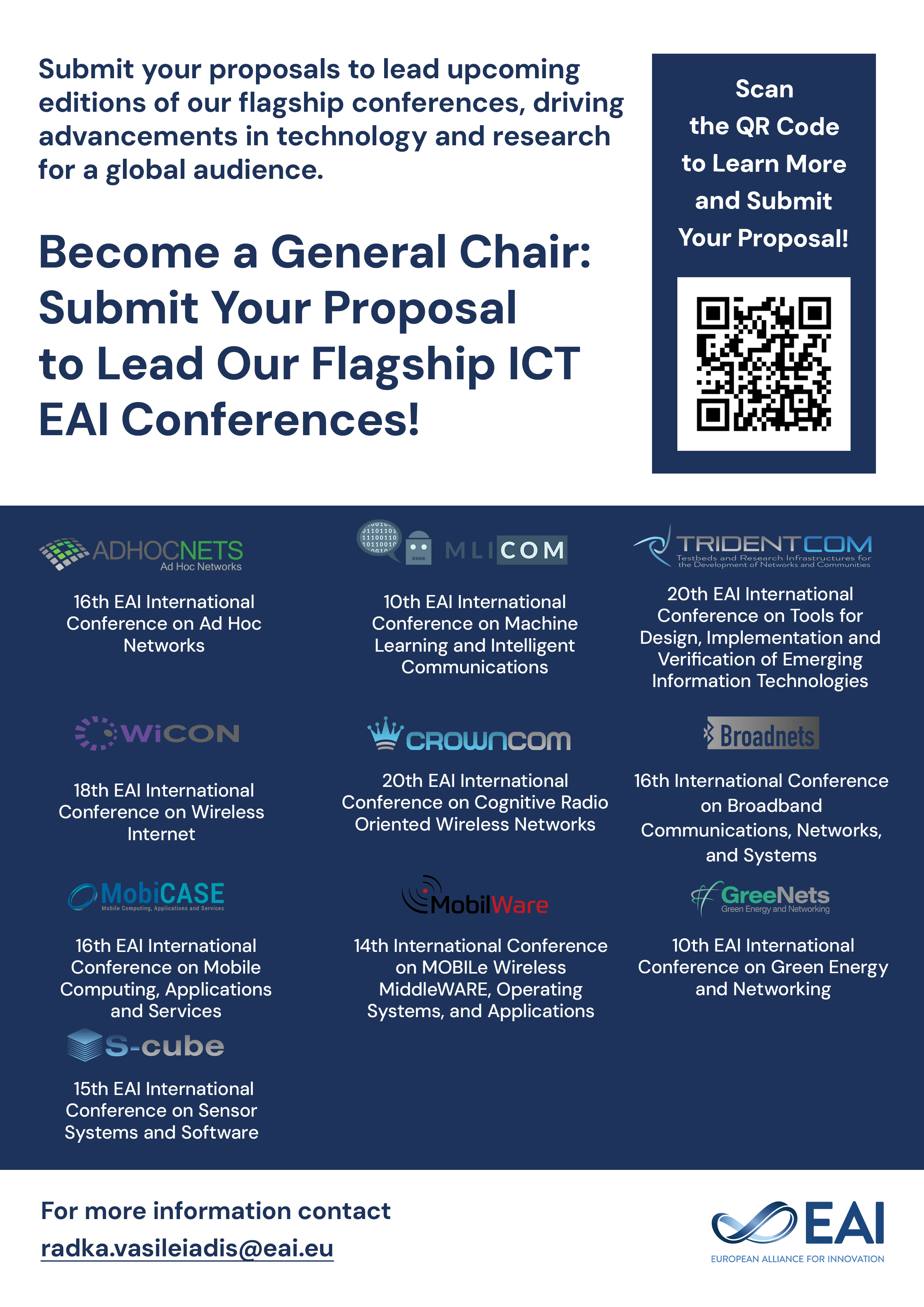
Research Article
A Neural Network Model Based on Co-occurrence Matrix for Fall Prediction
335 downloads
@INPROCEEDINGS{10.1007/978-3-319-58877-3_32, author={Masoud Hemmatpour and Renato Ferrero and Bartolomeo Montrucchio and Maurizio Rebaudengo}, title={A Neural Network Model Based on Co-occurrence Matrix for Fall Prediction}, proceedings={Wireless Mobile Communication and Healthcare. 6th International Conference, MobiHealth 2016, Milan, Italy, November 14-16, 2016, Proceedings}, proceedings_a={MOBIHEALTH}, year={2017}, month={6}, keywords={}, doi={10.1007/978-3-319-58877-3_32} }
- Masoud Hemmatpour
Renato Ferrero
Bartolomeo Montrucchio
Maurizio Rebaudengo
Year: 2017
A Neural Network Model Based on Co-occurrence Matrix for Fall Prediction
MOBIHEALTH
Springer
DOI: 10.1007/978-3-319-58877-3_32
Abstract
Fall avoidance systems reduce injuries due to unintentional falls, but most of them are fall detections that activate an alarm after the fall occurrence. Since predicting a fall is the most promising approach to avoid a fall injury, this study proposes a method based on new features and multilayer perception that outperforms state-of-the-art approaches. Since accelerometer and gyroscope embedded in a smartphone are recognized to be precise enough to be used in fall avoidance systems, they have been exploited in an experimental analysis in order to compare the proposal with state-of-the-art approaches. The results have shown that the proposed approach improves the accuracy from 83% to 90%.
Copyright © 2016–2024 ICST