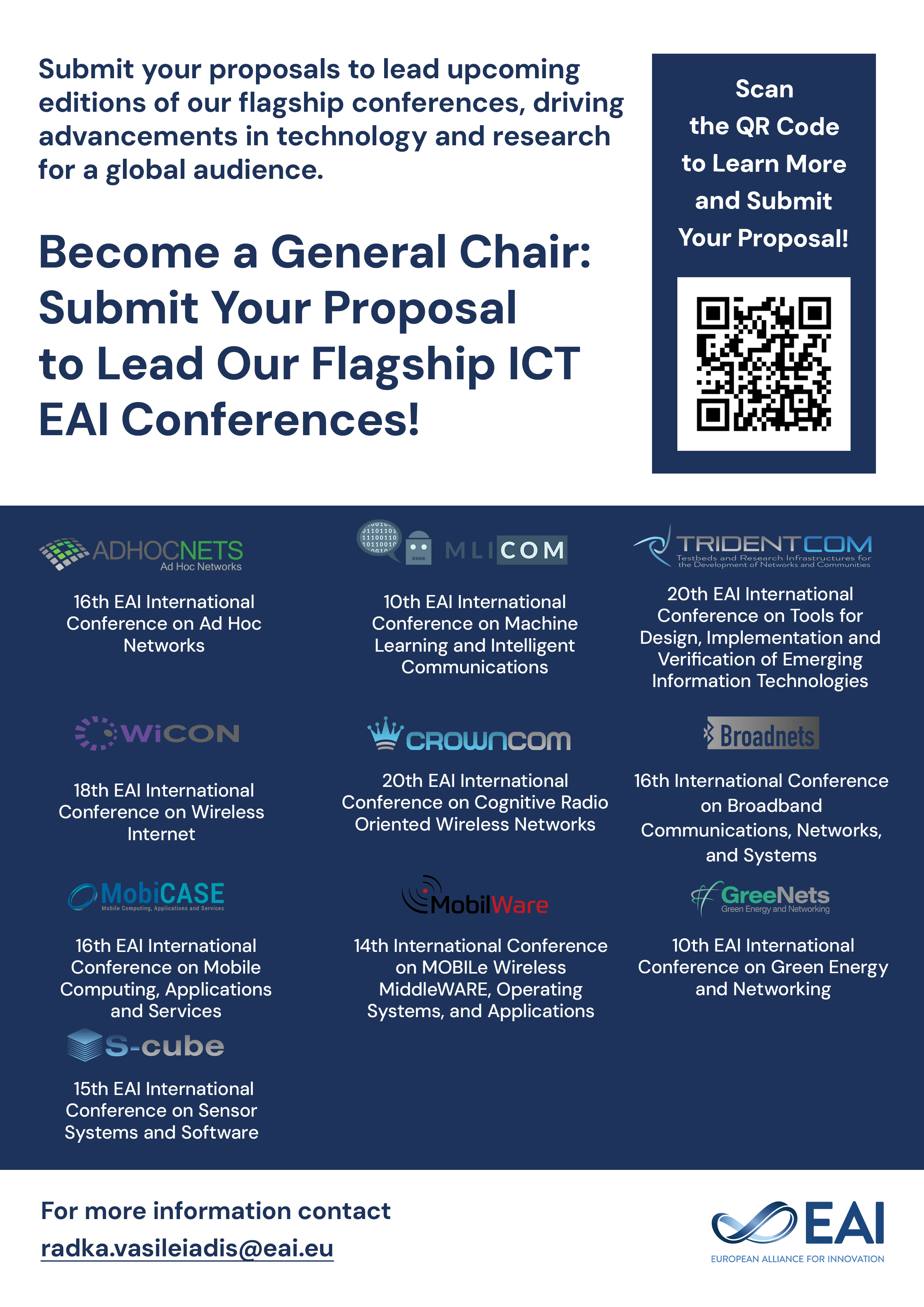
Research Article
Intrusion Detection Using Unsupervised Approach
304 downloads
@INPROCEEDINGS{10.1007/978-3-319-67837-5_18, author={Jai Singh and Nizar Bouguila}, title={Intrusion Detection Using Unsupervised Approach}, proceedings={Emerging Technologies for Developing Countries. First International EAI Conference, AFRICATEK 2017, Marrakech, Morocco, March 27-28, 2017 Proceedings}, proceedings_a={AFRICATEK}, year={2017}, month={10}, keywords={Mixture models Intrusion Aitchison distance Feature selection}, doi={10.1007/978-3-319-67837-5_18} }
- Jai Singh
Nizar Bouguila
Year: 2017
Intrusion Detection Using Unsupervised Approach
AFRICATEK
Springer
DOI: 10.1007/978-3-319-67837-5_18
Abstract
The process of detecting intrusion on network traffic has always remained a key concern for security researchers. During the previous years, intrusion detection had attracted many researchers to find anomaly on NSL-KDD data set. Hence, most of the approaches applied on NSL KDD data set were supervised approaches. We had conducted statistical analysis on this data set using Dirichlet Mixture model. We have seen initialization using Aitchison distance fits better for proportional data. The feature selection highly affects both the performance and results into an improved evaluation of anomaly detection by an unsupervised approach.
Copyright © 2017–2024 ICST