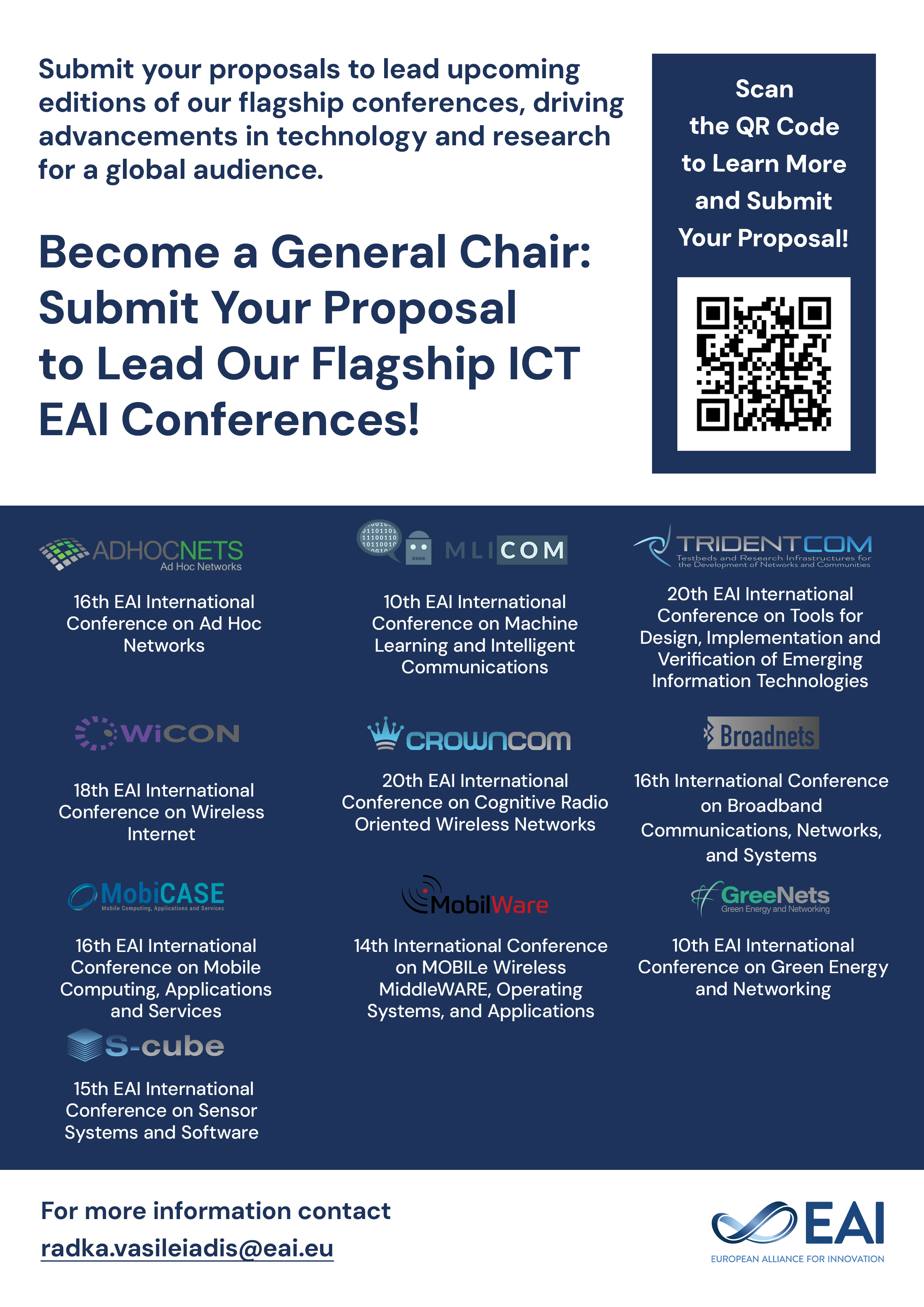
Research Article
Optimization of Voiced Excitation Model by MVF Algorithm
@INPROCEEDINGS{10.1007/978-3-319-52730-7_6, author={Bing Xue and Xiaoqun Zhao}, title={Optimization of Voiced Excitation Model by MVF Algorithm}, proceedings={Machine Learning and Intelligent Communications. First International Conference, MLICOM 2016, Shanghai, China, August 27-28, 2016, Revised Selected Papers}, proceedings_a={MLICOM}, year={2017}, month={2}, keywords={MVF Residual signal Pitch Voicing excitation model TBE}, doi={10.1007/978-3-319-52730-7_6} }
- Bing Xue
Xiaoqun Zhao
Year: 2017
Optimization of Voiced Excitation Model by MVF Algorithm
MLICOM
Springer
DOI: 10.1007/978-3-319-52730-7_6
Abstract
In mixed excitation linear prediction (MELP) vocoder incentive model, the decision error of traditional maximum voicing frequency (MVF) algorithm is relatively large. In this paper, it proposes a method to optimize MVF algorithm. Firstly, in many MVF algorithms, considering about algorithm accuracy, applicability and real-time, It selects the most appropriate algorithm (cumulative harmonic scoring algorithm) for very low rate speech coder. And then, the method optimize the definition and noise immunity of the algorithm, which use adaptive MVF value to divided spectrum into two sub-bands. Finally, using pitch as performance parameters of incentive model, it simulates voiced incentive model, and compares the pitch error rate of two band excitation (TBE) model and MELP model. A conclusion is generated that TBE model is closer to the original speech features, and its performance is better than that of the original MELP incentive model.