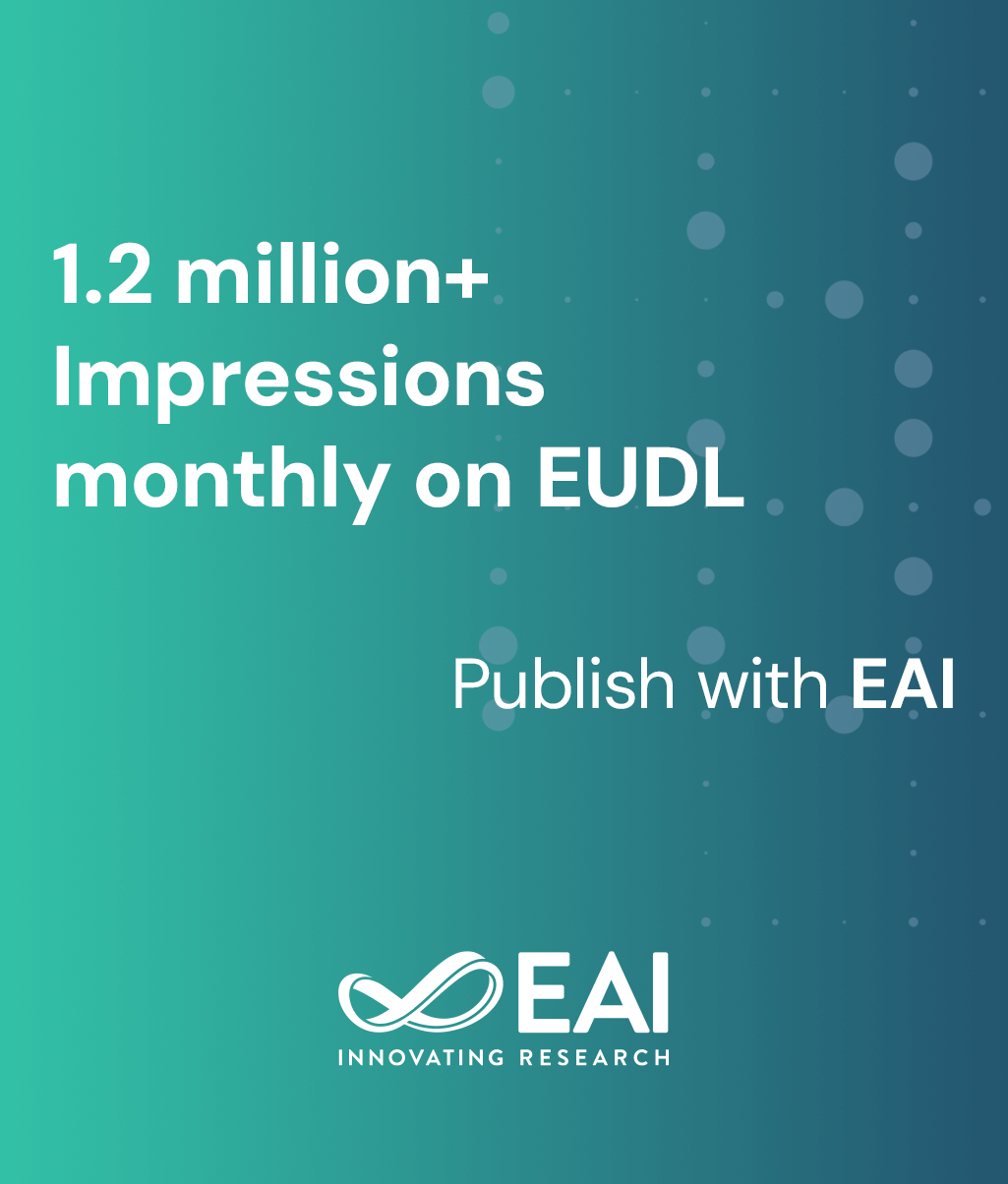
Research Article
Distributed Compressive Sensing for Correlated Information Sources
224 downloads
@INPROCEEDINGS{10.1007/978-3-319-58967-1_15, author={Jeonghun Park and Seunggye Hwang and Janghoon Yang and Kitae Bae and Hoon Ko and Dong Kim}, title={Distributed Compressive Sensing for Correlated Information Sources}, proceedings={Big Data Technologies and Applications. 7th International Conference, BDTA 2016, Seoul, South Korea, November 17--18, 2016, Proceedings}, proceedings_a={BDTA}, year={2017}, month={6}, keywords={Compressive sensing Distributed source coding Sparsity Random projection Sensor networks}, doi={10.1007/978-3-319-58967-1_15} }
- Jeonghun Park
Seunggye Hwang
Janghoon Yang
Kitae Bae
Hoon Ko
Dong Kim
Year: 2017
Distributed Compressive Sensing for Correlated Information Sources
BDTA
Springer
DOI: 10.1007/978-3-319-58967-1_15
Abstract
The abstract should summarize the contents of the paper and should Distributed Compressive Sensing (DCS) improves the signal recovery performance of multi signal ensembles by exploiting both intra- and inter-signal correlation and sparsity structure. In this paper, we propose a novel algorithm, which improves detection performance even without a priori-knowledge on the correlation structure for arbitrarily correlated sparse signal. Numerical results verify that the propose algorithm reduces the required number of measurements for correlated sparse signal detection compared to the existing DCS algorithm.
Copyright © 2016–2025 ICST