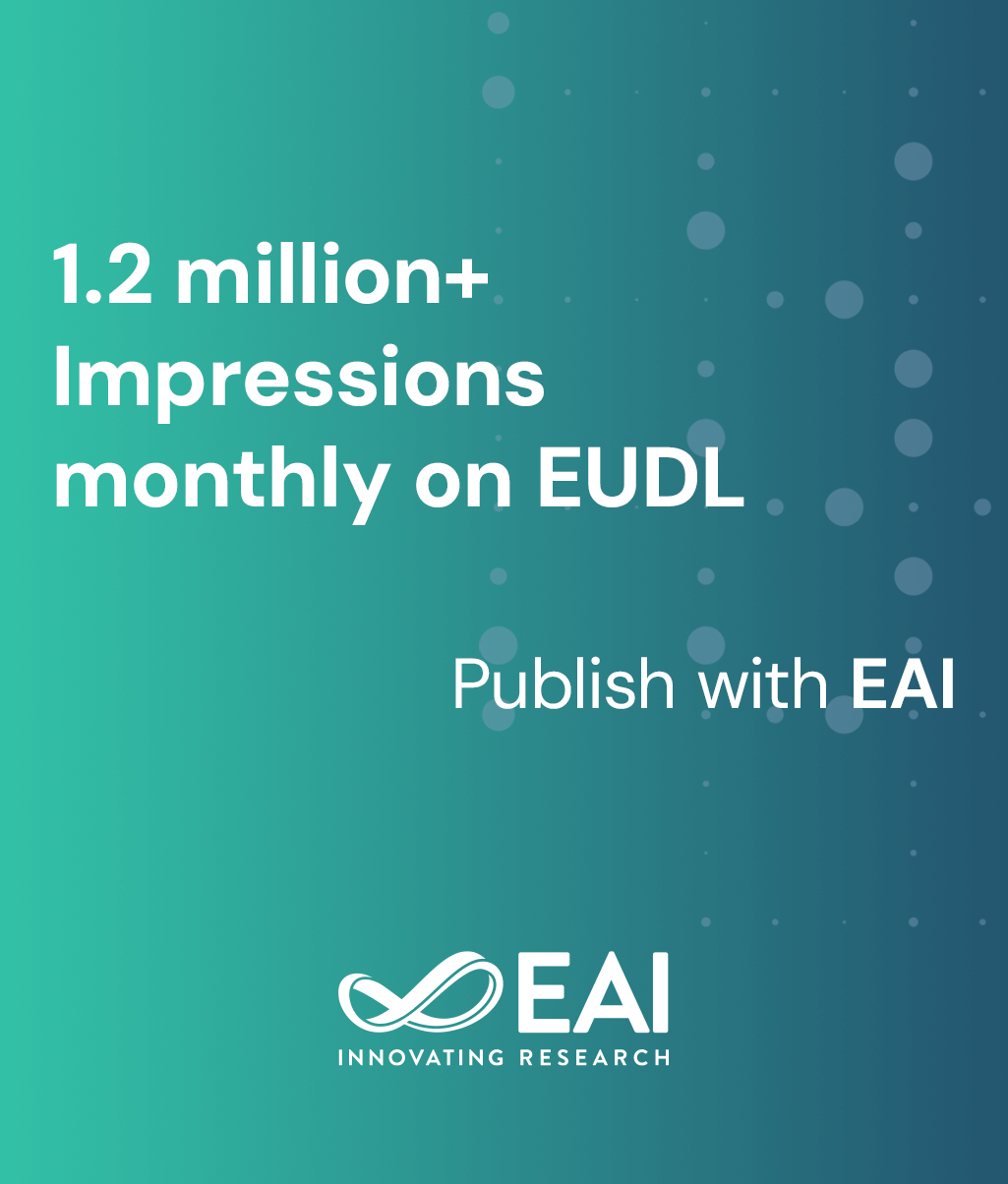
Research Article
Multilayer Radial Basis Function Kernel Machine
@INPROCEEDINGS{10.1007/978-3-319-58877-3_34, author={Mashail Alsalamah and Saad Amin}, title={Multilayer Radial Basis Function Kernel Machine}, proceedings={Wireless Mobile Communication and Healthcare. 6th International Conference, MobiHealth 2016, Milan, Italy, November 14-16, 2016, Proceedings}, proceedings_a={MOBIHEALTH}, year={2017}, month={6}, keywords={Machine learning Classification Unsupervised regression Supervised regression Principal component analysis}, doi={10.1007/978-3-319-58877-3_34} }
- Mashail Alsalamah
Saad Amin
Year: 2017
Multilayer Radial Basis Function Kernel Machine
MOBIHEALTH
Springer
DOI: 10.1007/978-3-319-58877-3_34
Abstract
Radial Basis Function (RBF) Kernel Machines have become commonly used in Machine Learning tasks, but they contain certain flaws (e.g., some suffer from fast growth in the number of learning parameters while predicting data with large number of variations). Besides, Kernel Machines with single hidden layers lack mechanisms for feature selection in multidimensional data space, and machine learning tasks become intractable. This paper investigates “deep learning” architecture composed of multilayered adaptive non-linear components – Multilayer RBF Kernel Machine – to address RBF limitations. Three different approaches of features selection and dimensionality reduction to train RBF based on Multilayer Kernel Learning are explored, and comparisons made between them in terms of accuracy, performance and computational complexity. Results show that the multilayered system produces better results than single-layer architecture. In particular, developing decision support system in term of data mining.