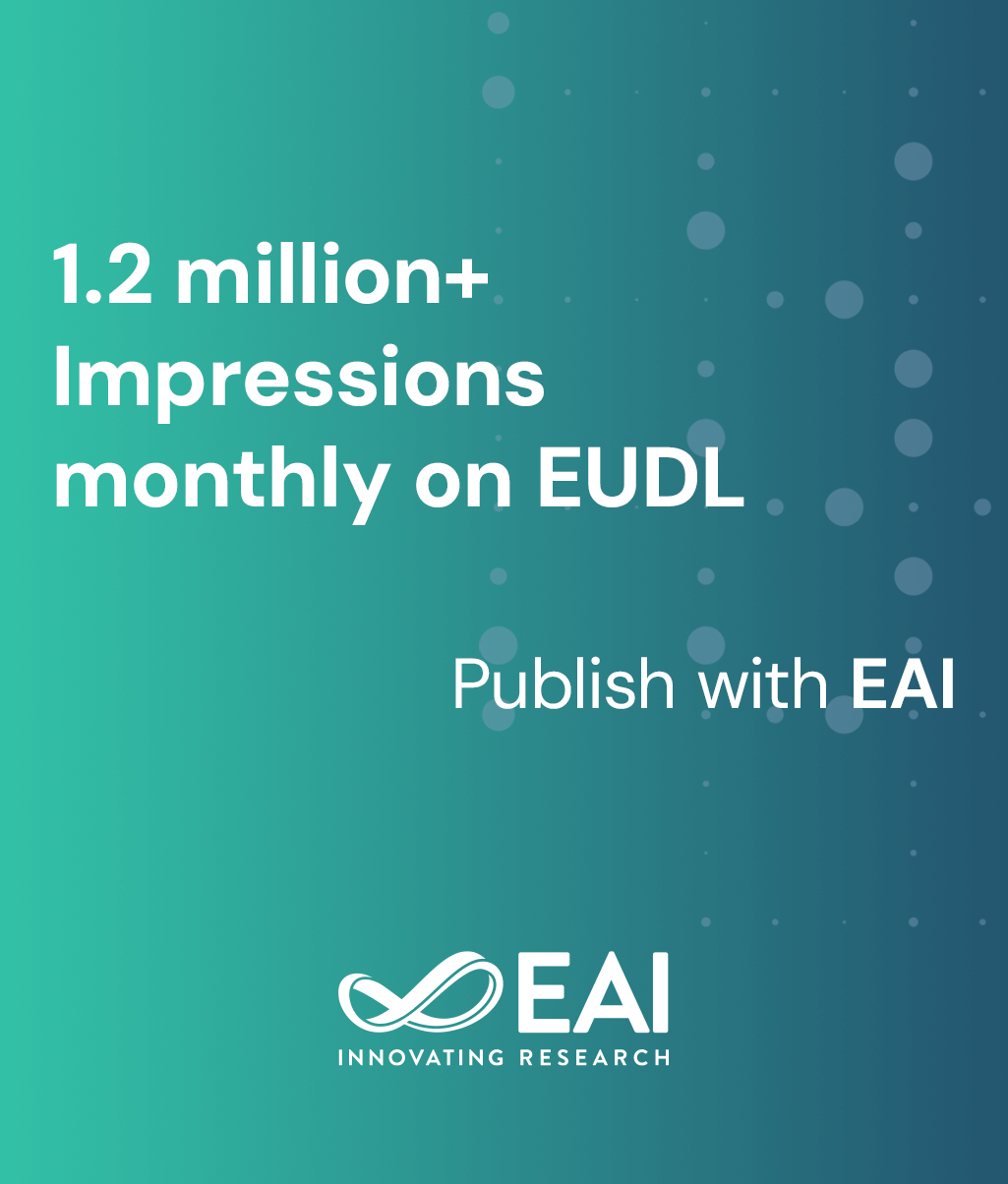
Research Article
Neural Networks for Biomedical Signals Classification Based on Empirical Mode Decomposition and Principal Component Analysis
478 downloads
@INPROCEEDINGS{10.1007/978-3-319-72965-7_25, author={Abdoul Abdou and Ndeye Ngom and Samba Sidib\^{e} and Oumar Niang and Abdoulaye Thioune and Cheikh Ndiaye}, title={Neural Networks for Biomedical Signals Classification Based on Empirical Mode Decomposition and Principal Component Analysis}, proceedings={Innovation and Interdisciplinary Solutions for Underserved Areas. First International Conference, InterSol 2017 and Sixth Collogue National sur la Recherche en Informatique et ses Applications, CNRIA 2017, Dakar, Senegal, April 11--12, 2017, Proceedings}, proceedings_a={INTERSOL \& CNRIA}, year={2018}, month={2}, keywords={Empirical mode decomposition Neural network ECG signal classification}, doi={10.1007/978-3-319-72965-7_25} }
- Abdoul Abdou
Ndeye Ngom
Samba Sidibé
Oumar Niang
Abdoulaye Thioune
Cheikh Ndiaye
Year: 2018
Neural Networks for Biomedical Signals Classification Based on Empirical Mode Decomposition and Principal Component Analysis
INTERSOL & CNRIA
Springer
DOI: 10.1007/978-3-319-72965-7_25
Abstract
The three main events presented in the electrocardiogram (ECG) signal of each heartbeat are: the wave, the QRS complex and the T wave. Each event contains its own peak, making this important to analyze their morphology, amplitude and duration for cardiac abnormalities. In this study, we propose a system for biomedical signal analysis based on empirical mode decomposition. Mustispectral analysis is first performed to remove noise, detect QRS complex and compute the QRS wide. Then statistical features and QRS wide are after used as inputs of classifier based on neural network model. The proposed methodology is tested on real biomedical data and discussed.
Copyright © 2017–2025 ICST