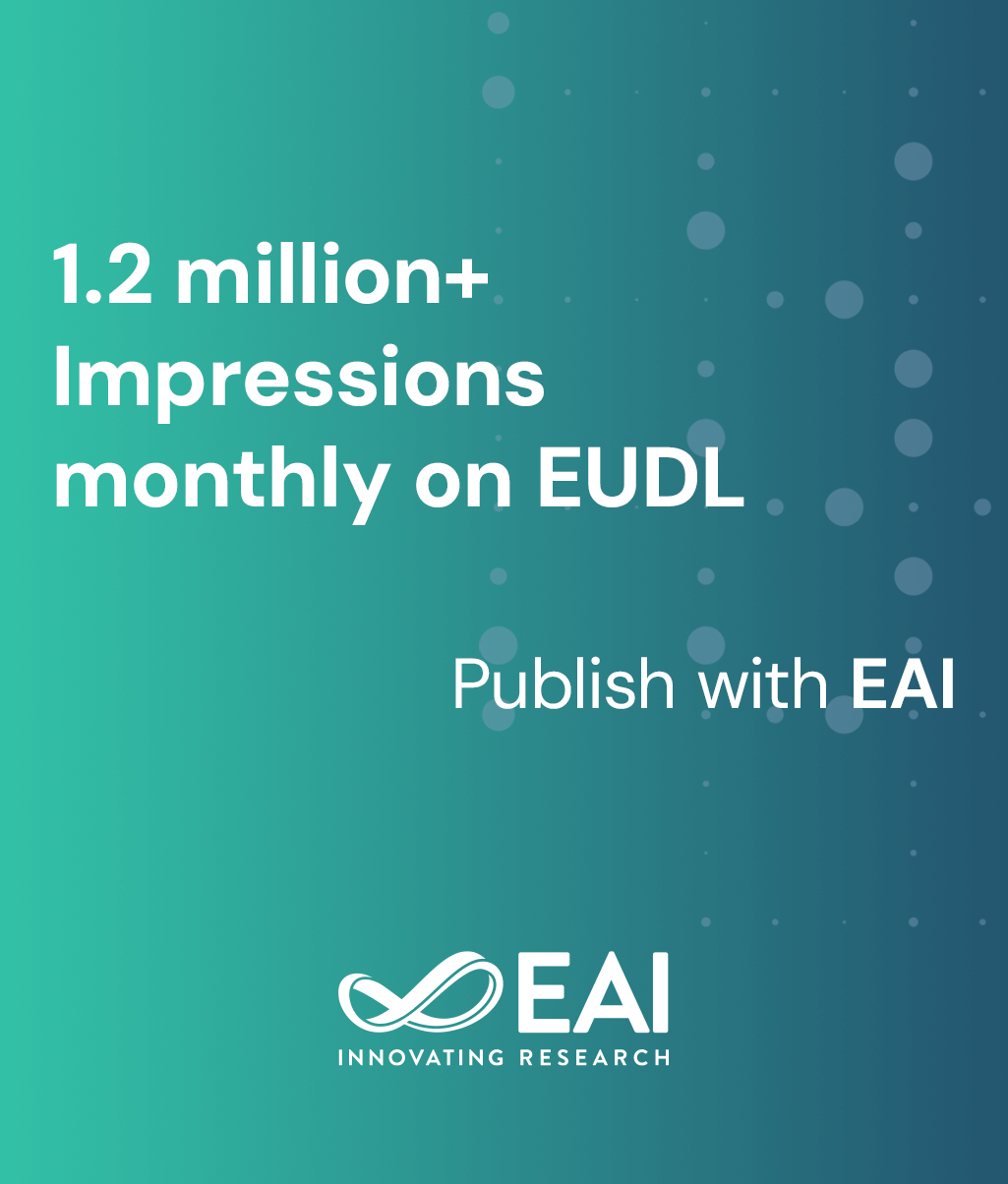
Research Article
Abnormal Traffic Flow Detection Based on Dynamic Hybrid Strategy
@INPROCEEDINGS{10.1007/978-3-319-73317-3_55, author={Yang Liu and Hongping Xu and Hang Yi and Xiaotao Yan and Jian Kang and Weiqiang Xia and Qingping Shi and Chaopeng Shen}, title={Abnormal Traffic Flow Detection Based on Dynamic Hybrid Strategy}, proceedings={Advanced Hybrid Information Processing. First International Conference, ADHIP 2017, Harbin, China, July 17--18, 2017, Proceedings}, proceedings_a={ADHIP}, year={2018}, month={2}, keywords={Port mapping Payload feature matching Dynamic hybrid strategy Machine learning}, doi={10.1007/978-3-319-73317-3_55} }
- Yang Liu
Hongping Xu
Hang Yi
Xiaotao Yan
Jian Kang
Weiqiang Xia
Qingping Shi
Chaopeng Shen
Year: 2018
Abnormal Traffic Flow Detection Based on Dynamic Hybrid Strategy
ADHIP
Springer
DOI: 10.1007/978-3-319-73317-3_55
Abstract
Efficient and accurate analysis of the traffic data contained in the network is the key measure to detect the abnormal behavior, resist the invasion and protect the information security. In this paper, we make a comprehensive utilization of the characteristics of port mapping identification, payload identification, statistical analysis and SVM machine learning, and propose the dynamic hybrid strategy. Firstly, the machine learning training samples are obtained through port mapping and load feature recognition. Then, on the basis of information gain feature selection, the SVM machine learning model is built and trained. Finally, through the voting mechanism, we achieve comprehensive analysis of the traffic data. The experimental results show that the accuracy of the proposed algorithm is as high as 99.1%, and the number of manual decision analysis is greatly reduced at the same time.