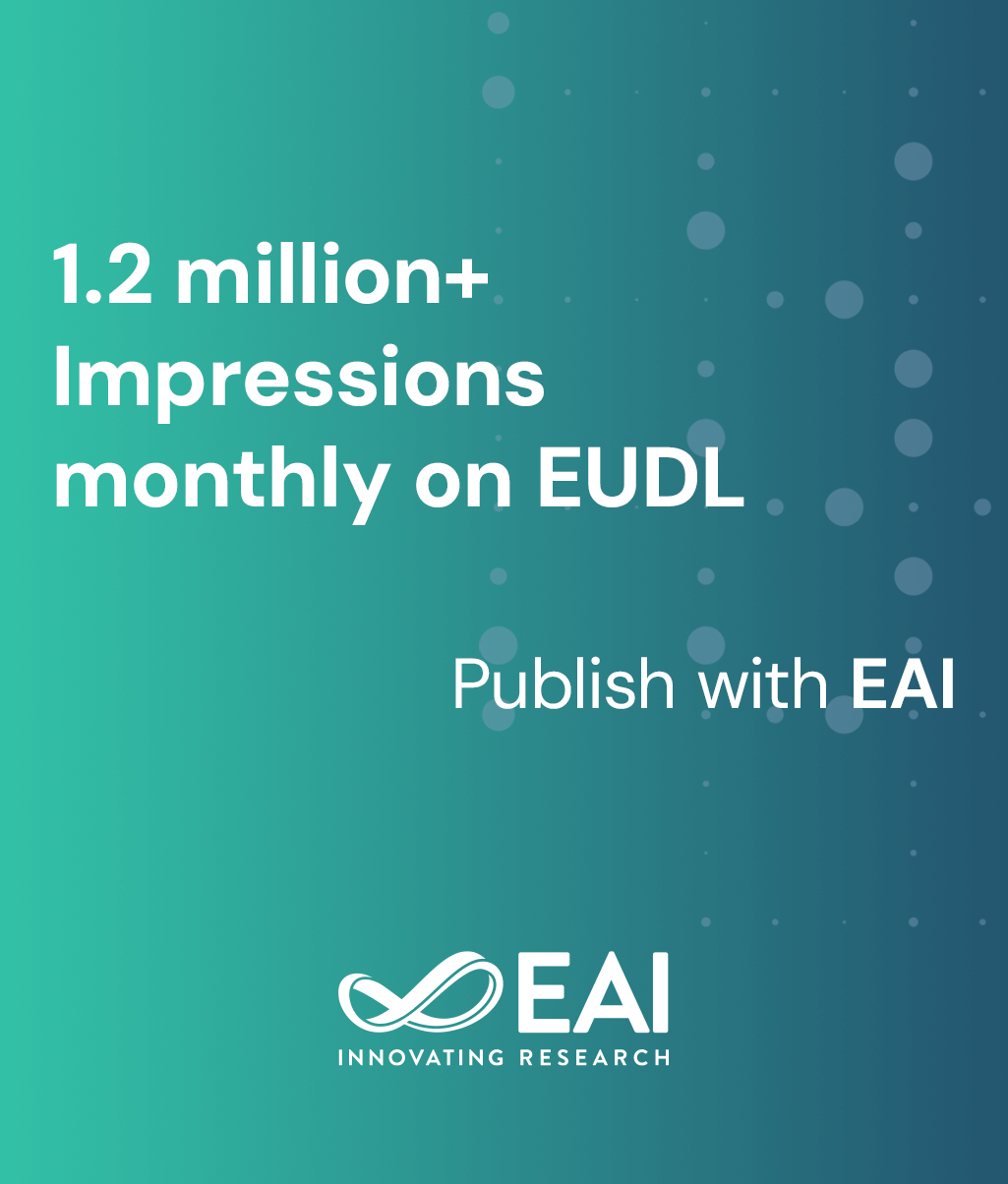
Research Article
DLRRS: A New Recommendation System Based on Double Linear Regression Models
@INPROCEEDINGS{10.1007/978-3-319-73317-3_29, author={Chenglong Li and Zhaoguo Wang and Shoufeng Cao and Longtao He}, title={DLRRS: A New Recommendation System Based on Double Linear Regression Models}, proceedings={Advanced Hybrid Information Processing. First International Conference, ADHIP 2017, Harbin, China, July 17--18, 2017, Proceedings}, proceedings_a={ADHIP}, year={2018}, month={2}, keywords={Recommendation system Linear regression RMSE}, doi={10.1007/978-3-319-73317-3_29} }
- Chenglong Li
Zhaoguo Wang
Shoufeng Cao
Longtao He
Year: 2018
DLRRS: A New Recommendation System Based on Double Linear Regression Models
ADHIP
Springer
DOI: 10.1007/978-3-319-73317-3_29
Abstract
Recently, it is difficulty for ordinary users to find their own points of interest when facing of massive information accompanied by the popularity and development of social networks. Recommendation system is considered to be the most potential way to solve the problem by profiling personalized interest model and initiatively pushing potential interesting contents to each user. However, collaborative filtering, one of the most mature and extensively applied recommender methods currently, is facing problems of data sparsity and diversity and so on, causing its effect unsatisfactory. In the article, we put forward DLRRS, a new recommendation system depending on double linear regression models. Compared with the traditional methods, such as item average scores, collaborative filtering, and rating frequency, DLRRS has the best predictive RMSE accuracy and less fluctuation. DLRRS also has high real-time performance, which makes the system complete all the calculations in the time of Ω(n).