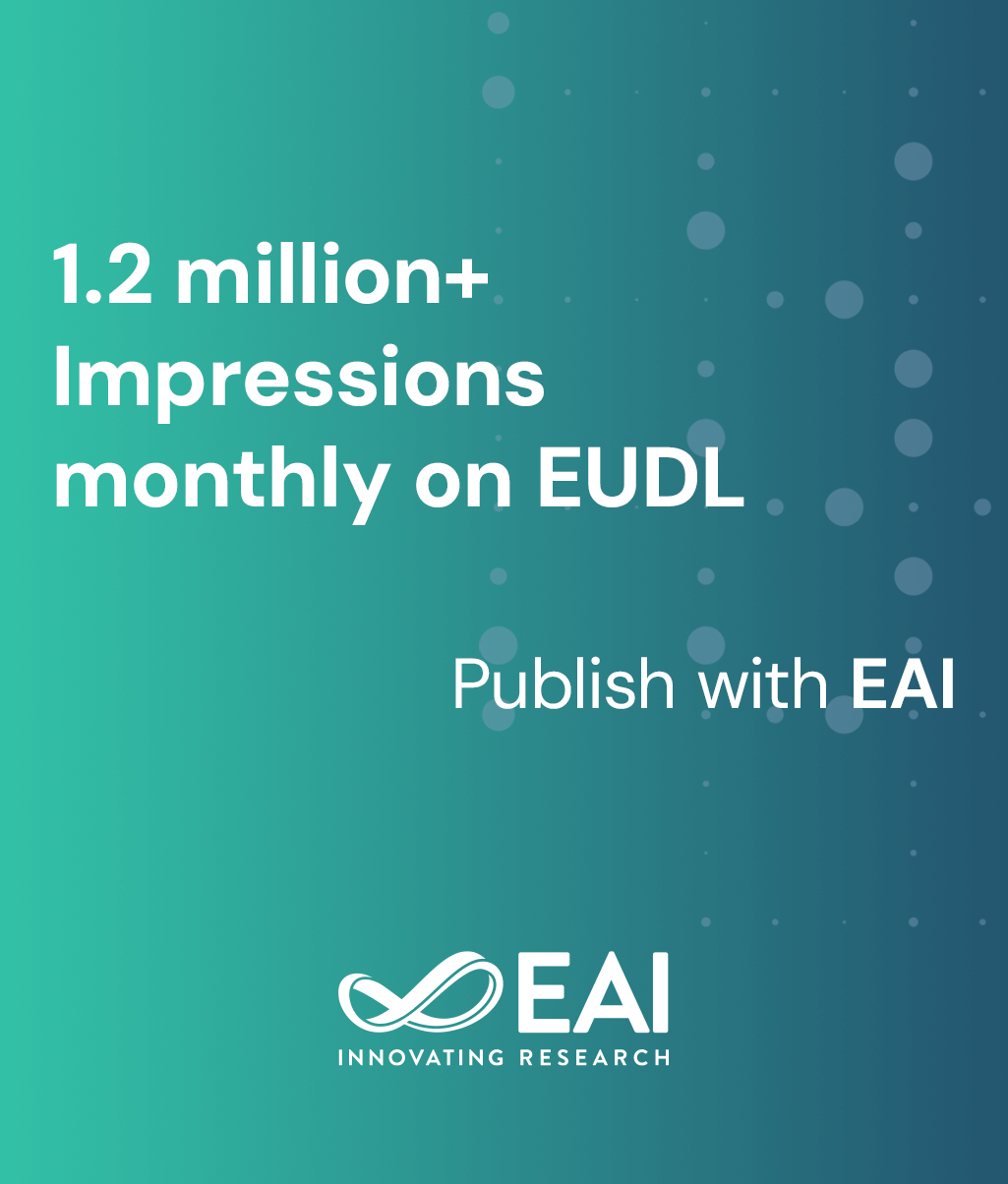
Research Article
Artificial-Neural-Network-Based Automatic Modulation Recognition in Satellite Communication
@INPROCEEDINGS{10.1007/978-3-319-73564-1_39, author={Yumeng Zhang and Mingchuan Yang and Xiaofeng Liu}, title={Artificial-Neural-Network-Based Automatic Modulation Recognition in Satellite Communication}, proceedings={Machine Learning and Intelligent Communications. Second International Conference, MLICOM 2017, Weihai, China, August 5-6, 2017, Proceedings, Part I}, proceedings_a={MLICOM}, year={2018}, month={2}, keywords={Modulation recognition Artificial neural network Satellite communication}, doi={10.1007/978-3-319-73564-1_39} }
- Yumeng Zhang
Mingchuan Yang
Xiaofeng Liu
Year: 2018
Artificial-Neural-Network-Based Automatic Modulation Recognition in Satellite Communication
MLICOM
Springer
DOI: 10.1007/978-3-319-73564-1_39
Abstract
In order to improve the correct recognition rate of signals transmitted in satellite communication system, three different structures of artificial neural network (ANN), including feed forward network (FFN), cascade forward network (CFN) and competitive neural network (CNN) are investigated in this paper. Then their performance of correct recognition rate and performance of convergence rate are compared. Results of simulation indicate that typical FFN’s performance dramatically deteriorates in the case of Rician fading, CFN’s performance is similar to the former one while it has higher convergence rate. CNN’s performance of correct recognition rate is the best among these three nets, but in the training process, its performance of convergence rate is not good.