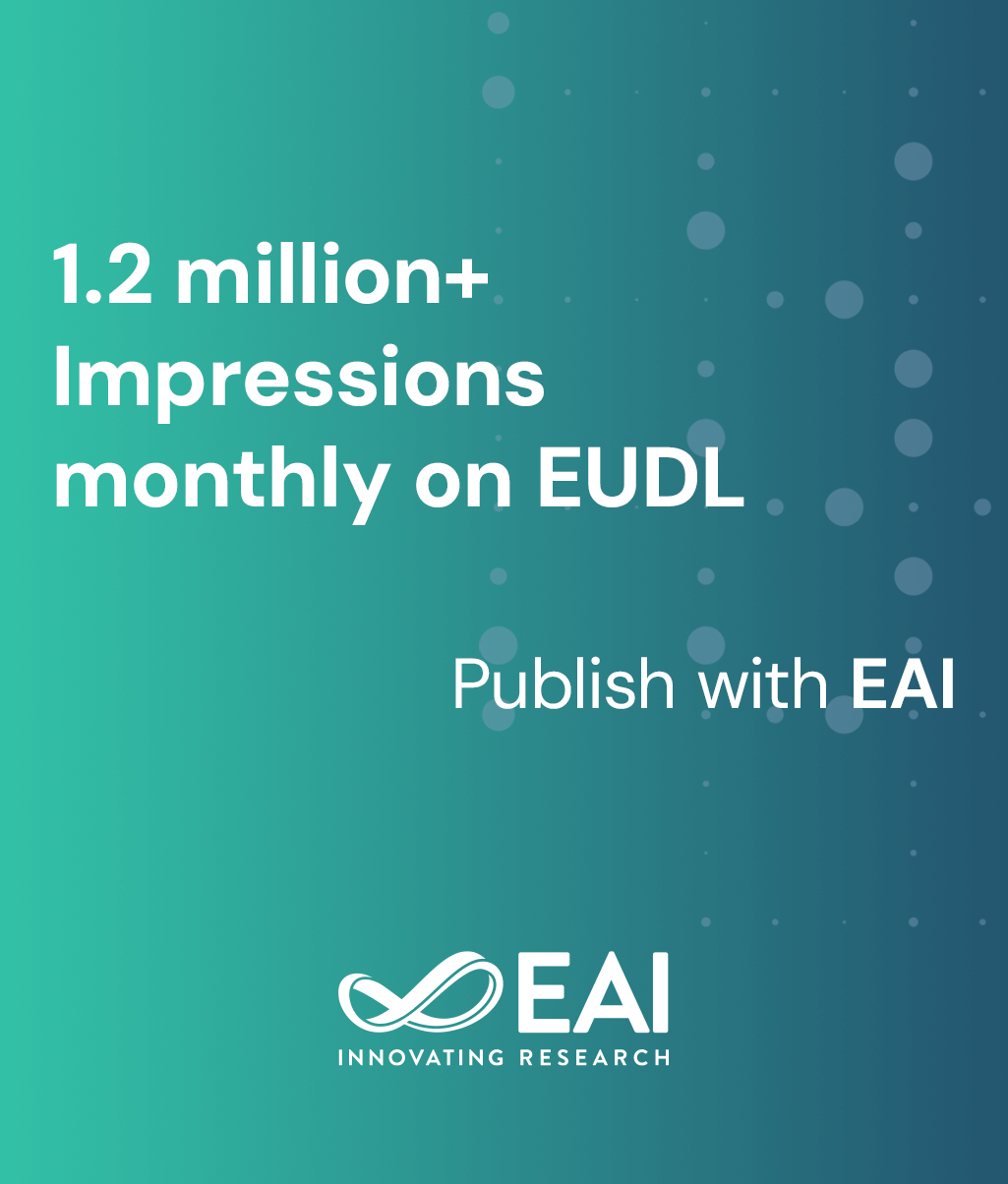
Research Article
Defect Detection of Photovoltaic Modules Based on Convolutional Neural Network
743 downloads
@INPROCEEDINGS{10.1007/978-3-319-73564-1_13, author={Mingjian Sun and Shengmiao Lv and Xue Zhao and Ruya Li and Wenhan Zhang and Xiao Zhang}, title={Defect Detection of Photovoltaic Modules Based on Convolutional Neural Network}, proceedings={Machine Learning and Intelligent Communications. Second International Conference, MLICOM 2017, Weihai, China, August 5-6, 2017, Proceedings, Part I}, proceedings_a={MLICOM}, year={2018}, month={2}, keywords={Convolutional Neural Network PV module cracks Defect detection Deep learning}, doi={10.1007/978-3-319-73564-1_13} }
- Mingjian Sun
Shengmiao Lv
Xue Zhao
Ruya Li
Wenhan Zhang
Xiao Zhang
Year: 2018
Defect Detection of Photovoltaic Modules Based on Convolutional Neural Network
MLICOM
Springer
DOI: 10.1007/978-3-319-73564-1_13
Abstract
Deep learning is employed to detect defects in photovoltaic (PV) modules in the thesis. Firstly, the thesis introduces related concepts of cracks. Then a convolutional neural network with seven layers is constructed to classify the defective battery panels. Finally, the accuracy of the validation set is 98.35%. Besides, the thesis introduces a method in which a single battery cell can be extracted from the Electro Luminescence (EL) image of the PV module. This method is very suitable for automatic inspection of photovoltaic power plants.
Copyright © 2017–2025 EAI