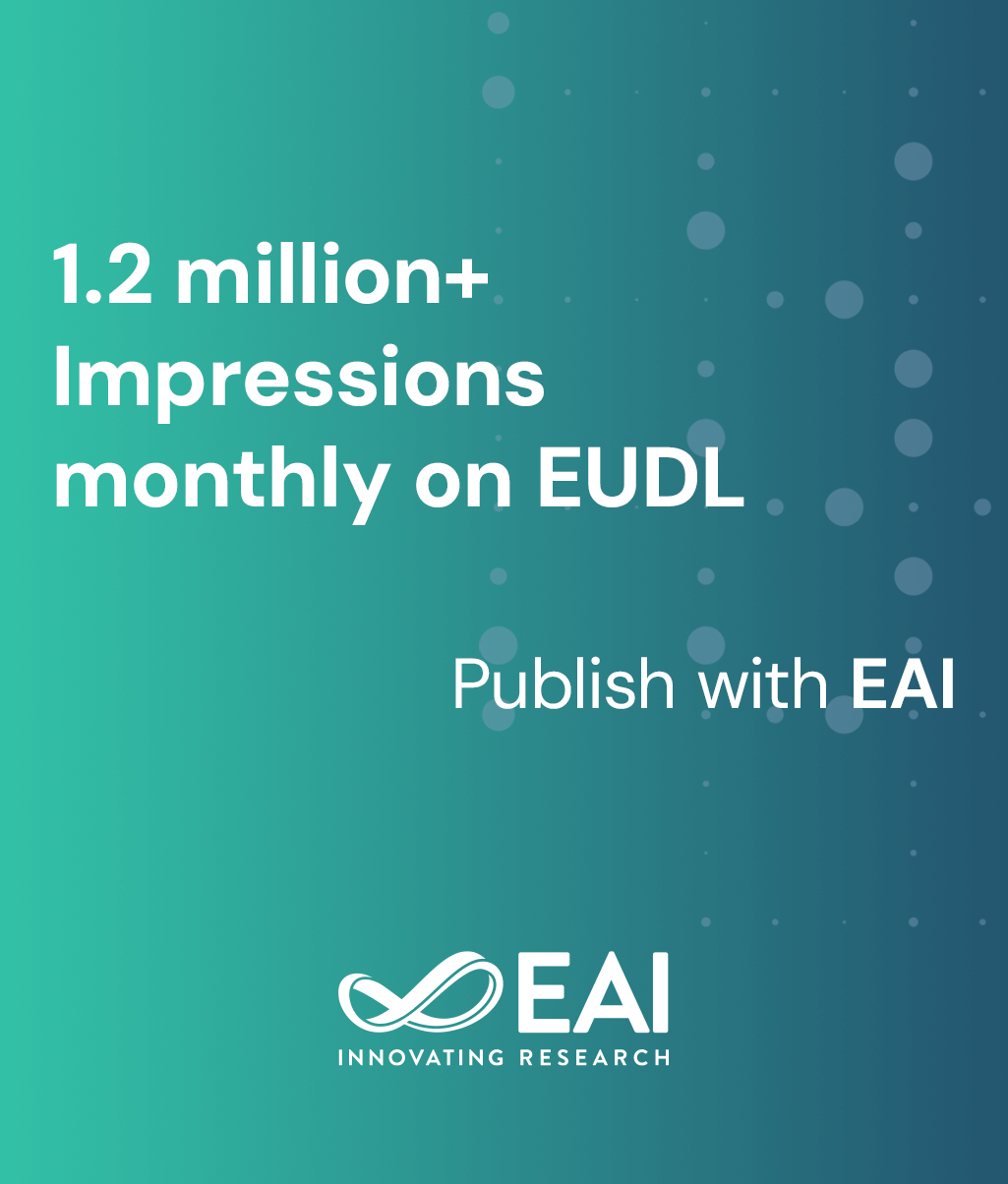
Research Article
Learning the Structure of Dynamic Bayesian Network with Hybrid Data and Domain Knowledges
@INPROCEEDINGS{10.1007/978-3-319-73317-3_28, author={Haiyang Jia and Juan Chen and Zhiming Song}, title={Learning the Structure of Dynamic Bayesian Network with Hybrid Data and Domain Knowledges}, proceedings={Advanced Hybrid Information Processing. First International Conference, ADHIP 2017, Harbin, China, July 17--18, 2017, Proceedings}, proceedings_a={ADHIP}, year={2018}, month={2}, keywords={Machine learning Dynamic system Bayesian network Domain knowledge}, doi={10.1007/978-3-319-73317-3_28} }
- Haiyang Jia
Juan Chen
Zhiming Song
Year: 2018
Learning the Structure of Dynamic Bayesian Network with Hybrid Data and Domain Knowledges
ADHIP
Springer
DOI: 10.1007/978-3-319-73317-3_28
Abstract
Dynamic Bayesian Networks (DBNs) is a powerful graphical model for representing temporal stochastic processes. Learning the structure of DBNs is the fundamental step for parameter learning, inference, application etc. In some cases, such as computational systems biology, learning the structure of DBNs facing the two challenges (1) experimental settings only capture few time series and steady state measurements. (2) the knowledge about DBNs is uncertainty, rare and even with conflict. The paper considers the time series data, steady state and domain knowledge simultaneously, presents a novel algorithm for learning the structure of DBNs. Compare with single source learning, empirical experiment shows that learning with hybrid data and domain knowledges improved the accuracy and effectiveness of the DBNs structure learning.