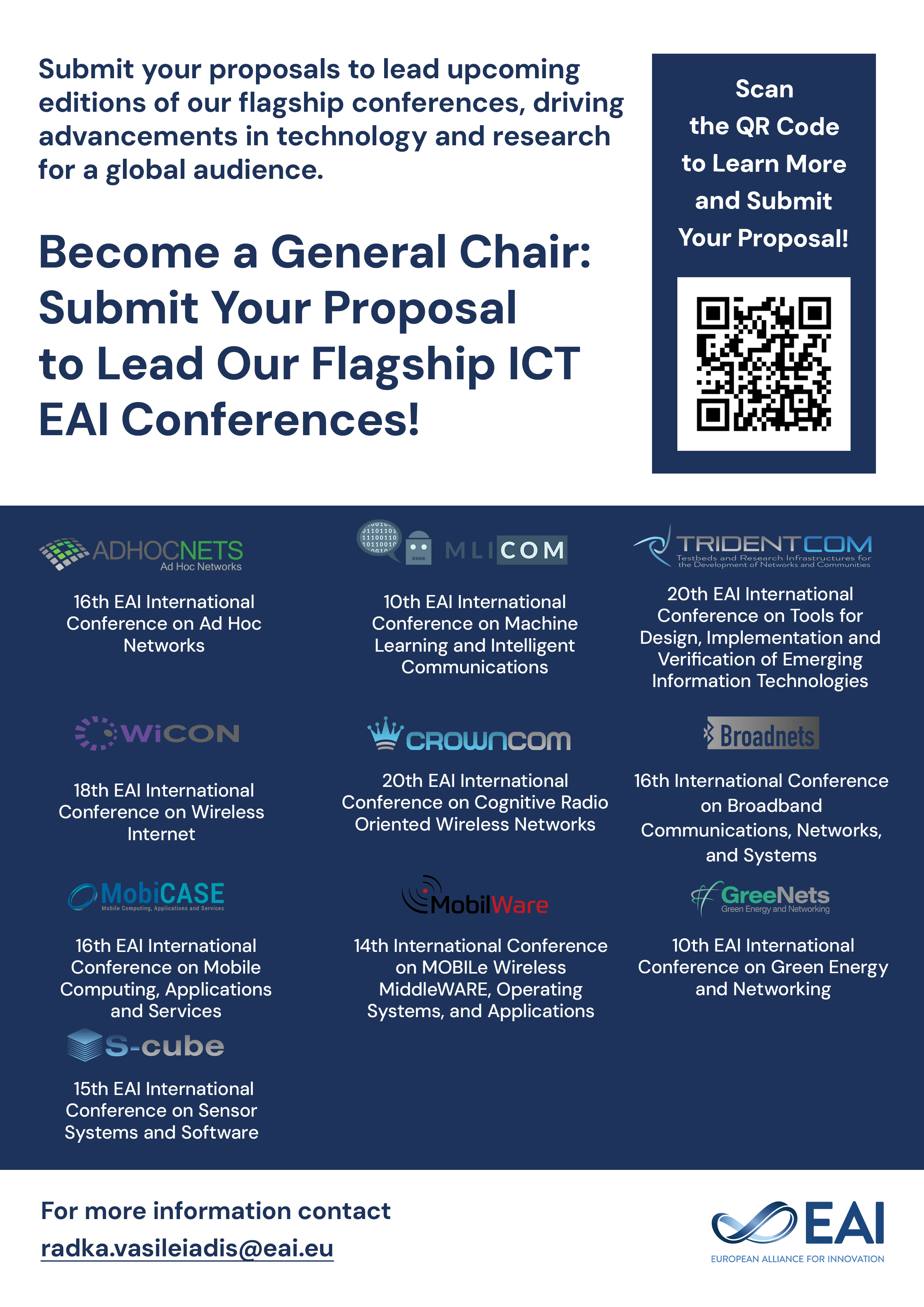
Research Article
Improving the Probability of Clinical Diagnosis of Coronary-Artery Disease Using Extended Kalman Filters with Radial Basis Function Network
@INPROCEEDINGS{10.1007/978-3-319-58877-3_35, author={Mashail Alsalamah and Saad Amin}, title={Improving the Probability of Clinical Diagnosis of Coronary-Artery Disease Using Extended Kalman Filters with Radial Basis Function Network}, proceedings={Wireless Mobile Communication and Healthcare. 6th International Conference, MobiHealth 2016, Milan, Italy, November 14-16, 2016, Proceedings}, proceedings_a={MOBIHEALTH}, year={2017}, month={6}, keywords={Coronary artery disease Extended kalman filter Radial basis function Quasi-Newton and Scaled Conjugate Gradient}, doi={10.1007/978-3-319-58877-3_35} }
- Mashail Alsalamah
Saad Amin
Year: 2017
Improving the Probability of Clinical Diagnosis of Coronary-Artery Disease Using Extended Kalman Filters with Radial Basis Function Network
MOBIHEALTH
Springer
DOI: 10.1007/978-3-319-58877-3_35
Abstract
Kalman filters have been popular in applications to predict time-series data analysis and prediction. This paper uses a form of Extended Kalman Filter to predict the occurrence of CAD (Coronary Artery Disease) using patients data based on different relevant parameters. The work takes a novel approach by using different neural networks training algorithms Quasi-Newton and SCG with combination of activation functions to predict the existence/non-existence of CAD in a patient based on patient’s data set. The prediction probability of this combination is resulted in accuracy of about 92% or above, using cross validation and thresholding to remove the limitation of time-series prediction introduced because of the Extended Kalman filter behavior.