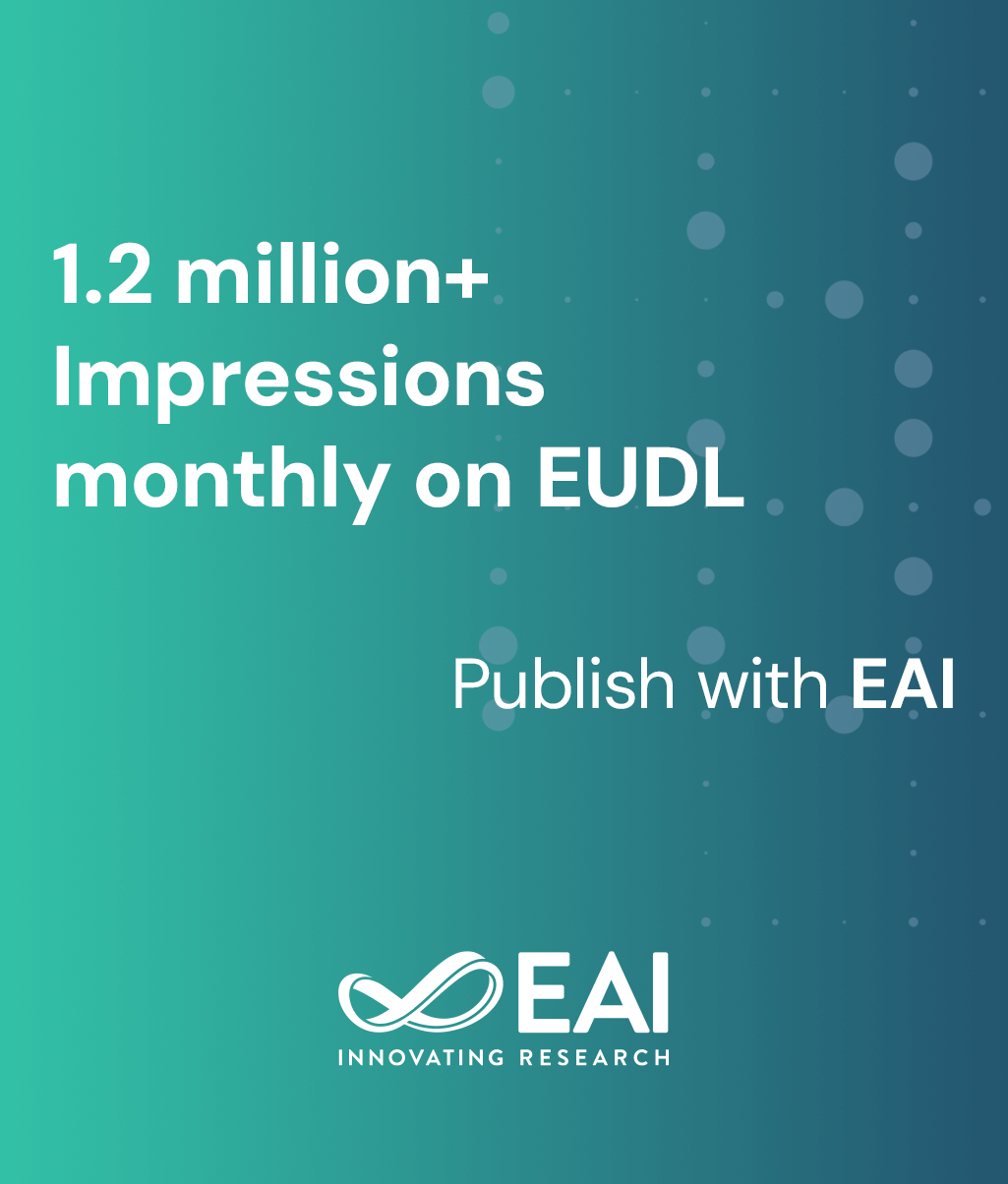
Research Article
Efficient Data Gathering with Compressed Sensing Multiuser Detection in Underwater Wireless Sensor Networks
@INPROCEEDINGS{10.1007/978-3-319-73564-1_62, author={Rui Du and Wenjing Kang and Bo Li and Gongliang Liu}, title={Efficient Data Gathering with Compressed Sensing Multiuser Detection in Underwater Wireless Sensor Networks}, proceedings={Machine Learning and Intelligent Communications. Second International Conference, MLICOM 2017, Weihai, China, August 5-6, 2017, Proceedings, Part I}, proceedings_a={MLICOM}, year={2018}, month={2}, keywords={Compressed sensing Data gathering Multiuser detection Underwater wireless sensor networks}, doi={10.1007/978-3-319-73564-1_62} }
- Rui Du
Wenjing Kang
Bo Li
Gongliang Liu
Year: 2018
Efficient Data Gathering with Compressed Sensing Multiuser Detection in Underwater Wireless Sensor Networks
MLICOM
Springer
DOI: 10.1007/978-3-319-73564-1_62
Abstract
Bandwidth and energy constraints of underwater wireless sensors networks necessitate an intelligent data transmission between sensor nodes and the fusion center. This paper considers a data gathering underwater networks for monitoring oceanic environmental elements (e.g. temperature, salinity) and only a portion of measurements from sensors allows for oceanic information map reconstruction under compressed sensing (CS) theory. By utilizing the spatial sparsity of active sensors’ data, we introduce an activity and data detection based on CS at the receiver side, which results in an efficient data communication by avoiding the necessity of conveying identity information. For an interleave division multiple access (IDMA) sporadic transmission, CS-CBC detection that combines the benefits from chip-by-chip (CBC) multi-user detection and CS detection is proposed. Further, by successively exploring the sparsity of sensor data in spatial and frequency domain, we propose a novel efficient data gathering scheme named Dual-domain compressed sensing (DCS). Simulation results validate the effectiveness of the proposed scheme and an optimal sensing probability problem related to minimum reconstruction error is explored.