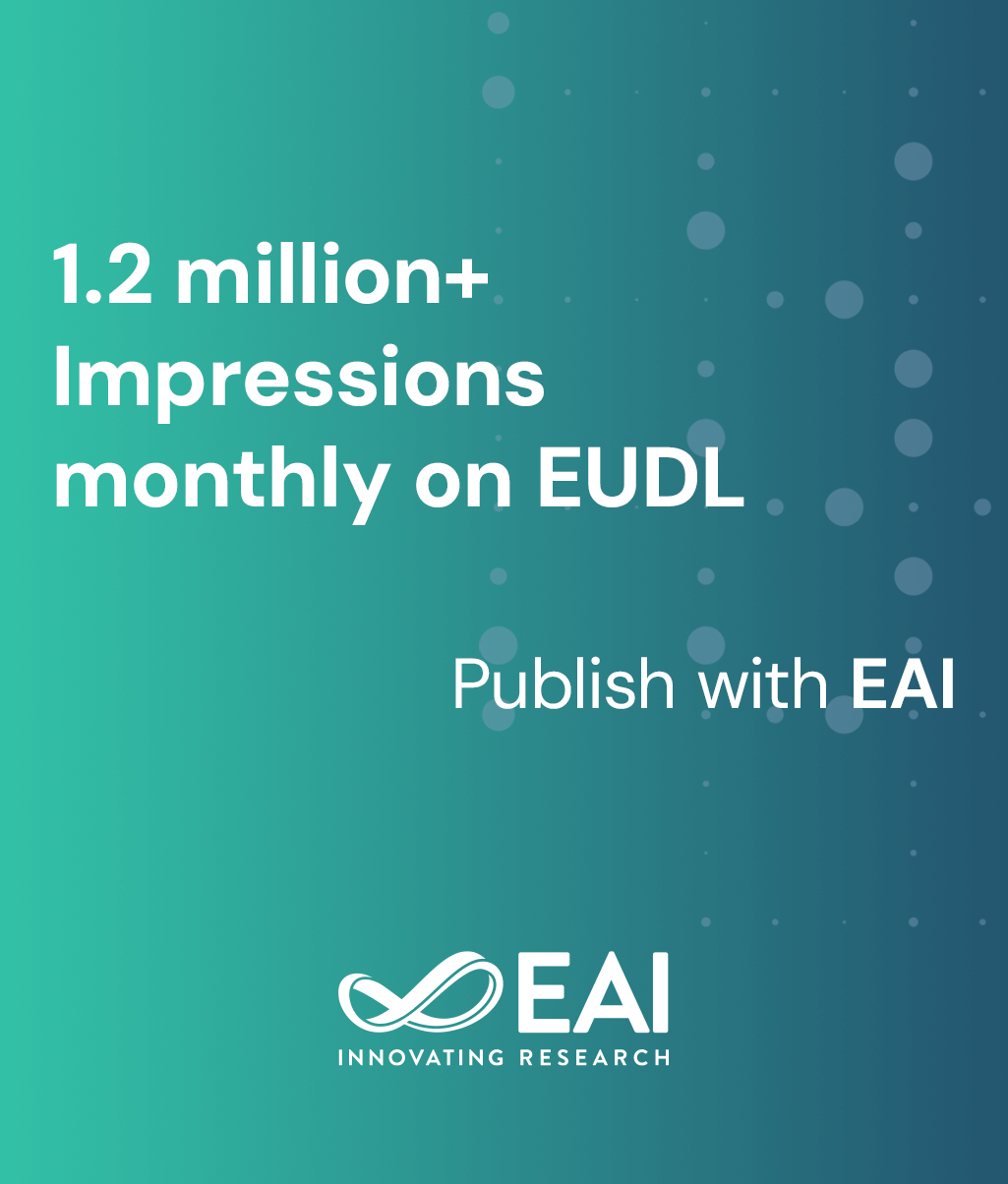
Research Article
Using Smartwatch Sensors to Support the Acquisition of Sleep Quality Data for Supervised Machine Learning
@INPROCEEDINGS{10.1007/978-3-319-58877-3_33, author={Cinzia Bernardeschi and Mario Cimino and Andrea Domenici and Gigliola Vaglini}, title={Using Smartwatch Sensors to Support the Acquisition of Sleep Quality Data for Supervised Machine Learning}, proceedings={Wireless Mobile Communication and Healthcare. 6th International Conference, MobiHealth 2016, Milan, Italy, November 14-16, 2016, Proceedings}, proceedings_a={MOBIHEALTH}, year={2017}, month={6}, keywords={Sleep monitoring Smartwatch Sleep quality estimation}, doi={10.1007/978-3-319-58877-3_33} }
- Cinzia Bernardeschi
Mario Cimino
Andrea Domenici
Gigliola Vaglini
Year: 2017
Using Smartwatch Sensors to Support the Acquisition of Sleep Quality Data for Supervised Machine Learning
MOBIHEALTH
Springer
DOI: 10.1007/978-3-319-58877-3_33
Abstract
It is a common practice in supervised learning techniques to use human judgment to label training data. For this process, data reliability is fundamental. Research on sleep quality found that human sleep stage misperception may occur. In this paper we propose that human judgment be supported by software-driven evaluation based on physiological parameters, selecting as training data only data sets for which human judgment and software evaluation are aligned. A prototype system to provide a broad-spectrum perception of sleep quality data comparable with human judgment is presented. The system requires users to wear a smartwatch recording heartbeat rate and wrist acceleration. It estimates an overall percentage of the sleep stages, to achieve an effective approximation of conventional sleep measures, and to provide a three-class sleep quality evaluation. The training data are composed of the heartbeat rate, the wrist acceleration and the three-class sleep quality. As a proof of concept, we experimented the approach on three subjects, each one over 20 nights.