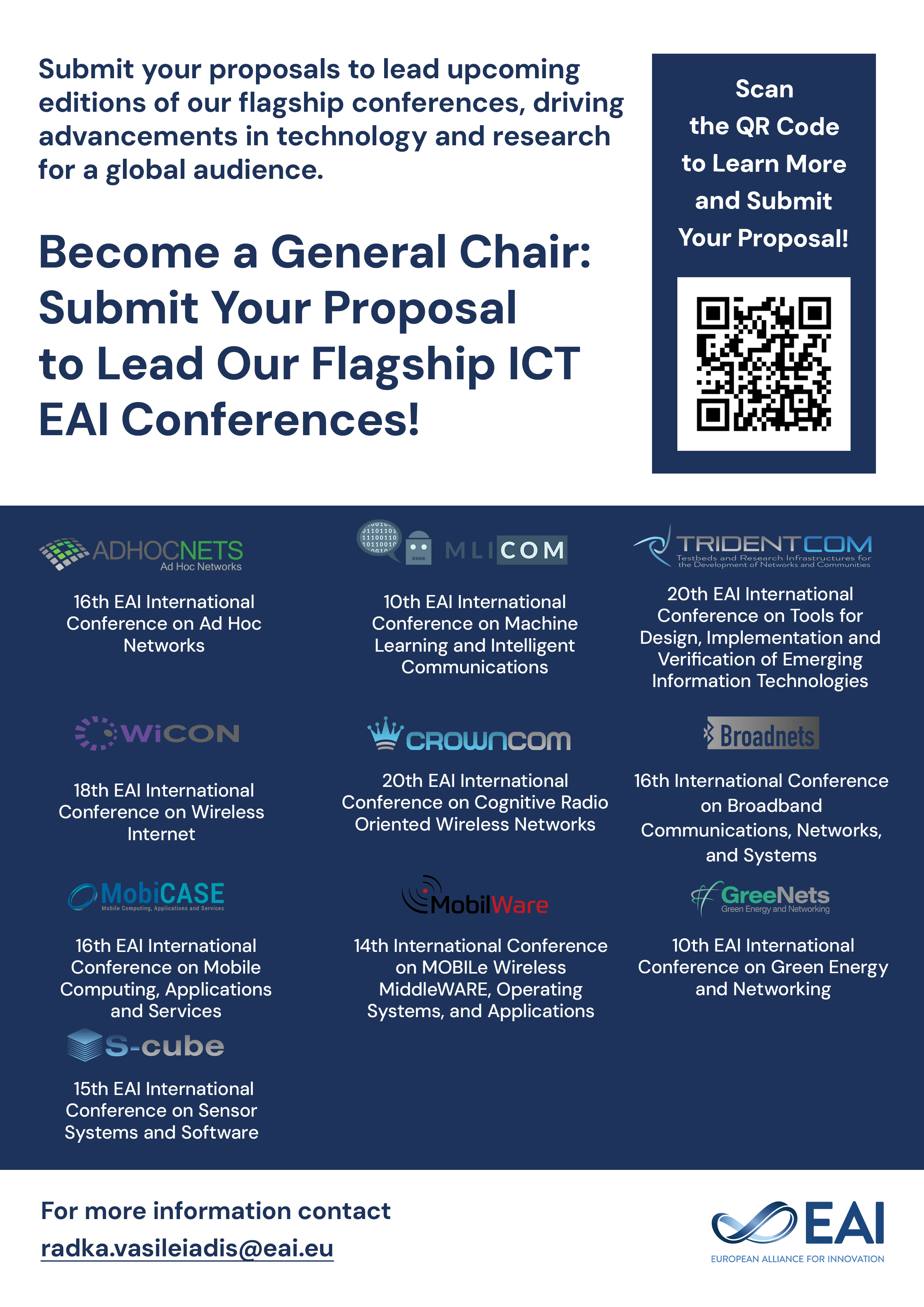
Research Article
Energy Trading Game for Microgrids Using Reinforcement Learning
@INPROCEEDINGS{10.1007/978-3-319-67540-4_12, author={Xingyu Xiao and Canhuang Dai and Yanda Li and Changhua Zhou and Liang Xiao}, title={Energy Trading Game for Microgrids Using Reinforcement Learning}, proceedings={Game Theory for Networks. 7th International EAI Conference, GameNets 2017 Knoxville, TN, USA, May 9, 2017, Proceedings}, proceedings_a={GAMENETS}, year={2017}, month={9}, keywords={Energy trading Game theory Reinforcement learning Smart grids}, doi={10.1007/978-3-319-67540-4_12} }
- Xingyu Xiao
Canhuang Dai
Yanda Li
Changhua Zhou
Liang Xiao
Year: 2017
Energy Trading Game for Microgrids Using Reinforcement Learning
GAMENETS
Springer
DOI: 10.1007/978-3-319-67540-4_12
Abstract
Due to the intermittent production of renewable energy and the time-varying power demand, microgrids (MGs) can exchange energy with each other to enhance their operational performance and reduce their dependence on power plants. In this paper, we investigate the energy trading game in smart grids, in which each MG chooses its energy trading strategy with its connected MGs and power plants according to the energy generation model, the current battery level, the energy demand, and the energy trading history. The Nash equilibria of this game are provided, revealing the conditions under which the MGs can satisfy their energy demands by using local renewable energy generations. In a dynamic version of the game, a Q-learning based strategy is proposed for an MG to obtain the optimal energy trading strategy with other MGs and the energy plants without being aware of the future energy consumption model and the renewable generation of other MGs in the trading market. We apply the estimated renewable energy generation model of the MG and design a hotbooting technique to exploit the energy trading experiences in similar scenarios to initialize the quality values in the learning process to accelerate the convergence speed. The proposed hotbooting Q-learning based energy trading scheme significantly reduces the total energy that the MGs in the smart grid purchase from the power plant and improves the utility of the MG.