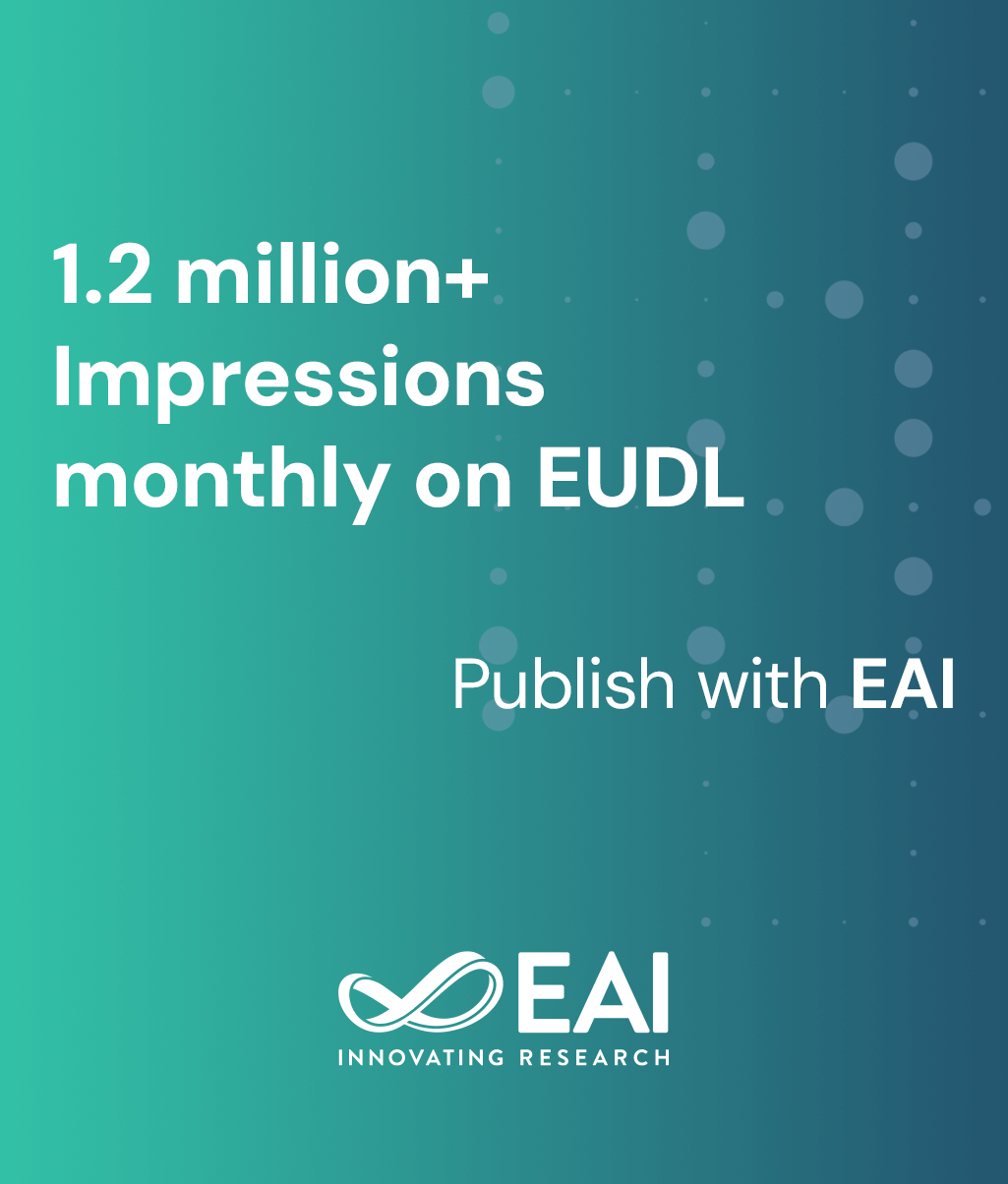
Research Article
Analysing Emotional Sentiment in People’s YouTube Channel Comments
@INPROCEEDINGS{10.1007/978-3-319-55834-9_21, author={Eleanor Mulholland and Paul Mc Kevitt and Tom Lunney and Karl-Michael Schneider}, title={Analysing Emotional Sentiment in People’s YouTube Channel Comments}, proceedings={Interactivity, Game Creation, Design, Learning, and Innovation. 5th International Conference, ArtsIT 2016, and First International Conference, DLI 2016, Esbjerg, Denmark, May 2--3, 2016, Proceedings}, proceedings_a={ARTSIT \& DLI}, year={2017}, month={3}, keywords={360-MAM-Affect 360-MAM-Select Affective computing Brit Lab EmoSenticNet Gamification Google YouTube API Head Squeeze Machine learning Natural language processing Recommender system Sentiment analysis YouTube YouTube EDU}, doi={10.1007/978-3-319-55834-9_21} }
- Eleanor Mulholland
Paul Mc Kevitt
Tom Lunney
Karl-Michael Schneider
Year: 2017
Analysing Emotional Sentiment in People’s YouTube Channel Comments
ARTSIT & DLI
Springer
DOI: 10.1007/978-3-319-55834-9_21
Abstract
Online recommender systems are useful for media asset management where they select the best content from a set of media assets. We are developing a recommender system called 360-MAM-Select for educational video content. 360-MAM-Select utilises sentiment analysis, emotion modeling and gamification techniques applied to people’s comments on videos, for the recommendation of media assets. Here, we discuss the architecture of 360-MAM-Select, including its sentiment analysis module, 360-MAM-Affect and gamification module, 360-Gamify. 360-MAM-Affect is implemented with the YouTube API [9], GATE [5] for natural language processing, EmoSenticNet [8] for identifying emotion words and RapidMiner [20] to count the average frequency of emotion words identified. 360-MAM-Affect is tested by tagging comments on the YouTube channels, Brit Lab/Head Squeeze [3], YouTube EDU [28], Sam Pepper [22] and MyTop100Videos [18] with EmoSenticNet [8] in order to identify emotional sentiment. Our results show that and are the most frequent emotions across all the YouTube channel comments. Future work includes further implementation and testing of 360-MAM-Select deploying the Unifying Framework [25] and Emotion-Imbued Choice (EIC) model [13] within 360-MAM-Affect for emotion modelling, by collecting emotion feedback and sentiment from users when they interact with media content. Future work also includes implementation of the gamification module, 360-Gamify, in order to check its suitability for improving user participation with the Octalysis gamification framework [4].