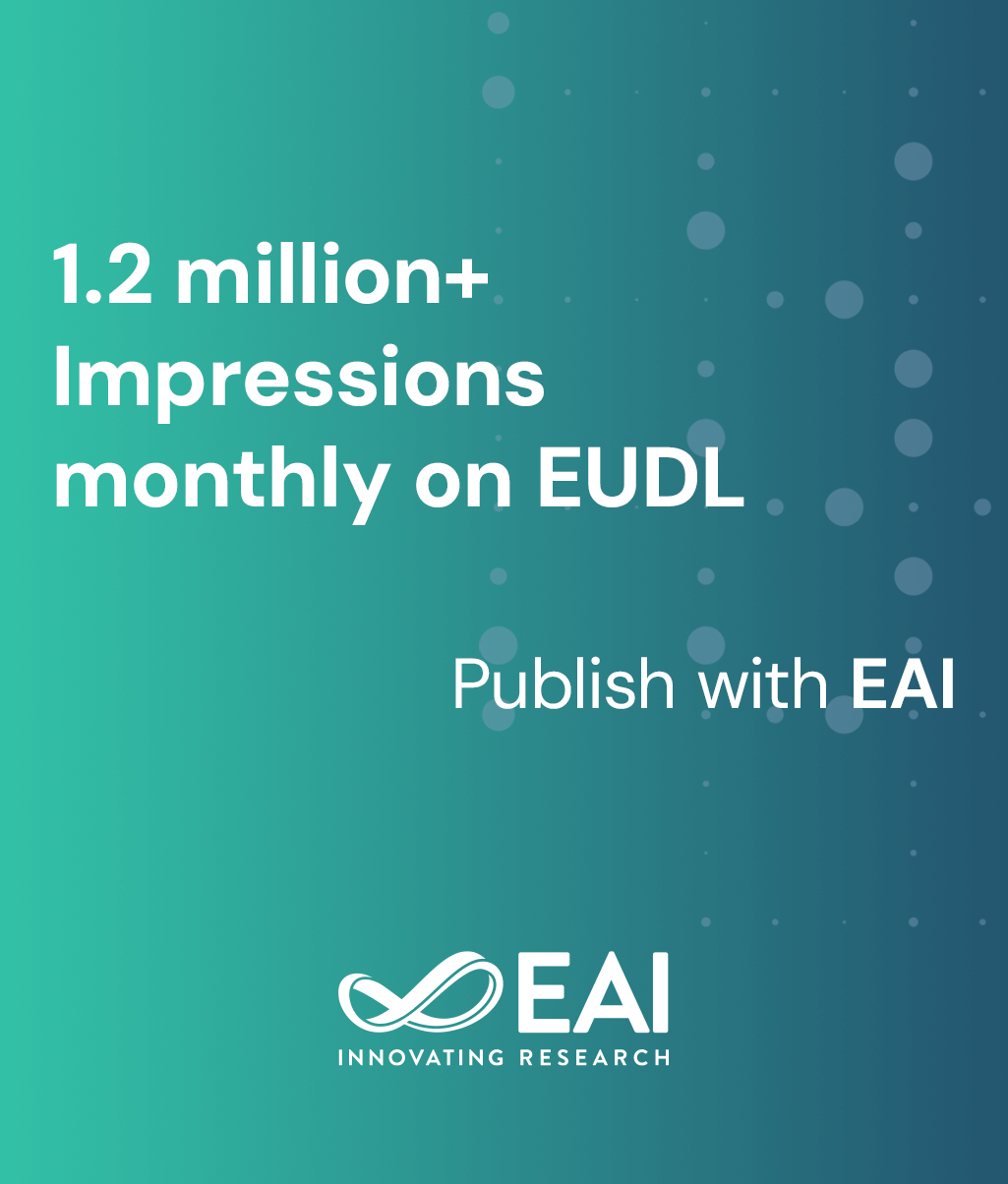
Research Article
An Artificial Neural Network Approach to Student Study Failure Risk Early Warning Prediction Based on TensorFlow
@INPROCEEDINGS{10.1007/978-3-319-73317-3_38, author={Mi Chunqiao and Peng Xiaoning and Deng Qingyou}, title={An Artificial Neural Network Approach to Student Study Failure Risk Early Warning Prediction Based on TensorFlow}, proceedings={Advanced Hybrid Information Processing. First International Conference, ADHIP 2017, Harbin, China, July 17--18, 2017, Proceedings}, proceedings_a={ADHIP}, year={2018}, month={2}, keywords={Artificial neural network Study failure risk Early warning prediction TensorFlow}, doi={10.1007/978-3-319-73317-3_38} }
- Mi Chunqiao
Peng Xiaoning
Deng Qingyou
Year: 2018
An Artificial Neural Network Approach to Student Study Failure Risk Early Warning Prediction Based on TensorFlow
ADHIP
Springer
DOI: 10.1007/978-3-319-73317-3_38
Abstract
Higher education is now facing big challenges about low course study completion and graduation degree completion rate, for which student study failure in course is the main reason. However, the failure in course study is a comprehensive result of various factors and is characterized by uncertainty. Artificial neural network approach is advantageous for dealing with this issue, and in this study we provided such an approach to predicting student study failure risk for early warning in course study based on the TensorFlow platform. In our model, for each student, four input variables: (1) times of login onto the online study system; (2) times of downloading study resource; (3) attendance earned points; and (4) assignment earned points, and one target variable: the final course grade point were collected for network training. At last, by validating with the observed data, consistency is shown between our predicted results and the actual observed data, which indicates that the employed model is a promising approach for identifying at-risk student. It is helpful for educators to timely apply corresponding strategic pedagogical interventions to help at-risk students avoid academic failure, and to effectively improve early warning education management.