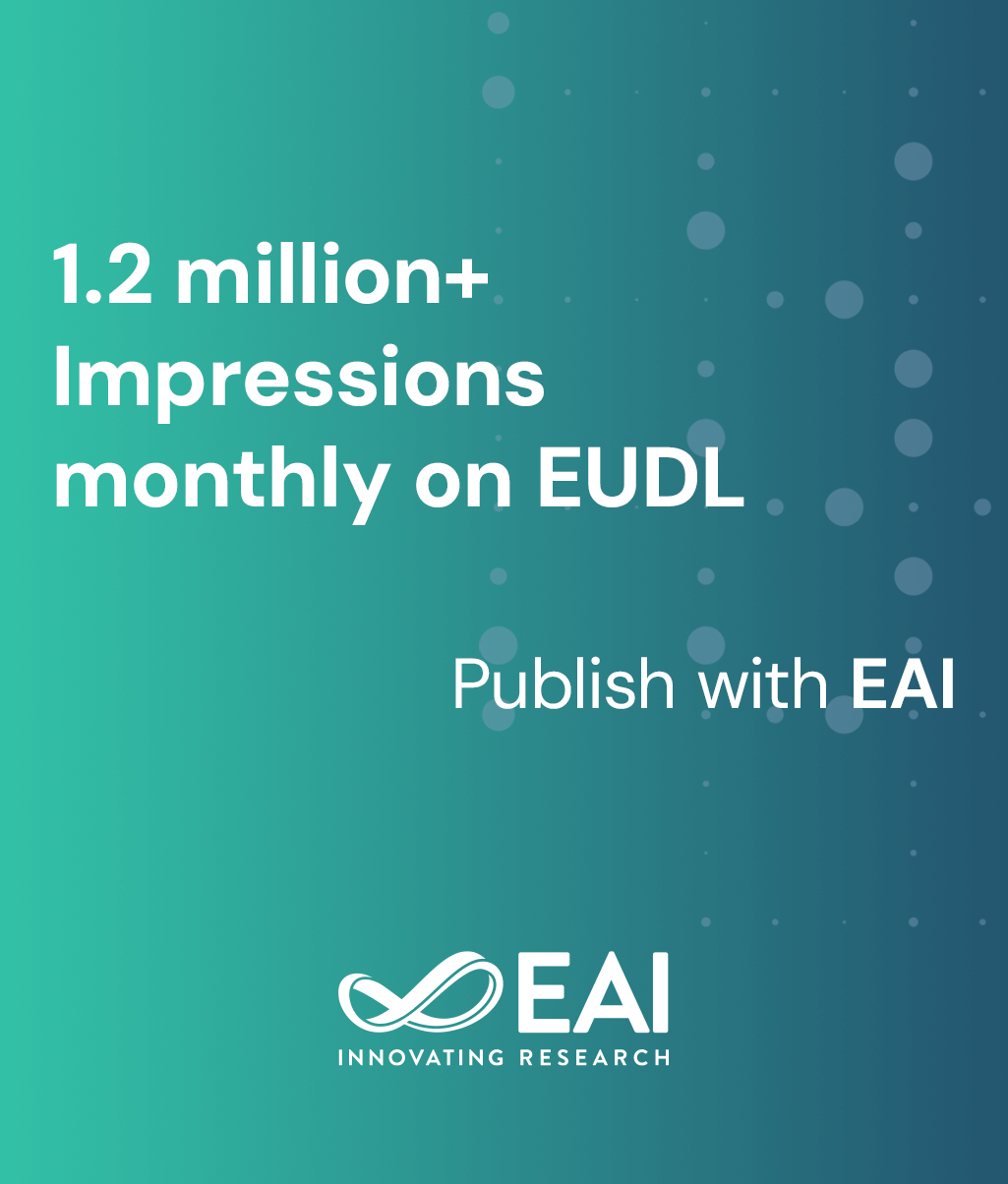
Research Article
Robust Spectral-Temporal Two-Dimensional Spectrum Prediction
@INPROCEEDINGS{10.1007/978-3-319-52730-7_40, author={Guoru Ding and Siyu Zhai and Xiaoming Chen and Yuming Zhang and Chao Liu}, title={Robust Spectral-Temporal Two-Dimensional Spectrum Prediction}, proceedings={Machine Learning and Intelligent Communications. First International Conference, MLICOM 2016, Shanghai, China, August 27-28, 2016, Revised Selected Papers}, proceedings_a={MLICOM}, year={2017}, month={2}, keywords={Spectrum prediction Anomaly data Missing data Matrix completion and recovery}, doi={10.1007/978-3-319-52730-7_40} }
- Guoru Ding
Siyu Zhai
Xiaoming Chen
Yuming Zhang
Chao Liu
Year: 2017
Robust Spectral-Temporal Two-Dimensional Spectrum Prediction
MLICOM
Springer
DOI: 10.1007/978-3-319-52730-7_40
Abstract
With the development of mobile network, the limited spectrum resources are being running out of. Therefore, there is a harsh need for us to be able to know the current spectrum state as well as predict the future spectrum state. Though a number of studies are about spectrum prediction, some fundamental issues still remain unresolved: (i) the existing studies do not account for anomaly data, which causes serious performance degradation, (ii) they do not account for missing data, which may not hold in reality. To address these issues, in this paper, we develop a robust spectral-temporal spectrum prediction (R-STSP) framework from corrupted and incomplete observations. Firstly, we present data analytic of real-world spectrum measurements to analyze the impact of anomalies on the rank distribution of spectrum matrices. Then, from a spectral-temporal spectrum perspective, we formulate the R-STSP as a matrix recovery problem and develop an optimization method to efficiently solve it. We apply the formulated R-STSP to real-world VHF spectrum data and the results show that R-STSP outperforms state-of-the-art schemes.