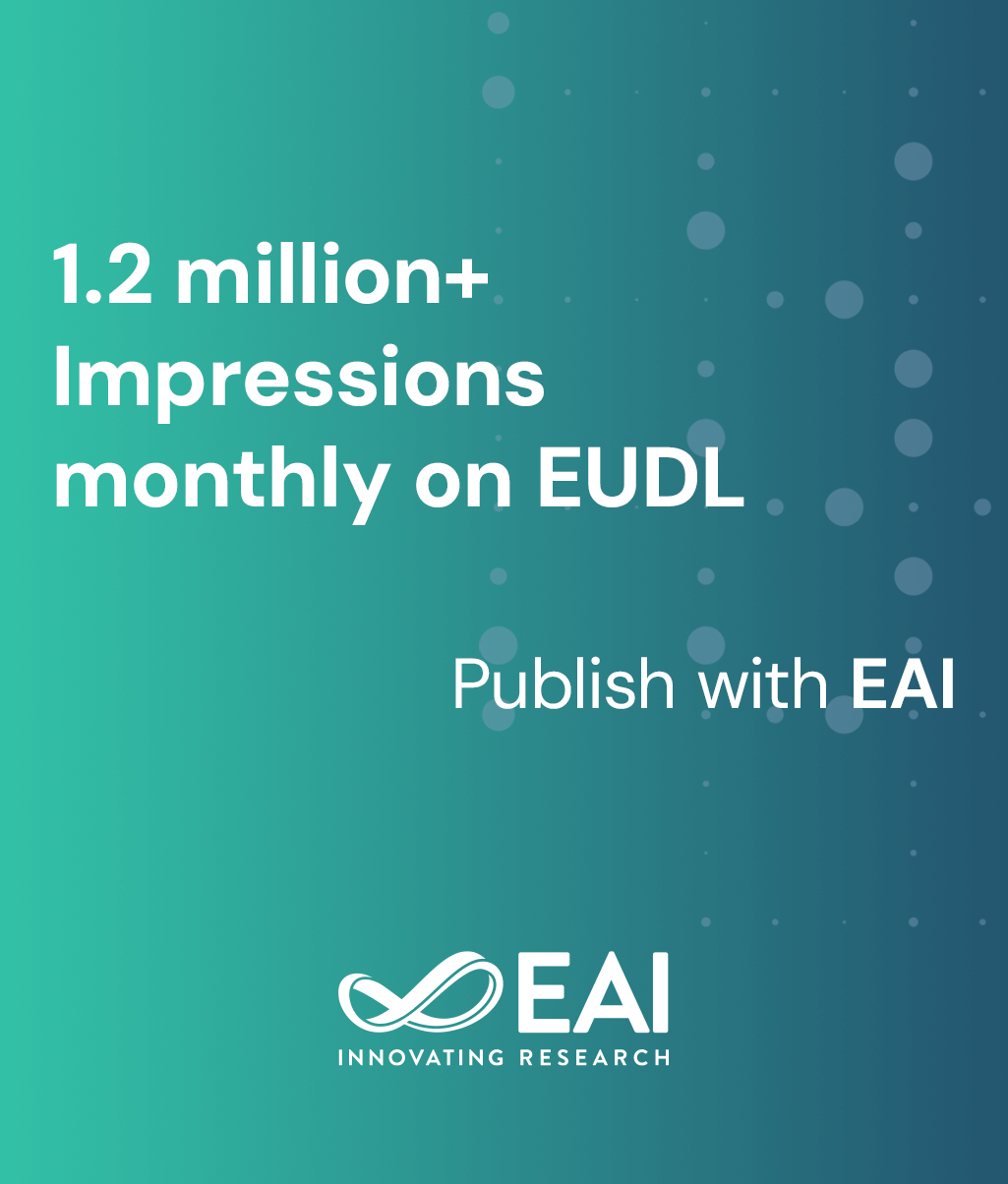
Research Article
A Visualization Scheme for Network Forensics Based on Attribute Oriented Induction Based Frequent Item Mining and Hyper Graph
@INPROCEEDINGS{10.1007/978-3-319-73697-6_10, author={Jianguo Jiang and Jiuming Chen and Kim-Kwang Choo and Chao Liu and Kunying Liu and Min Yu}, title={A Visualization Scheme for Network Forensics Based on Attribute Oriented Induction Based Frequent Item Mining and Hyper Graph}, proceedings={Digital Forensics and Cyber Crime. 9th International Conference, ICDF2C 2017, Prague, Czech Republic, October 9-11, 2017, Proceedings}, proceedings_a={ICDF2C}, year={2018}, month={1}, keywords={Visualization Big data analysis Network forensic Hypergraph}, doi={10.1007/978-3-319-73697-6_10} }
- Jianguo Jiang
Jiuming Chen
Kim-Kwang Choo
Chao Liu
Kunying Liu
Min Yu
Year: 2018
A Visualization Scheme for Network Forensics Based on Attribute Oriented Induction Based Frequent Item Mining and Hyper Graph
ICDF2C
Springer
DOI: 10.1007/978-3-319-73697-6_10
Abstract
Visualizing massive network traffic flows or security logs can facilitate network forensics, such as in the detection of anomalies. However, existing visualization methods do not generally scale well, or are not suited for dealing with large datasets. Thus, in this paper, we propose a visualization scheme, where an attribute-oriented induction-based frequent-item mining algorithm (AOI-FIM) is used to extract attack patterns hidden in a large dataset. Also, we leverage the hypergraph to display multi-attribute associations of the extracted patterns. An interaction module designed to facilitate forensics analyst in fetching event information from the database and identifying unknown attack patterns is also presented. We then demonstrate the utility of our approach (i.e. using both frequent item mining and hypergraphs to deal with visualization problems in network forensics).