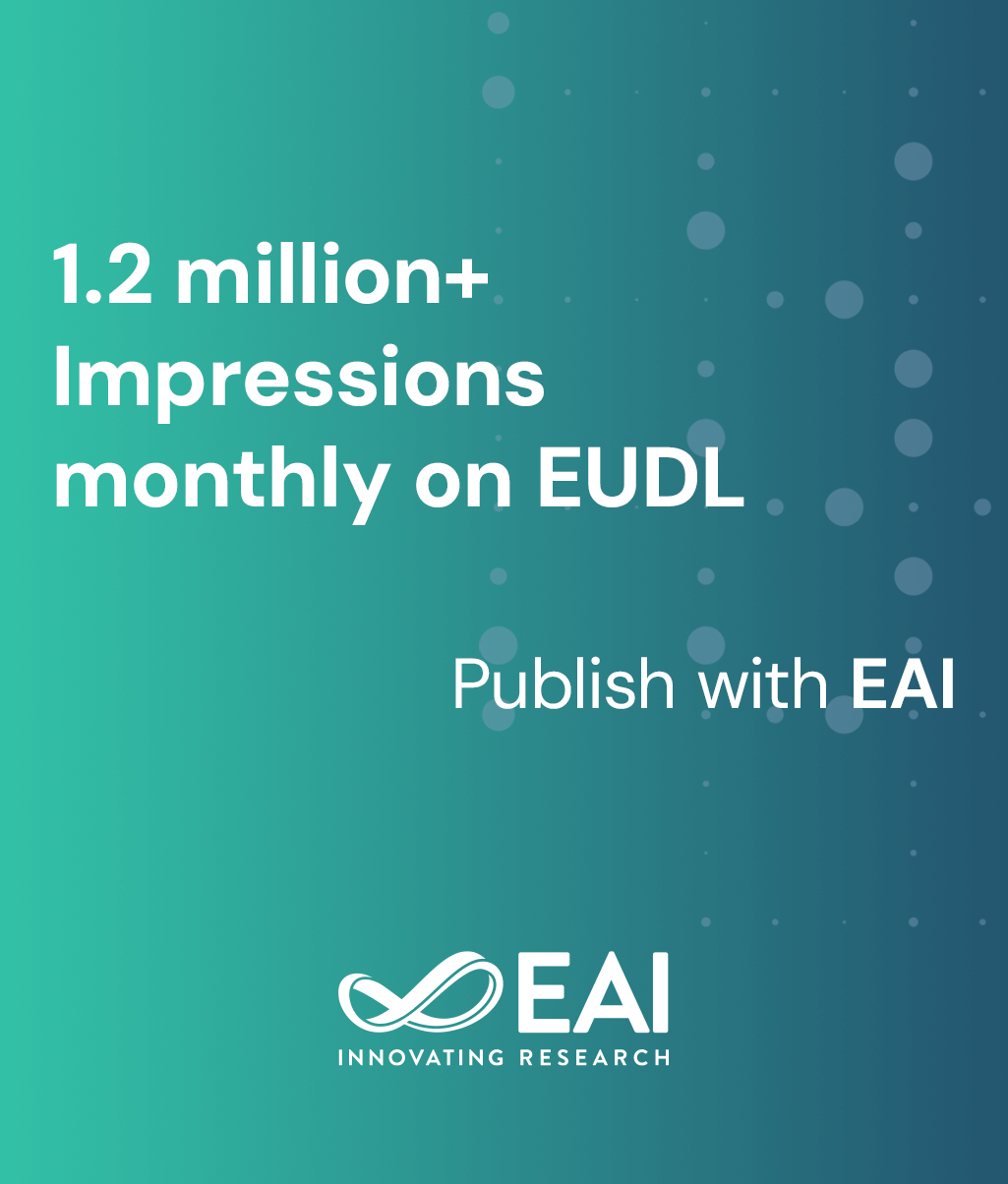
Research Article
SLNR-Oriented Power Control in Cognitive Radio Networks with Channel Uncertainty
@INPROCEEDINGS{10.1007/978-3-319-52730-7_37, author={Le Wang and Guoru Ding and Guochun Ren and Jin Chen and Zhen Xue and Haichao Wang and Yumeng Wang}, title={SLNR-Oriented Power Control in Cognitive Radio Networks with Channel Uncertainty}, proceedings={Machine Learning and Intelligent Communications. First International Conference, MLICOM 2016, Shanghai, China, August 27-28, 2016, Revised Selected Papers}, proceedings_a={MLICOM}, year={2017}, month={2}, keywords={Cognitive radio network Power control Channel uncertainty Massive secondary connections Signal-to-leakage-and-noise ratio}, doi={10.1007/978-3-319-52730-7_37} }
- Le Wang
Guoru Ding
Guochun Ren
Jin Chen
Zhen Xue
Haichao Wang
Yumeng Wang
Year: 2017
SLNR-Oriented Power Control in Cognitive Radio Networks with Channel Uncertainty
MLICOM
Springer
DOI: 10.1007/978-3-319-52730-7_37
Abstract
The majority of existing studies on power control in cognitive radio networks focus on maximization of signal-to-interference-noise ratio (SINR), while this paper firstly introduces the (SLNR)-oriented power control to optimize throughput in a cognitive radio network (CRN), where massive secondary connections (SCs) and a primary user (PU) coexist with each other sharing the same frequency spectrum. Considering the practical challenge that the channel gains between SCs and PU are typically uncertain, we introduce a probabilistic interference constraint to protect the PU’s transmission and reformulate it according to the ’s central limit theorem (CLT). Then, we apply the convex optimization theory to solve the intractable problem by excluding the probabilistic constraint. Especially, a novel algorithm based on the first-order Lagrangian is developed where the dual variables are updated simultaneously. Furthermore, we provide numerial results using different parameter, which display that the proposed method can achieve higher throughput with much lower computational complexity comparing with the existing literature.