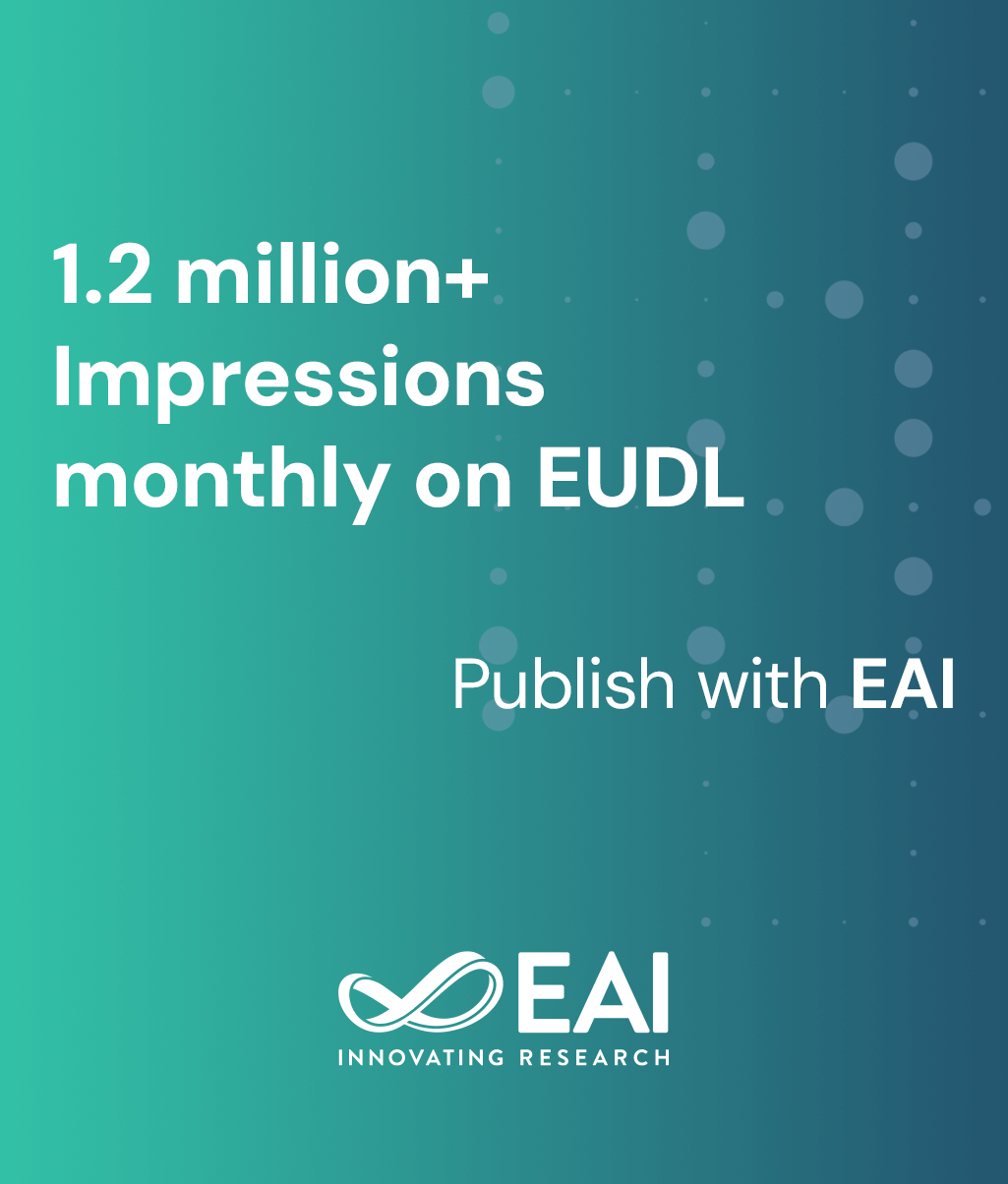
Research Article
Dimensionality Reduction Using PCA and SVD in Big Data: A Comparative Case Study
@INPROCEEDINGS{10.1007/978-3-319-73712-6_12, author={Sudeep Tanwar and Tilak Ramani and Sudhanshu Tyagi}, title={Dimensionality Reduction Using PCA and SVD in Big Data: A Comparative Case Study}, proceedings={Future Internet Technologies and Trends. First International Conference, ICFITT 2017, Surat, India, August 31 - September 2, 2017, Proceedings}, proceedings_a={ICFITT}, year={2018}, month={2}, keywords={Dimensionality reduction Principle component analysis Singular value decomposition Big data}, doi={10.1007/978-3-319-73712-6_12} }
- Sudeep Tanwar
Tilak Ramani
Sudhanshu Tyagi
Year: 2018
Dimensionality Reduction Using PCA and SVD in Big Data: A Comparative Case Study
ICFITT
Springer
DOI: 10.1007/978-3-319-73712-6_12
Abstract
With the advancement in technology, data produced from different sources such as Internet, health care, financial companies, social media, etc. are increases continuously at a rapid rate. Potential growth of this data in terms of volume, variety and velocity coined a new emerging area of research, Big Data (BD). Continuous storage, processing, monitoring (if required), real time analysis are few current challenges of BD. However, these challenges becomes more critical when data can be uncertain, inconsistent and redundant. Hence, to reduce the overall processing time dimensionality reduction (DR) is one of the efficient techniques. Therefore, keeping in view of the above, in this paper, we have used principle component analysis (PCA) and singular value decomposition (SVD) techniques to perform DR over BD. We have compared the performance of both techniques in terms of accuracy and mean square error (MSR). Comparative results shows that for numerical reasons SVD is preferred PCA. Whereas, using PCA to train the data in dimension reduction for an image gives good classification output.