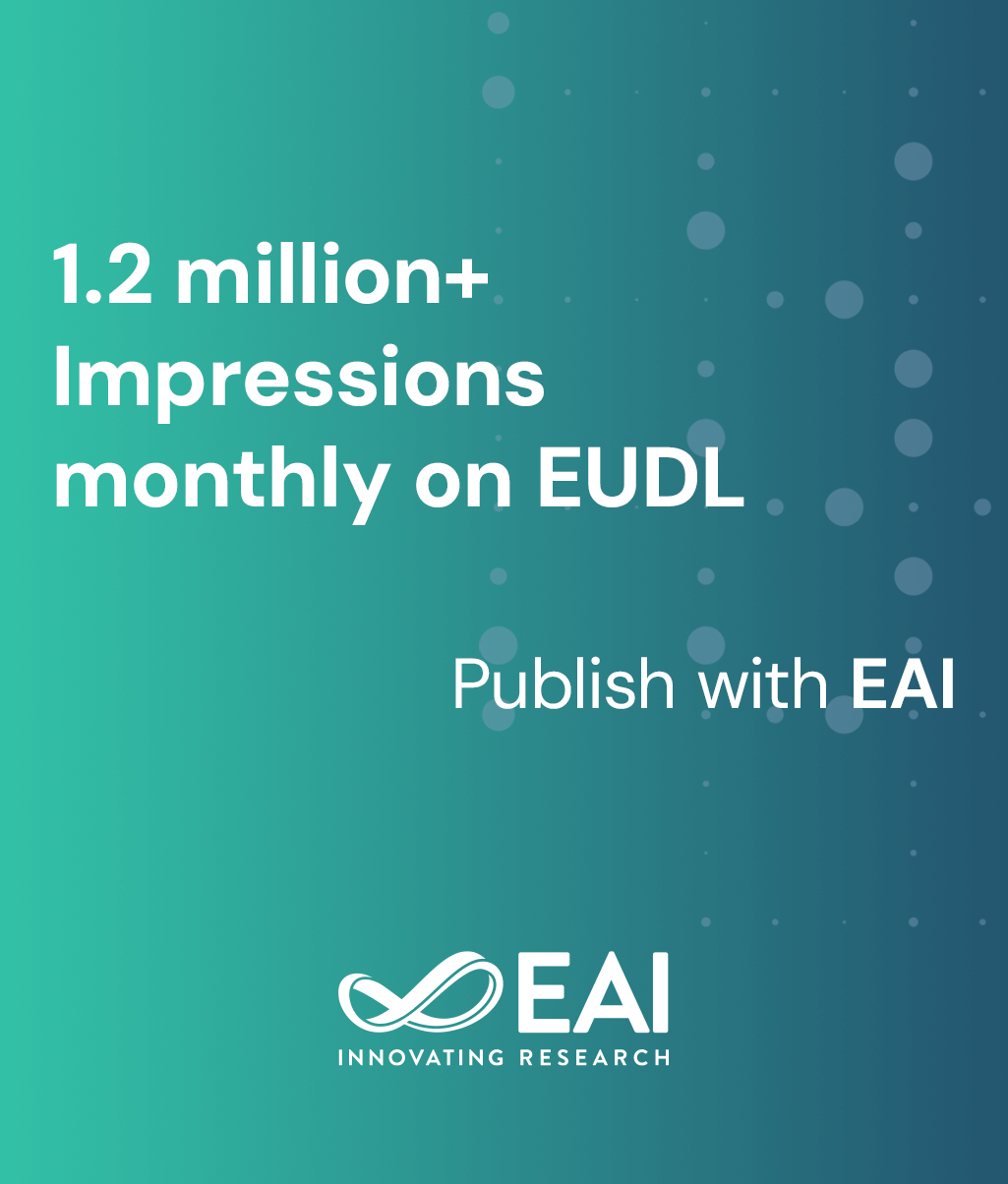
Research Article
ApEn-Based Epileptic EEG Classification Using Support Vector Machine
@INPROCEEDINGS{10.1007/978-3-319-73712-6_8, author={Hardika Gabani and Chirag Paunwala}, title={ApEn-Based Epileptic EEG Classification Using Support Vector Machine}, proceedings={Future Internet Technologies and Trends. First International Conference, ICFITT 2017, Surat, India, August 31 - September 2, 2017, Proceedings}, proceedings_a={ICFITT}, year={2018}, month={2}, keywords={ElectroEncephaloGram (EEG) signal Epilepsy seizure classification Approximate Entropy (ApEn) SVM (Support Vector Machine)}, doi={10.1007/978-3-319-73712-6_8} }
- Hardika Gabani
Chirag Paunwala
Year: 2018
ApEn-Based Epileptic EEG Classification Using Support Vector Machine
ICFITT
Springer
DOI: 10.1007/978-3-319-73712-6_8
Abstract
The ElectroEncephaloGram (EEG) signal plays an important role to identifying the disorder of epilepsy. Epilepsy is a neurological disorder which is an unexpected electrical disturbance of the brain. Due to which nerve cell activity in the brain becomes disrupted, causes people to have a “Seizure”. Now a day researchers are working and focusing on an automatic analysis of EEG signal to classify the Epilepsy. The EEG signal recording system generate very lengthy data. So, classification of epilepsy seizure requires a time-consuming process. This paper proposes SVM (Support Vector Machine) based automatic epilepsy seizure classification system that uses ApEn (Approximation Entropy). ApEn is reducing the patient data size without any loss of patient data so; we can easily classify the epilepsy seizure. ApEn is statistical parameters that measure the current amplitude value of an EEG signal based on its previous amplitude value. In this paper, we measure sensitivity, specificity, and accuracy using SVM classifier. The overall values as high as 100% can be achieved using the proposed system to differentiate epileptic state (Seizure class) out of normal state (Non-seizure Class) using time domain method.